Pyramid-attentive GAN for Multimodal Brain Image Complementation in Alzheimer’s Disease Classification
BIOMEDICAL SIGNAL PROCESSING AND CONTROL(2024)
关键词
Alzheimer’s disease classification,Generative adversarial network,Pyramid attention,Image fusion,Data completion
AI 理解论文
溯源树
样例
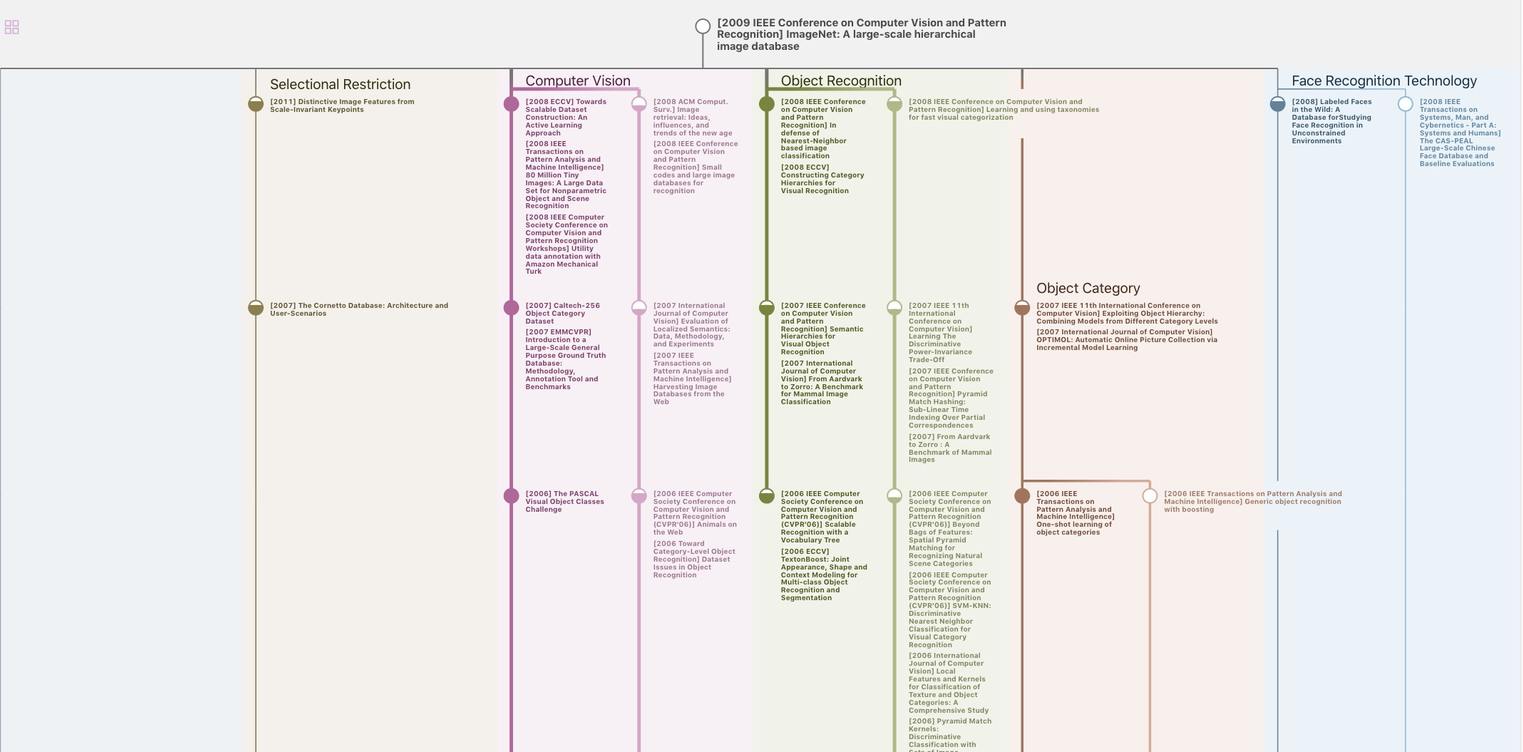
生成溯源树,研究论文发展脉络
Chat Paper
正在生成论文摘要