Parametric Data-Driven Reduced Order Model using neural networks and manifold-based interpolation
2023 24TH INTERNATIONAL CONFERENCE ON PROCESS CONTROL, PC(2023)
摘要
The simulation of many industrial processes can be prohibitively expensive, due to the complexity of the mathematical models used to describe them. This makes the control of such processes a challenging task, especially when the models are parametric. In light of this, we present a data-driven framework to build a parametric reduced-order model (ROM) through the combination of singular value decomposition (SVD) and neural networks. A manifold-based nonlinear interpolation is used to deal with the effect of the parameter change on the spatial modes. On the other hand, we train a neural network to resolve the dynamics of the system. The framework presented in this work is non-intrusive, i.e., it does not require access to the governing equations of the original model. The performance of the framework is evaluated on a process of convective drying of wood chip particles. Results show that the ROM developed can accurately predict the dynamics of the process for a new set of parameters.
更多查看译文
关键词
Reduced-order model,proper orthogonal decomposition,Recurrent neural network,manifold-based interpolation
AI 理解论文
溯源树
样例
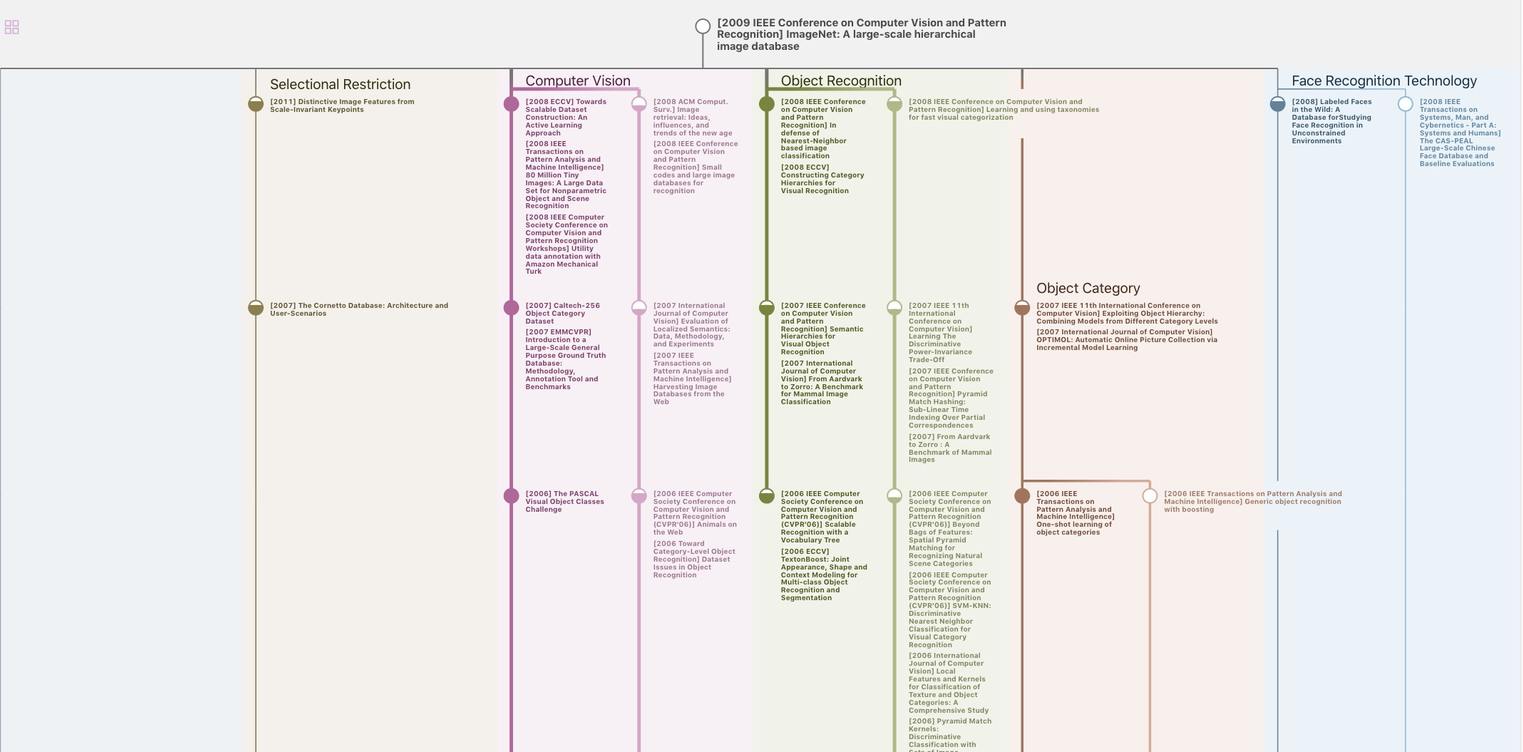
生成溯源树,研究论文发展脉络
Chat Paper
正在生成论文摘要