High-dimensional Newey-Powell Test Via Approximate Message Passing
arXiv (Cornell University)(2023)
摘要
Homoscedastic regression error is a common assumption in many high-dimensional regression models and theories. Although heteroscedastic error commonly exists in real-world datasets, testing heteroscedasticity remains largely underexplored under high-dimensional settings. We consider the heteroscedasticity test proposed in Newey and Powell (1987), whose asymptotic theory has been well-established for the low-dimensional setting. We show that the Newey-Powell test can be developed for high-dimensional data. For asymptotic theory, we consider the setting where the number of dimensions grows with the sample size at a linear rate. The asymptotic analysis for the test statistic utilizes the Approximate Message Passing (AMP) algorithm, from which we obtain the limiting distribution of the test. The numerical performance of the test is investigated through an extensive simulation study. As real-data applications, we present the analysis based on "international economic growth" data (Belloni et al. 2011), which is found to be homoscedastic, and "supermarket" data (Lan et al., 2016), which is found to be heteroscedastic.
更多查看译文
关键词
approximate message,high-dimensional,newey-powell
AI 理解论文
溯源树
样例
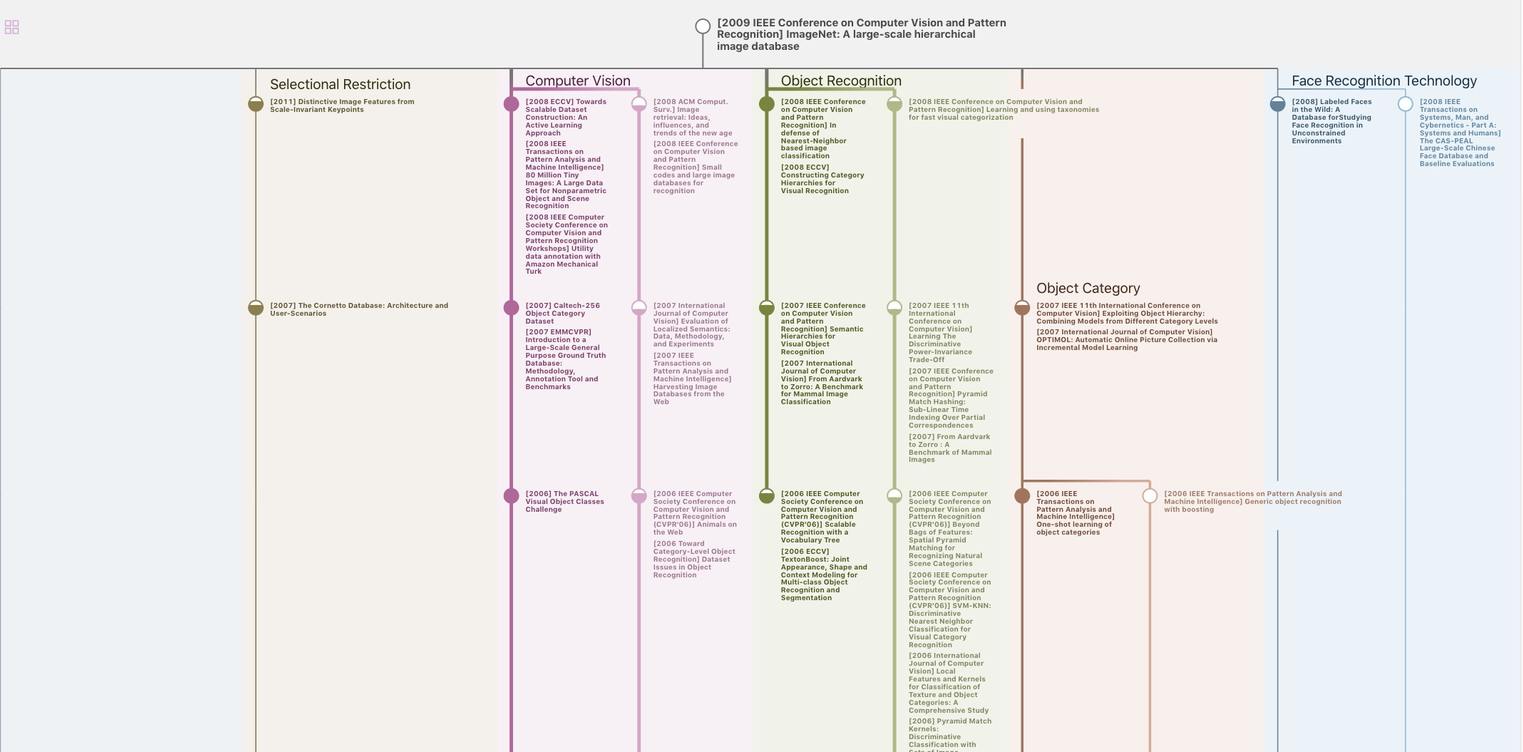
生成溯源树,研究论文发展脉络
Chat Paper
正在生成论文摘要