A Precise and Adaptive Graph Regularized Low Rank Representation Model for Recognizing Oil-bearing
CONTROL ENGINEERING AND APPLIED INFORMATICS(2023)
摘要
The recognition of oil-bearing formation is an important part in oil exploration, and recognition technology influences the predictive accuracy and efficiency. Low rank representation (LRR) has aroused much attention in the field of data mining. As a modified version, the low rank representation with adaptive graph regularization (LRR-AGR) exploits the global and local information of data for graph learning, and it simultaneously integrates distance regularization term, non-negative constraint and a rank constraint into the framework of LRR. However, how to balance these regularization terms according to the data greatly limits its clustering performance. To adaptively balance these regularization terms according to the data and further improve the clustering performance, we propose a novel model named low-rank representation with adaptive parameters and graph regularization (LRR-APGR) in this paper. Firstly, a novel parameter optimization model is formulated and designed based on the framework of LRR-AGR and the feedback mechanism. Secondly, two global intelligent optimization algorithms, which can effectively solve the parameter optimization problem are presented based on particle swarm optimization (PSO) in multi-dimensional continuous space. Experimental results on the data oilsk81, oilsk83 and oilsk85 wells of Jianghan oil fields in China show that the proposed method can significantly improve the clustering performance and the predictive accuracy.
更多查看译文
关键词
Low rank representation, graph regularization, rank constraint, particle swarm optimization
AI 理解论文
溯源树
样例
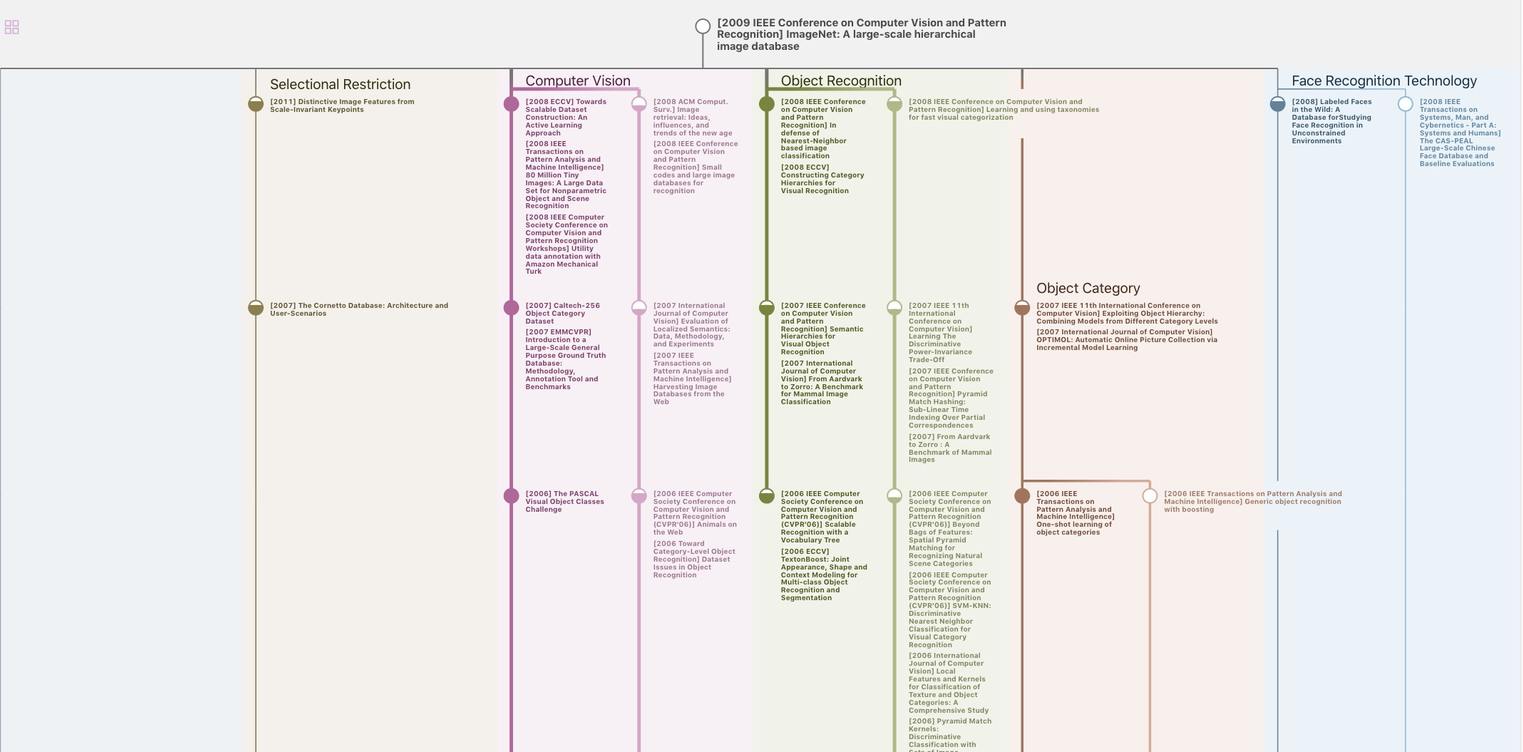
生成溯源树,研究论文发展脉络
Chat Paper
正在生成论文摘要