Development of a privacy preserving large language model for automated data extraction from thyroid cancer pathology reports
medRxiv (Cold Spring Harbor Laboratory)(2023)
摘要
Introduction: Popularized by ChatGPT, large language models (LLM) are poised to transform the scalability of clinical natural language processing (NLP) downstream tasks such as medical question answering (MQA) and may enhance the ability to rapidly and accurately extract key information from clinical narrative reports. However, the use of LLMs in the healthcare setting is limited by cost, computing power and concern for patient privacy. In this study we evaluate the extraction performance of a privacy preserving LLM for automated MQA from surgical pathology reports. Study Design: 84 thyroid cancer surgical pathology reports were assessed by two independent reviewers and the open-source FastChat-T5 3B-parameter LLM using institutional computing resources. Longer text reports were converted to embeddings. 12 medical questions for staging and recurrence risk data extraction were formulated and answered for each report. Time to respond and concordance of answers were evaluated. Results: Out of a total of 1008 questions answered, reviewers 1 and 2 had an average concordance rate of responses of 99.1% (SD: 1.0%). The LLM was concordant with reviewers 1 and 2 at an overall average rate of 88.86% (SD: 7.02%) and 89.56% (SD: 7.20%). The overall time to review and answer questions for all reports was 206.9, 124.04 and 19.56 minutes for Reviewers 1, 2 and LLM, respectively. Conclusion: A privacy preserving LLM may be used for MQA with considerable time-saving and an acceptable accuracy in responses. Prompt engineering and fine tuning may further augment automated data extraction from clinical narratives for the provision of real-time, essential clinical insights.
### Competing Interest Statement
The authors have declared no competing interest.
### Funding Statement
This study did not receive any funding.
### Author Declarations
I confirm all relevant ethical guidelines have been followed, and any necessary IRB and/or ethics committee approvals have been obtained.
Yes
The details of the IRB/oversight body that provided approval or exemption for the research described are given below:
Institutional Review Board of the Icahn School of Medicine at Mount Sinai
I confirm that all necessary patient/participant consent has been obtained and the appropriate institutional forms have been archived, and that any patient/participant/sample identifiers included were not known to anyone (e.g., hospital staff, patients or participants themselves) outside the research group so cannot be used to identify individuals.
Yes
I understand that all clinical trials and any other prospective interventional studies must be registered with an ICMJE-approved registry, such as ClinicalTrials.gov. I confirm that any such study reported in the manuscript has been registered and the trial registration ID is provided (note: if posting a prospective study registered retrospectively, please provide a statement in the trial ID field explaining why the study was not registered in advance).
Yes
I have followed all appropriate research reporting guidelines, such as any relevant EQUATOR Network research reporting checklist(s) and other pertinent material, if applicable.
Yes
All data produced in the present study are available upon reasonable request to the authors.
更多查看译文
关键词
data extraction,thyroid cancer pathology,large language model,privacy
AI 理解论文
溯源树
样例
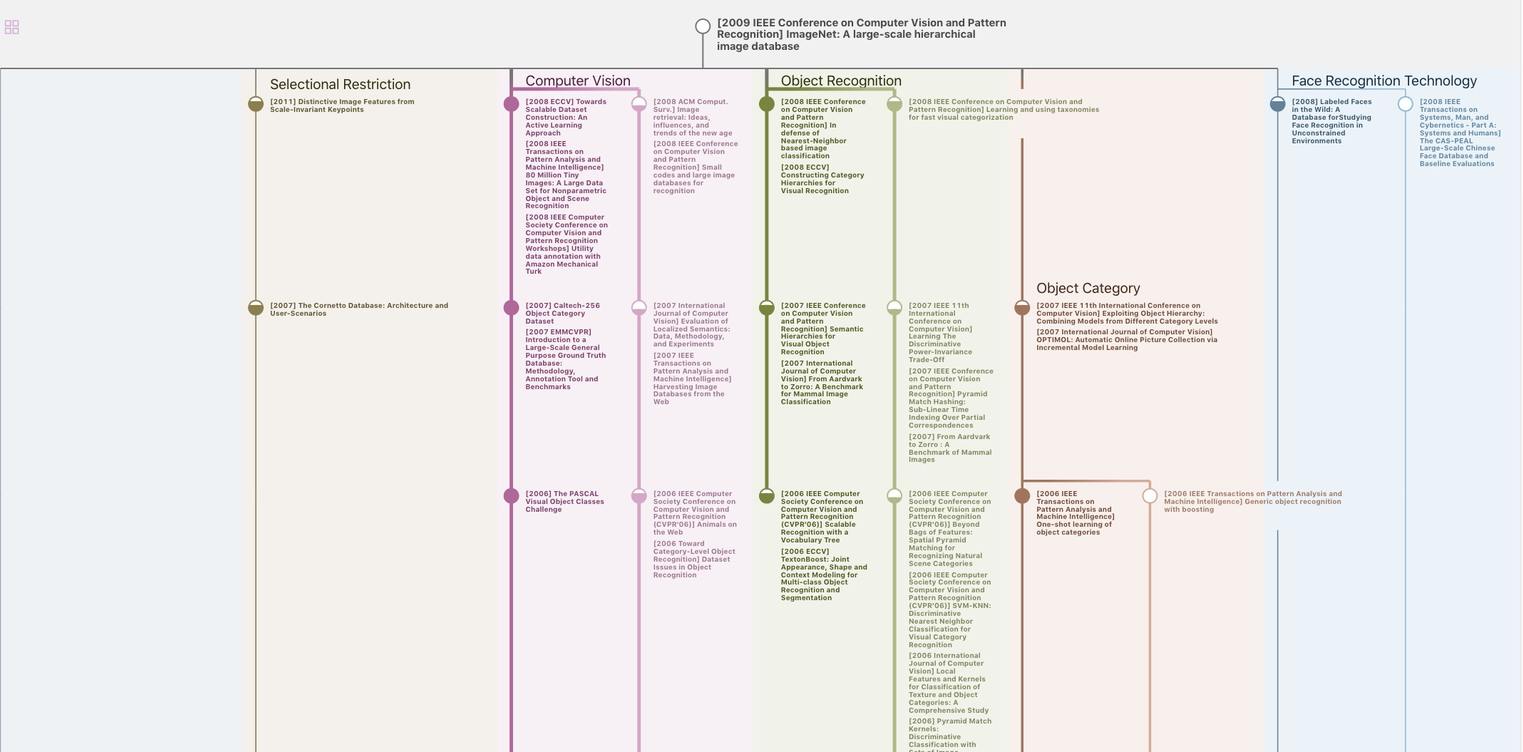
生成溯源树,研究论文发展脉络
Chat Paper
正在生成论文摘要