Deep transfer learning-based approach for detection of cracks on eggs
JOURNAL OF FOOD PROCESS ENGINEERING(2023)
摘要
Eggs are the most nutrient-rich and protein-dense food that is affordable and consumed by most of the population. But cracks on the eggshells can pave the way for microbial contamination of the eggs posing threat to the health of the consumers. Therefore, it is crucial for the egg industries to check for the quality of eggs before reaching consumers to avoid outbreaks. Automatic visual inspection is one of the techniques that has gained popularity in the field of eggshell crack identification because of the advancements in hardware and deep learning. However, due to the limited training data, classifying eggs using eggshell crack images is challenging. Therefore, deep transfer learning was employed in this study using a pre-trained DenseNet121 architecture in three ways-fine-tuning (FT), feature extraction, and training deep features on machine learning classifiers. The results revealed that the FT technique performed best among the three approaches with 98.38% accuracy. Practical Applications Deep transfer learning-based techniques in food quality assessments are seeing a rise in the food industry. Eggshell crack detection is one of the challenges that can be addressed using the transfer learning approach. The purpose of this study was to investigate the effects of different transfer learning approaches in classifying the image patches extracted from egg images and determine the most effective technique to identify cracks on eggs, despite the difficulty of the classification task using a small data set of egg images. These results can promote the application of transfer learning in non-destructive egg quality evaluation in the egg processing industries.
更多查看译文
关键词
crack detection, DenseNet121, feature extraction, fine-tuning, transfer learning
AI 理解论文
溯源树
样例
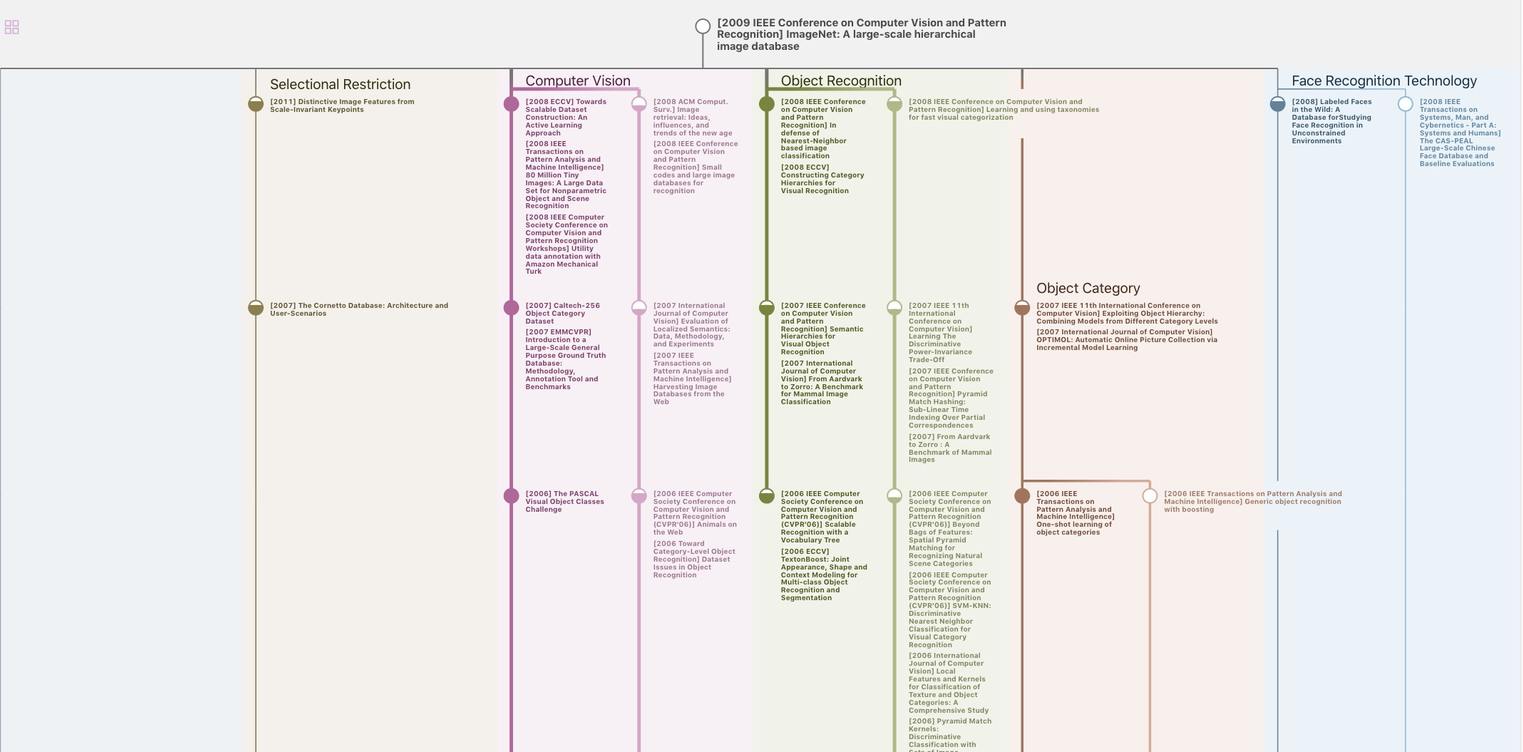
生成溯源树,研究论文发展脉络
Chat Paper
正在生成论文摘要