Individualized Learning-Based Ground Reaction Force Estimation in People Post-Stroke Using Pressure Insoles
2023 INTERNATIONAL CONFERENCE ON REHABILITATION ROBOTICS, ICORR(2023)
关键词
Sensory Feedback,Gait Analysis,Postural Stability
AI 理解论文
溯源树
样例
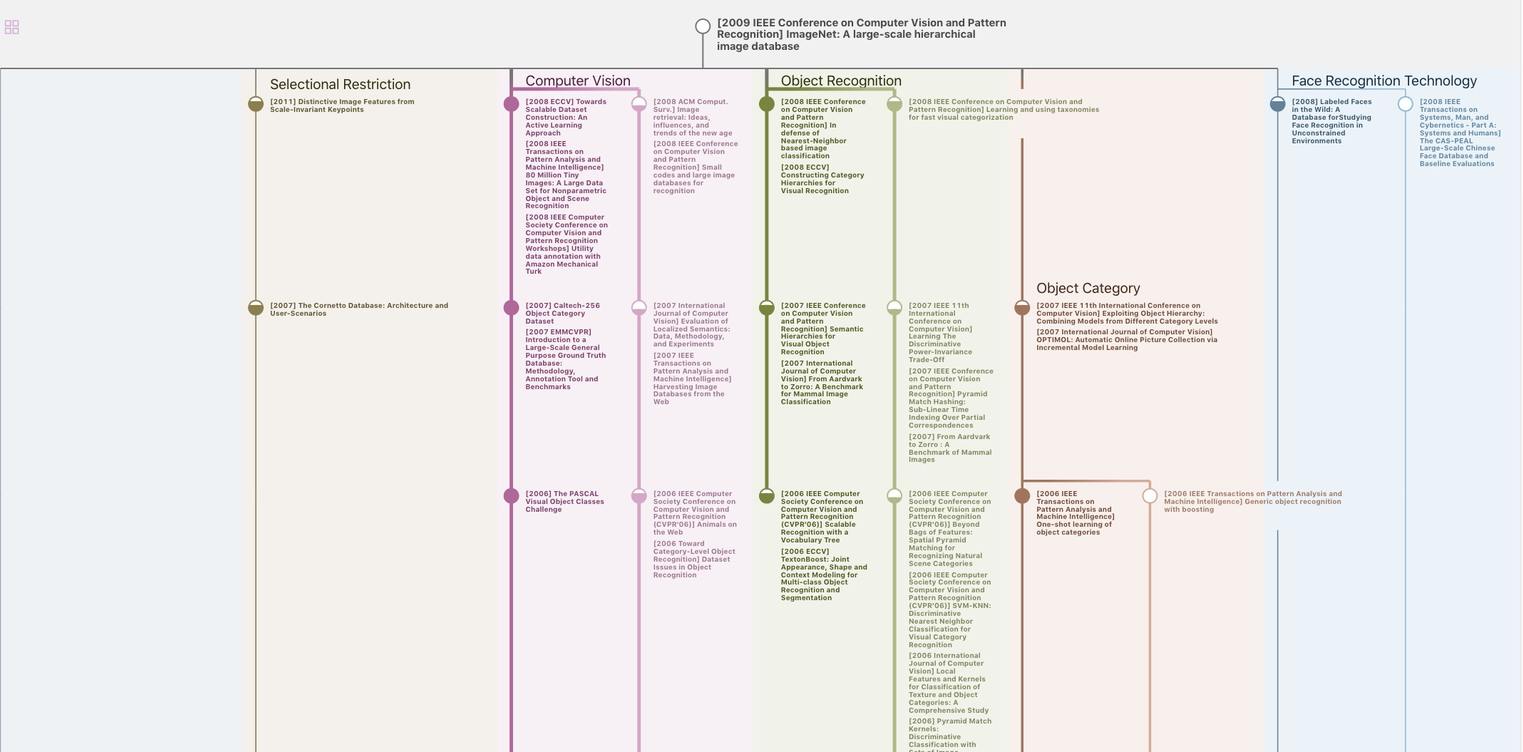
生成溯源树,研究论文发展脉络
Chat Paper
正在生成论文摘要