Incremental learning for crop growth parameters estimation and nitrogen diagnosis from hyperspectral data
COMPUTERS AND ELECTRONICS IN AGRICULTURE(2023)
摘要
Nitrogen is an essential nutrient in crop growth cycle and directly affects the photosynthesis of crops. The leaf chlorophyll content (LCC) and leaf area index (LAI) are important for characterizing photosynthetic capacity of crops and are critical indicators for diagnosing nitrogen status of crops. Hyperspectral remote sensing technology provided a means to achieve crop LCC and LAI estimation. However, the redundancy of spectral data and canopy structure effect can cause poor robustness of the estimating models, further hindering the development and application of estimating models across different crop species. In this study, a method based on incremental learning was proposed for the simultaneous estimation of LCC and LAI, and for nitrogen diagnosis across crops. First, for the spectral dataset generated by the PROSAIL model, a deep neural network was used to construct LCC and LAI estimation models (called DNNCA model). Secondly, for the hyperspectral data collected from field crops (soybean, canola and wheat), incremental learning using regularization (LwF algorithm) was used to update the DNNCA model parameters. Finally, the dilution curve model based on the LCC-LAI anisotropic growth relationship was developed to assess crop nitrogen status. The results showed that: (1) The constrained bi-objective optimizated DNNCA model can consider the interactive effect of LAI and LCC on spectral reflectance, and achieved reliable estimation on PROSAIL simulation data set (LAI:R2 = 0.82, RMSE = 0.77 m2/m2; LCC:R2 = 0.91, RMSE = 6.4 ug/cm2). (2) By incremental learning, DNNCA model has continuous learning capability and stable estimation on cross-crop (canola, soybean and wheat) field-measured data (LAI:R2 = 0.64-0.82, RMSE = 0.58-1.02 m2/m2; LCC:R2 = 0.56-0.82, RMSE = 3.9-10.5 ug/cm2). (3) The relationship between the NNILCC and the NNILNC was significant. The NNILCC derived from the anisotropy relationship between crop LAI and LCC was an effective tool for crop nitrogen status diagnosis. (4) The process of crop LAI, LCC and NNILCC reflected the effect of water and nitrogen supply on crop growth. The appropriate water-nitrogen treatments contributed to LAI increase and LCC accumulation. The study demonstrated that hyperspectral remote sensing technology combined with incremental learning is an effective method for cross-crop growth monitoring and nitrogen diagnosis. These results provide a reference and basis for filed water-nitrogen supply and management.
更多查看译文
关键词
Incremental learning,PROSAIL,Hyperspectral,Leaf area index,Leaf chlorophyll content,UAV
AI 理解论文
溯源树
样例
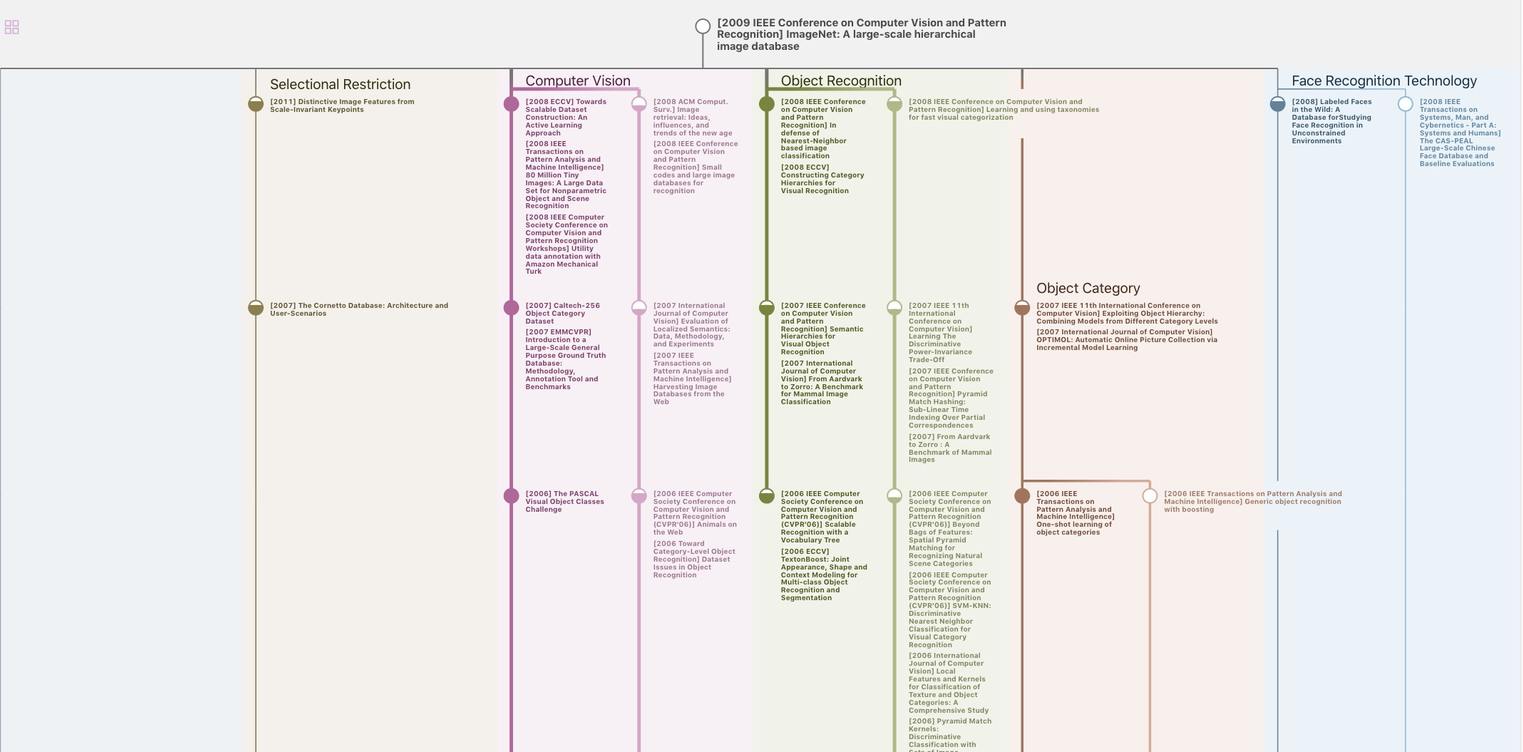
生成溯源树,研究论文发展脉络
Chat Paper
正在生成论文摘要