ETDPC: A Multimodality Framework for Classifying Pages in Electronic Theses and Dissertations
AAAI 2024(2024)
摘要
Electronic theses and dissertations (ETDs) have been proposed, advocated, and generated for more than 25 years. Although ETDs are hosted by commercial or institutional digital library repositories, they are still an understudied type of scholarly big data, partially because they are usually longer than conference and journal papers. Segmenting ETDs will allow researchers to study sectional content. Readers can navigate to particular pages of interest, to discover and explore the content buried in these long documents. Most existing frameworks on document page classification are designed for classifying general documents, and perform poorly on ETDs. In this paper, we propose ETDPC. Its backbone is a two-stream multimodal model with a cross-attention network to classify ETD pages into 13 categories. To overcome the challenge of imbalanced labeled samples, we augmented data for minority categories and employed a hierarchical classifier. ETDPC outperforms the state-of-the-art models in all categories, achieving an F1 of 0.84 -- 0.96 for 9 out of 13 categories. We also demonstrated its data efficiency. The code and data can be found on GitHub (https://github.com/lamps-lab/ETDMiner/tree/master/etd_segmentation).
更多查看译文
关键词
Machine Learning,Natural Language,Vision,Transfer Learning,Track: Emerging Applications
AI 理解论文
溯源树
样例
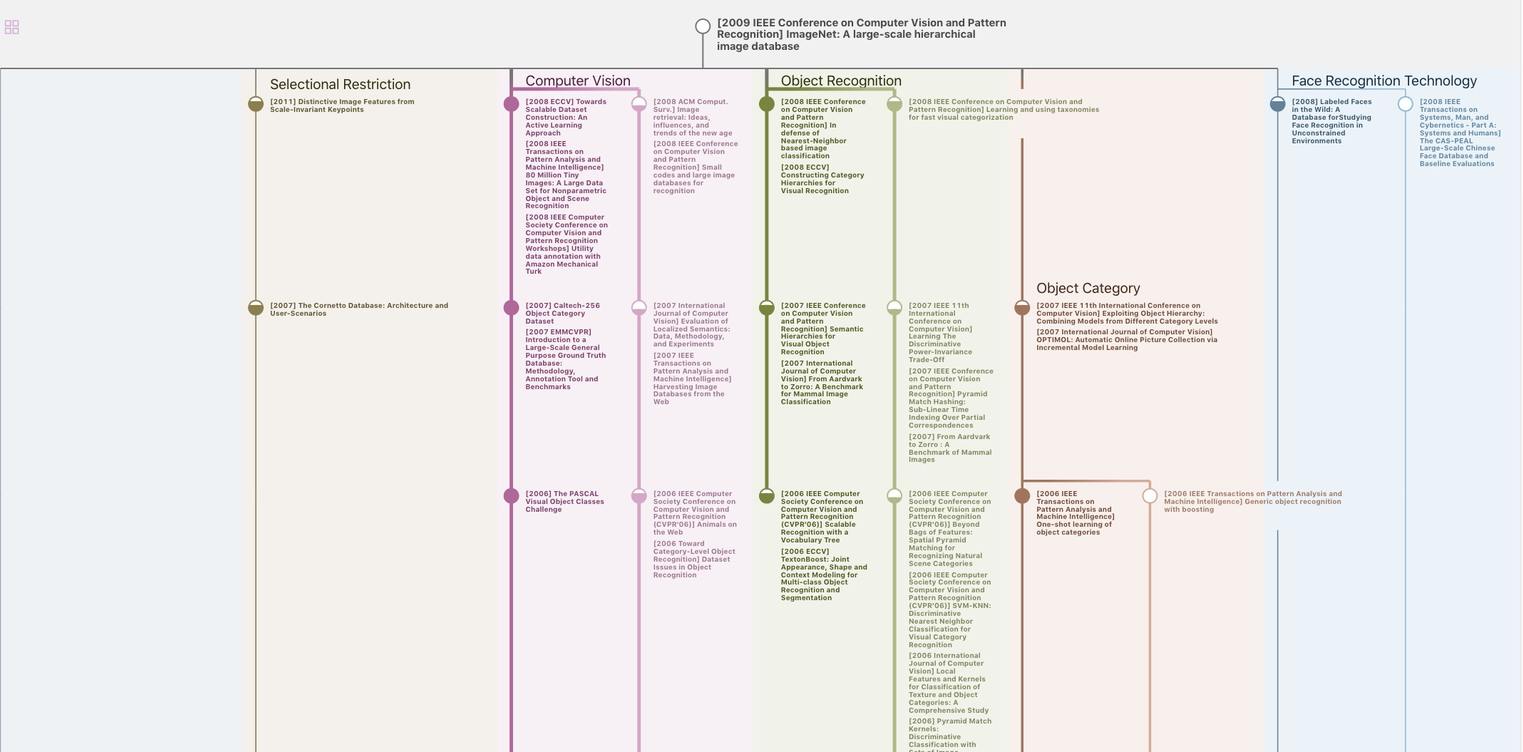
生成溯源树,研究论文发展脉络
Chat Paper
正在生成论文摘要