Proprioceptive Invariant Robot State Estimation.
CoRR(2023)
摘要
This paper reports on developing a real-time invariant proprioceptive robot
state estimation framework called DRIFT. A didactic introduction to invariant
Kalman filtering is provided to make this cutting-edge symmetry-preserving
approach accessible to a broader range of robotics applications. Furthermore,
this work dives into the development of a proprioceptive state estimation
framework for dead reckoning that only consumes data from an onboard inertial
measurement unit and kinematics of the robot, with two optional modules, a
contact estimator and a gyro filter for low-cost robots, enabling a significant
capability on a variety of robotics platforms to track the robot's state over
long trajectories in the absence of perceptual data. Extensive real-world
experiments using a legged robot, an indoor wheeled robot, a field robot, and a
full-size vehicle, as well as simulation results with a marine robot, are
provided to understand the limits of DRIFT.
更多查看译文
关键词
state estimation,robot
AI 理解论文
溯源树
样例
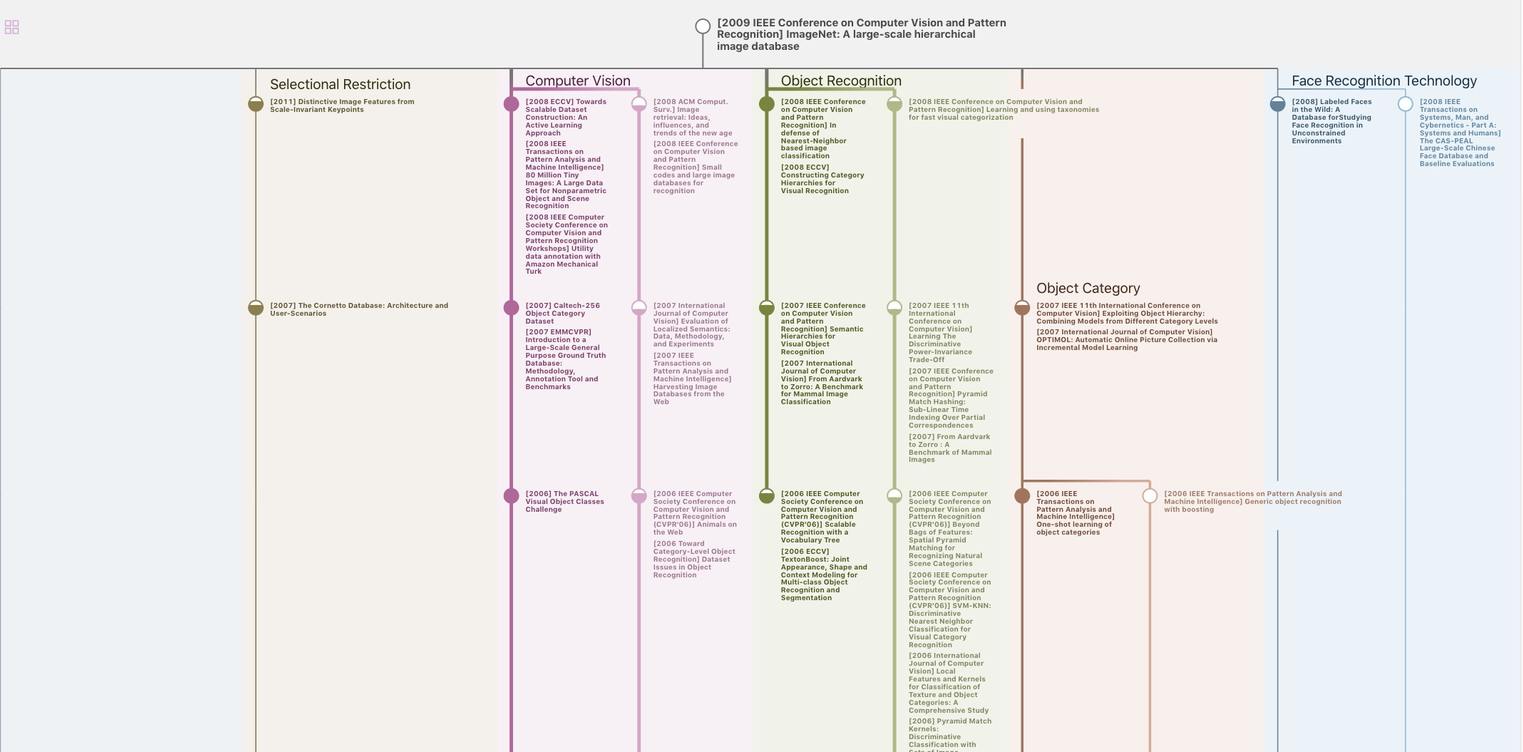
生成溯源树,研究论文发展脉络
Chat Paper
正在生成论文摘要