Saw Blade Wear Identification Based on Data Enhancement and Feature Fusion
IEEE Access(2023)
Abstract
In order to solve the problem of low accuracy of tool wear detection due to the poor quality of generated data under small sample problems, a deep learning model based on data enhancement and feature fusion is proposed. Firstly, in order to solve the problem that there is no quality evaluation standard in the training process of the traditional generative adversarial network (GAN), the K nearest neighbor algorithm is proposed to test the data generated by the GAN model for the second time. The improved GAN model can be automatically trained to get the optimal model according to the second test results. Secondly, in order to enhance the anti-interference effect of the model, a double-path parallel convolutional neural network (DPCNN) which combines with the characteristics of frequency domain and time-frequency domain is constructed to analyze the wear data. Furthermore, the hyperparameters of the model are optimized by Bayesian optimization algorithm (BOA). Finally, the effectiveness of this method is verified in the saw blade wear detection experiment. The results show that the performance of this model is better than other models, and the accuracy rate in the experimental detection reaches 100%.
MoreTranslated text
Key words
Data models, Generative adversarial networks, Convolutional neural networks, Milling, Feature extraction, Monitoring, Training, Predictive control, Aging, Tools, Product safety, Saw blade wear identification, feature fusion, Bayesian optimization algorithm, double-path parallel convolution neural network, generative adversarial networks
AI Read Science
Must-Reading Tree
Example
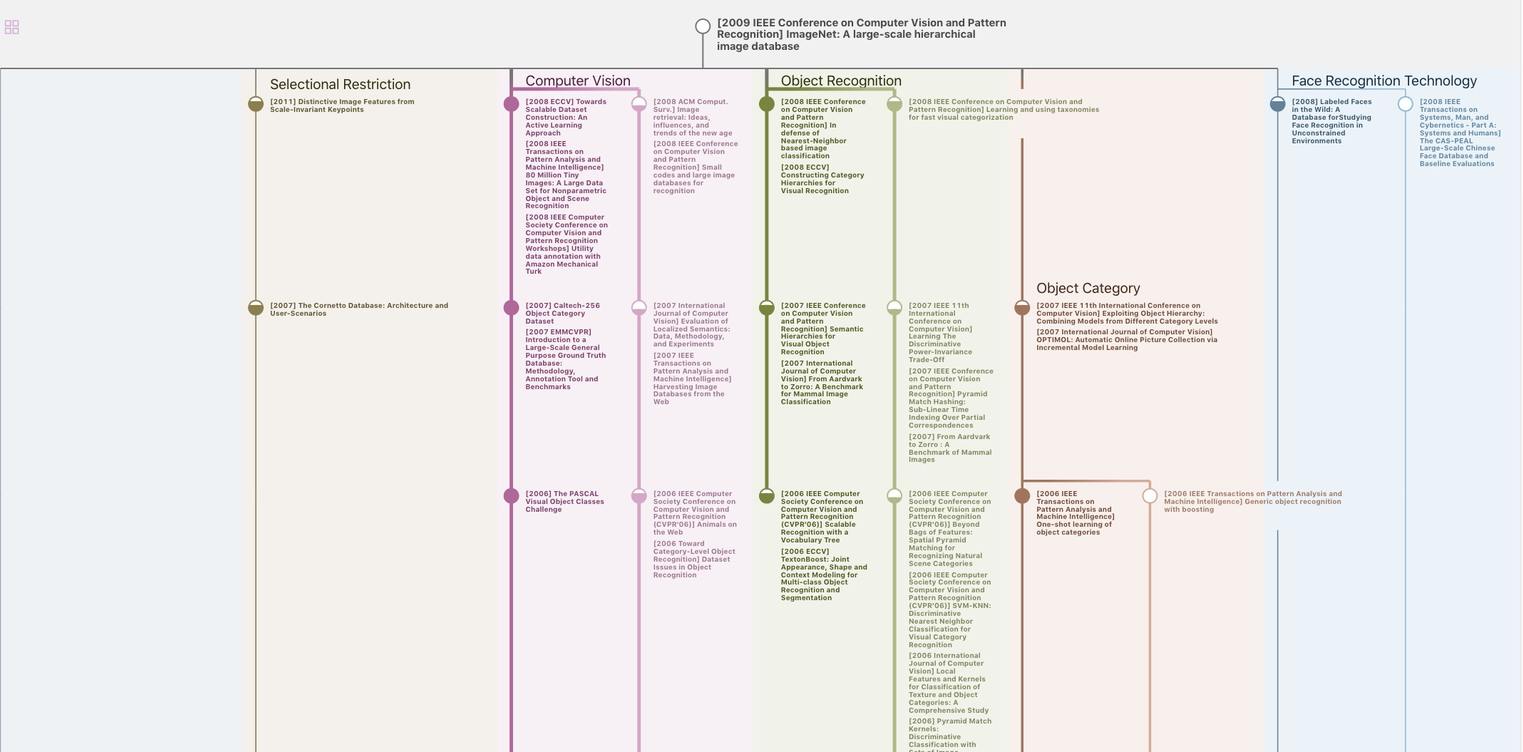
Generate MRT to find the research sequence of this paper
Chat Paper
Summary is being generated by the instructions you defined