Enabling on-demand Crowdsourced Federated Learning Over IoT
2023 EIGHTH INTERNATIONAL CONFERENCE ON FOG AND MOBILE EDGE COMPUTING, FMEC(2023)
摘要
Federated learning (FL) is attributed to training a machine learning (ML) model over a large number of distributed devices. Under these settings, the edge devices perform computations on their local data before sending the required updates to the central server to improve the global model. This approach has shown great potential since hundreds of devices can collectively contribute to learning a single task without sharing their local data. However, IoT devices often have only small data samples, and collaboration among a large number of devices is required to collectively provide the extensive training data needed to train a high-performing model. Despite the success in many domains, FL is facing challenges when it comes to audit and scaling the clients to increase the performance of the federated network. In this paper, we argue that there's a need for a dynamic learning platform where IoT devices could volunteer to collaboratively learn a task through FL. Building on that, we propose a dynamic marketplace for on-demand, crowdsourced FL namely FedOnDemand, to train high-performing ML models over IoT devices. The proposed system coordinates client selection, task assignment, and model training to allow computationally efficient FL while maintaining communication efficiency. To drive FedOnDemand, we use the data valuation mechanism based on the Shapley Value to incentivize participants and ensure a fair reward distribution.
更多查看译文
关键词
Federated Learning,Crowdsourcing,Crowdsourced Federated Learning,Internet of Things,IoT,Marketplace
AI 理解论文
溯源树
样例
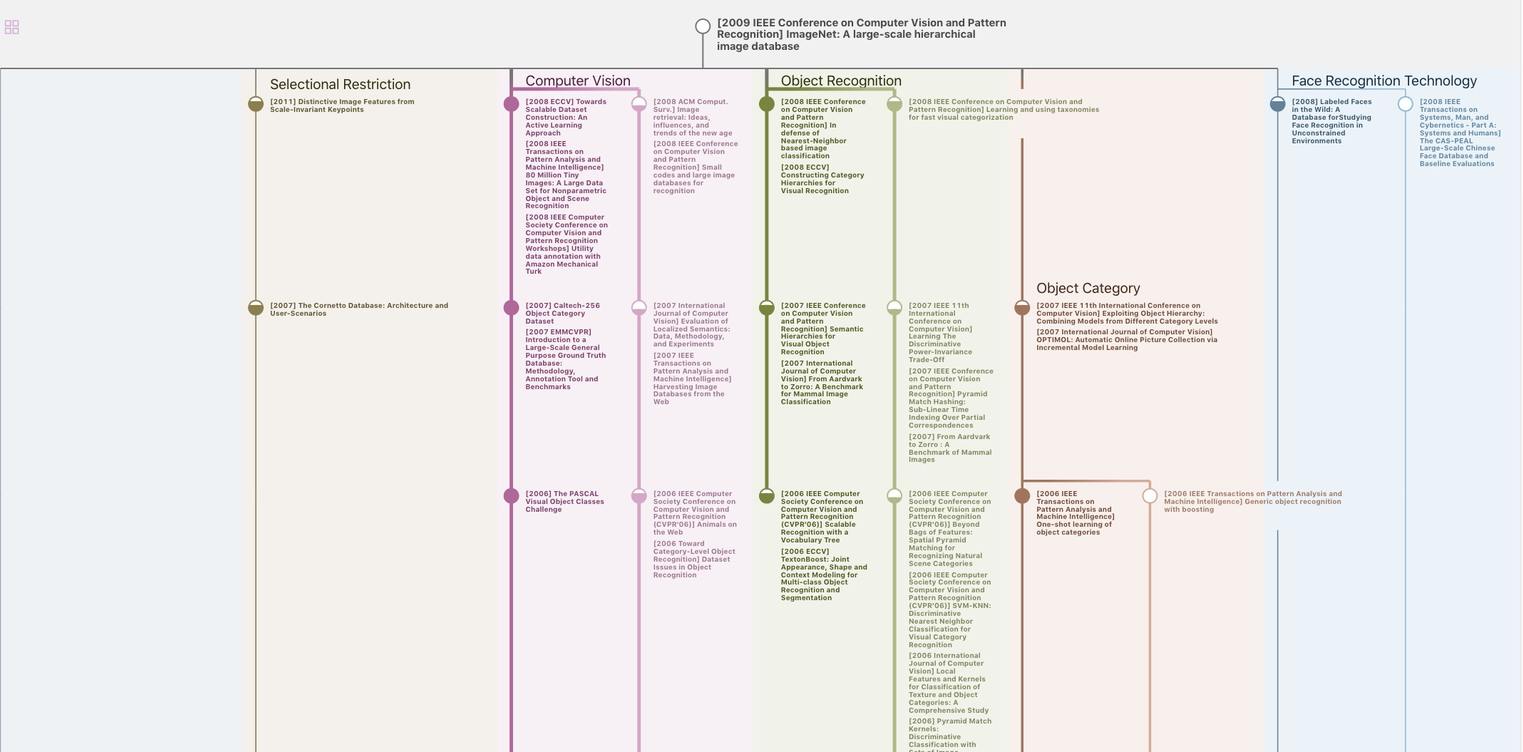
生成溯源树,研究论文发展脉络
Chat Paper
正在生成论文摘要