Multi-scale hybrid three-dimensional-two-dimensional-attention boosted convolutional neural network for hyperspectral image classification
JOURNAL OF APPLIED REMOTE SENSING(2023)
Abstract
Hyperspectral image (HSI) classification is a hot topic in the field of remote sensing applications. However, due to the high-dimensional and extensive spectral and spatial information of HSIs, effective feature extraction is difficult, which leads to a lower accuracy of HSI classification. In this work, a convolutional neural network (CNN) approach based on a multiscale hybrid 3D-2D-attention mechanism (MHAC) is proposed. Linear discriminant analysis dimension reduction is performed for HSIs, and the central pixel and its adjacent pixels are input into the network as a whole. Based on a multi-scale three-dimensional convolutional neural network (3D CNN) and a two-dimensional convolutional neural network (2D CNN), fine spectral and spatial features are automatically extracted, which improves the network classification capability. At the same time, the channel attention mechanism is embedded in the 2D CNN, and the spatial attention mechanism is embedded in the 3D CNN, which can assign different weights to the retrieved features, effectively utilizing the spatial spectral information to optimize features and improve the feature extraction ability. The experimental results demonstrate that the MHAC achieves an accuracy of 98.72%, 97.60%, and 97.90% on the WHU-Hi dataset and 98.93% on the Indian-Pines dataset based on only 100 samples. The MHAC model proposed in this paper has a good feature extraction ability that effectively reduces the impact of high-dimensional data and small sample problems on classification and improves the accuracy of HSIs classification.
MoreTranslated text
Key words
hyperspectral image classification,attention mechanism,multi-scale,convolutional neural network,machine learning
AI Read Science
Must-Reading Tree
Example
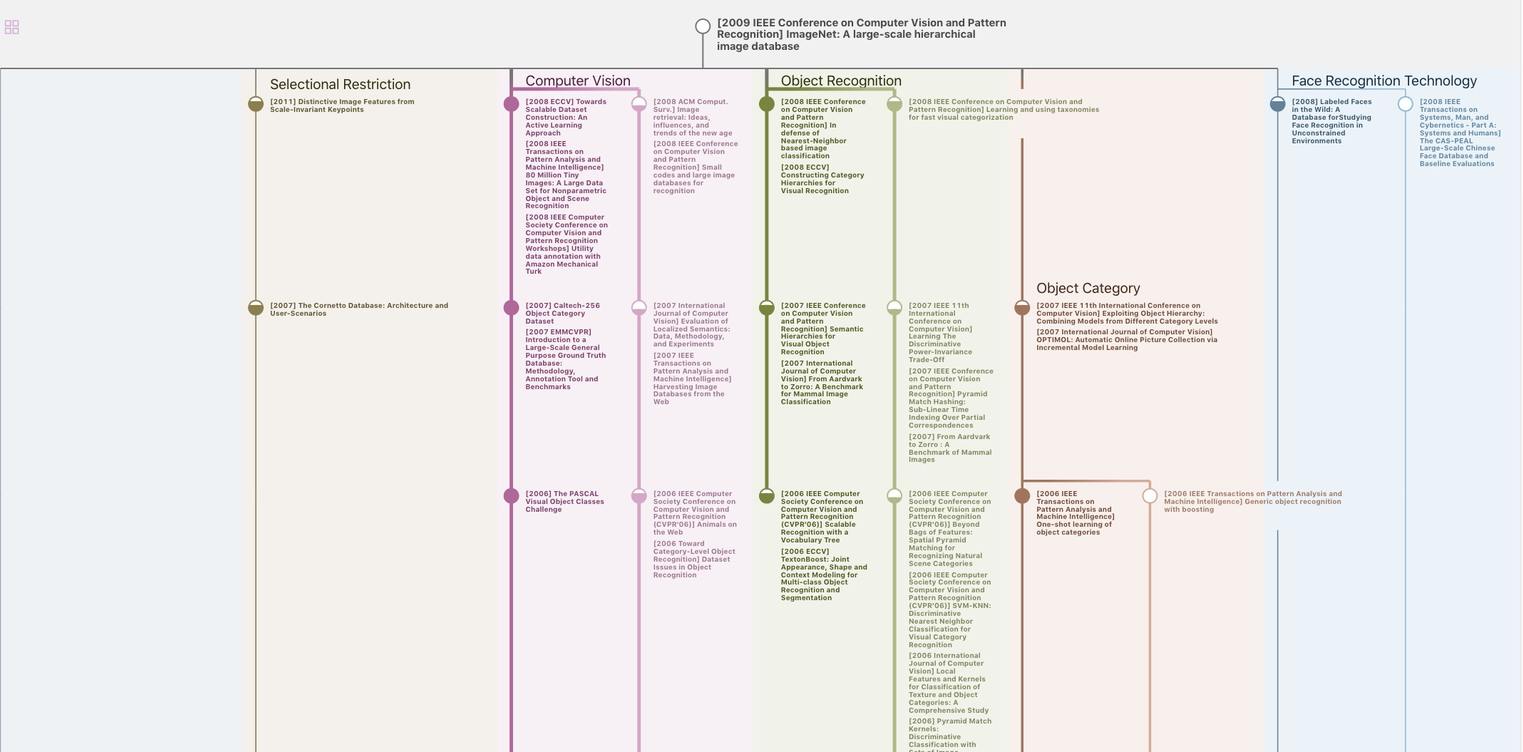
Generate MRT to find the research sequence of this paper
Chat Paper
Summary is being generated by the instructions you defined