Finite Population Regression Adjustment and Non-asymptotic Guarantees for Treatment Effect Estimation.
NeurIPS(2023)
Abstract
The design and analysis of randomized experiments is fundamental to many areas, from the physical and social sciences to industrial settings.
Regression adjustment is a popular technique to reduce the variance of estimates obtained from experiments, by utilizing information contained in auxiliary covariates.
While there is a large literature within the statistics community studying various approaches to regression adjustment and their asymptotic properties, little focus has been given to approaches in the finite population setting with non-asymptotic accuracy bounds.
Further, prior work typically assumes that an entire population is exposed to an experiment, whereas practitioners often seek to minimize the number of subjects exposed to an experiment, for ethical and pragmatic reasons.
In this work, we study the problems of estimating the sample mean, individual treatment effects, and average treatment effect with regression adjustment.
We propose approaches that use techniques from randomized numerical linear algebra to sample a subset of the population on which to perform an experiment. We give non-asymptotic accuracy bounds for our methods and demonstrate that they compare favorably with prior approaches.
MoreTranslated text
AI Read Science
Must-Reading Tree
Example
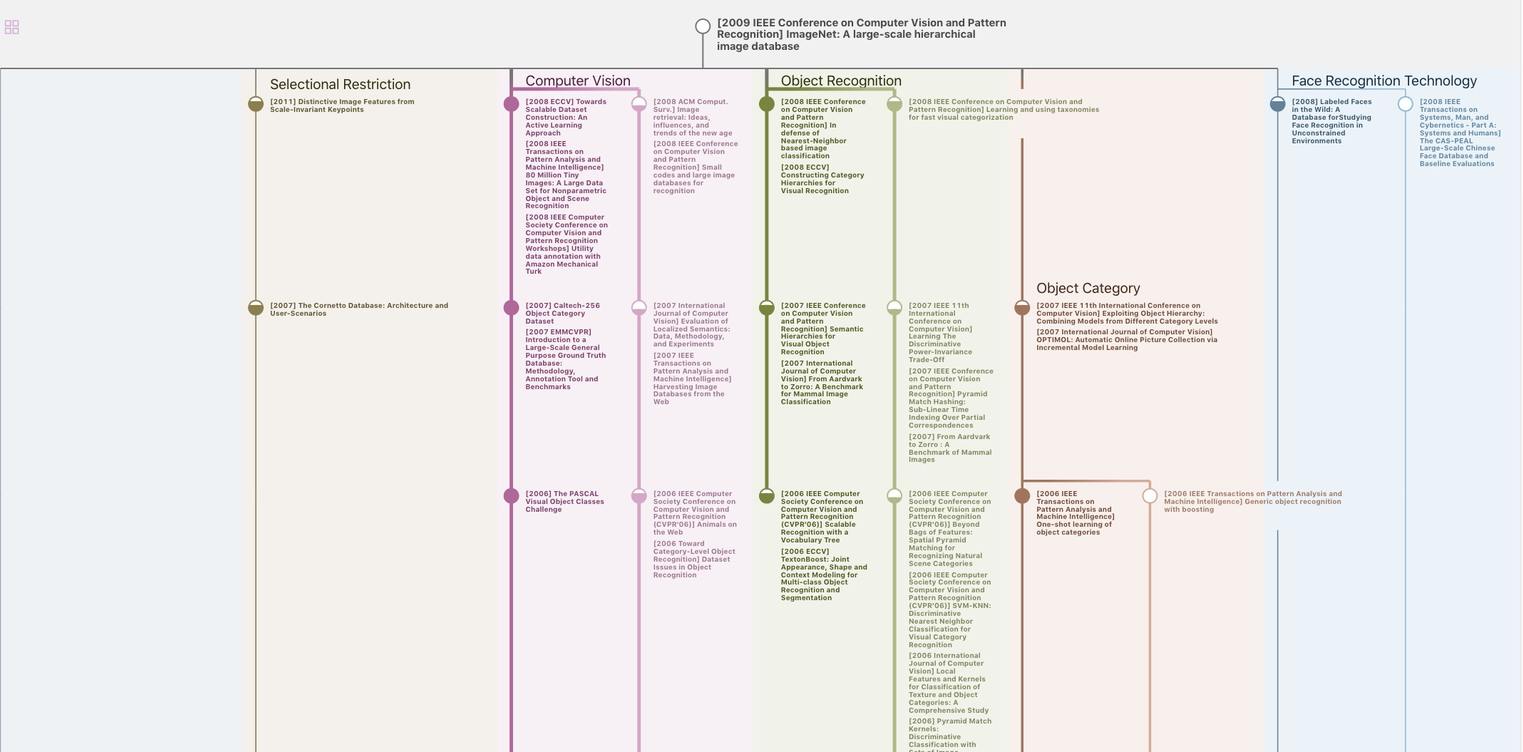
Generate MRT to find the research sequence of this paper
Chat Paper
Summary is being generated by the instructions you defined