Estimation and variable selection for high-dimensional spatial data models
JOURNAL OF ECONOMETRICS(2024)
摘要
Spatiotemporal modeling of networks is of great practical importance, with modern applications in epidemiology and social network analysis. Despite rapid methodological advances, how to effectively and efficiently estimate the parameters of spatial dynamic panel models remains a challenging problem. To tackle this problem, we construct consistent complex least-squares estimators by the eigendecomposition of a spatial weight matrix method originally proposed for undirected networks. We no longer require all eigenvalues and eigenvectors to be real, which is a remarkable achievement as it implies that the proposed method is now applicable to spatiotemporal data modeling of directed networks. Under mild, interpretable conditions, we show that the proposed parameter estimators are consistent and asymptotically normally distributed. We also present a complex orthogonal greedy algorithm for variable selection and rigorously investigate its convergence properties. Moreover, we incorporate fixed effects into the spatial dynamic panel models and provide a model transformation so that the proposed method can also be applied to the transformed model. Extensive simulation studies and data examples demonstrate the effectiveness of the proposed method.
更多查看译文
关键词
Spatial dynamic panel data model,Network structure,Eigendecomposition,Complex linear least square,Consistency
AI 理解论文
溯源树
样例
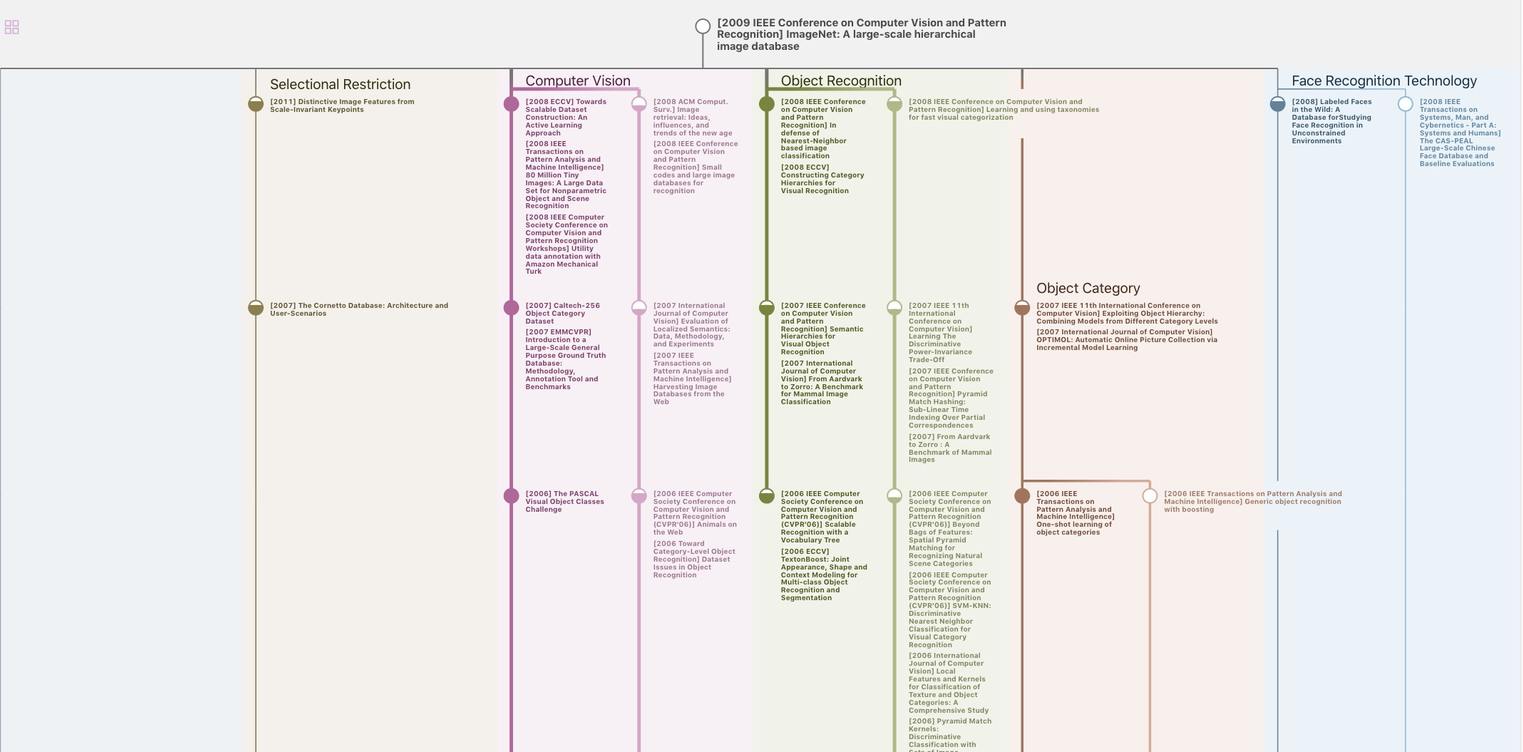
生成溯源树,研究论文发展脉络
Chat Paper
正在生成论文摘要