Histogram-Based Federated XGBoost using Minimal Variance Sampling for Federated Tabular Data
2023 EIGHTH INTERNATIONAL CONFERENCE ON FOG AND MOBILE EDGE COMPUTING, FMEC(2023)
摘要
Federated Learning (FL) has gained considerable traction, yet, for tabular data, FL has received less attention. Most FL research has focused on Neural Networks while Tree-Based Models (TBMs) such as XGBoost have historically performed better on tabular data. It has been shown that subsampling of training data when building trees can improve performance but it is an open problem whether such subsampling can improve performance in FL. In this paper, we evaluate a histogram-based federated XGBoost that uses Minimal Variance Sampling (MVS). We demonstrate the underlying algorithm and show that our model using MVS can improve performance in terms of accuracy and regression error in a federated setting. In our evaluation, our model using MVS performs better than uniform (random) sampling and no sampling at all. It achieves both outstanding local and global performance on a new set of federated tabular datasets. Federated XGBoost using MVS also outperforms centralized XGBoost in half of the studied cases.
更多查看译文
关键词
Federated Learning,XGBoost,Tabular Data,Minimal Variance Sampling
AI 理解论文
溯源树
样例
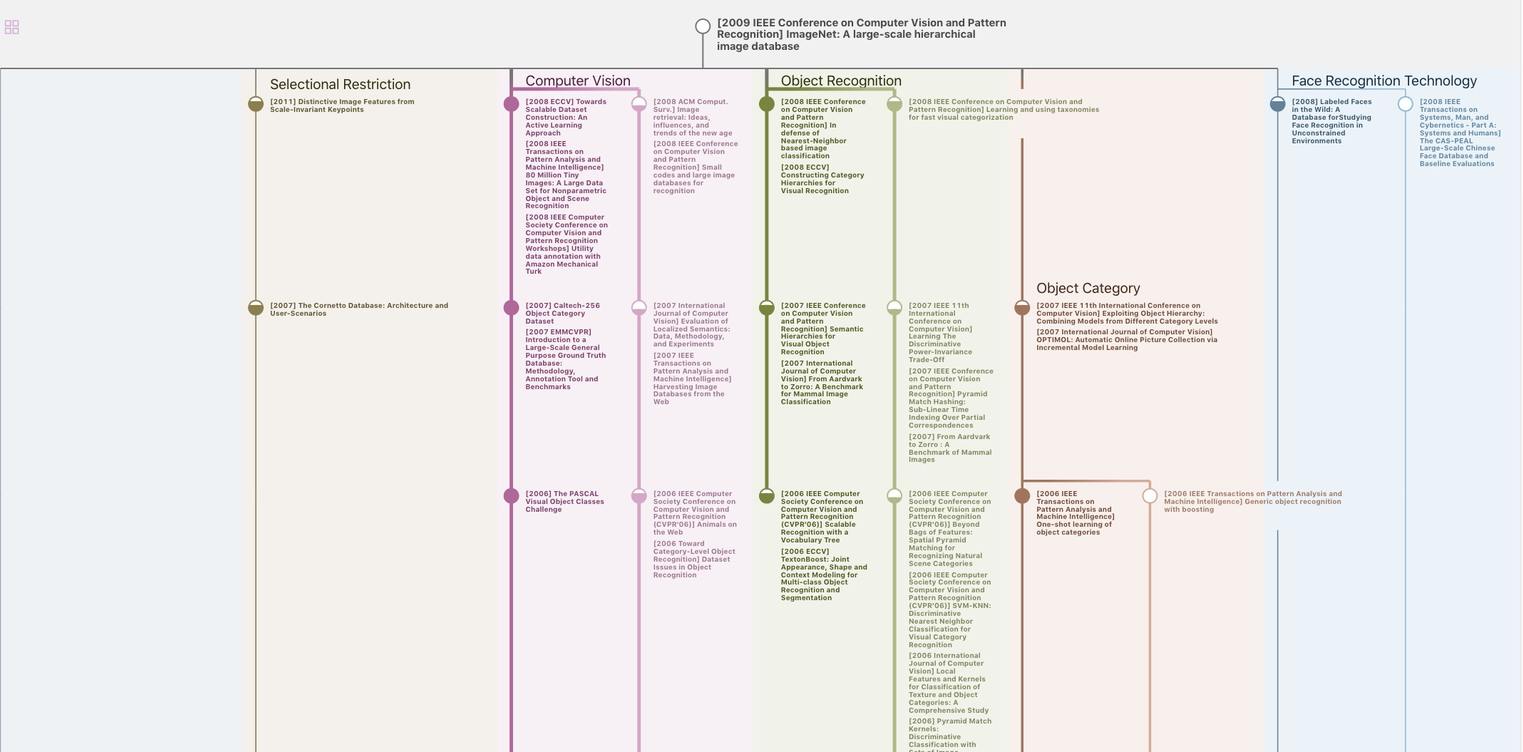
生成溯源树,研究论文发展脉络
Chat Paper
正在生成论文摘要