QRS detection in single-lead, telehealth electrocardiogram signals: benchmarking open-source algorithms
medRxiv (Cold Spring Harbor Laboratory)(2023)
摘要
Background and Objectives: A key step in electrocardiogram (ECG) analysis is the detection of QRS complexes, particularly for arrhythmia detection. Telehealth ECGs present a new challenge for automated analysis as they are noisier than traditional clinical ECGs. The aim of this study was to identify the best-performing open-source QRS detector for use with telehealth ECGs. Methods: The performance of 16 open-source QRS detectors was assessed on six datasets. These included four datasets of ECGs collected under supervision, and two datasets of telehealth ECGs collected without clinical supervision. The telehealth ECGs, consisting of single-lead ECGs recorded between the hands, included a novel dataset of 479 ECGs collected in the SAFER study of screening for atrial fibrillation (AF). Performance was assessed against manual annotations. Results: A total of 12 QRS detectors performed well on ECGs collected under clinical supervision (F1 score >= 0.96). However, fewer performed well on telehealth ECGs: five performed well on the TELE ECG Database (F1 of >= 0.99); four performed well on high-quality SAFER data (F1 of >= 0.96); and performance was poorer on low-quality SAFER data (three QRS detectors achieved F1 of 0.85-0.88). The presence of AF had little impact on performance. Conclusions: The Neurokit, `two average', and University of New South Wales QRS detectors performed best in this study. These performed sufficiently well on high-quality telehealth ECGs, but not on low-quality ECGs. This demonstrates the need to handle low-quality ECGs appropriately to ensure only ECGs which can be accurately analysed are used for clinical decision making.
### Competing Interest Statement
MRC is employed by Astrazeneca PLC. The remaining authors declare no competing interests.
### Funding Statement
This study is funded by the British Heart Foundation (grant number FS/20/20/34626), the National Institute for Health and Care Research (NIHR) Programme Grants for Applied Research Programme (Reference Number RP-PG0217-20007), and the NIHR School for Primary Care Research (SPCR-2014-10043, project 410). The views expressed are those of the author(s) and not necessarily those of the NIHR or the Department of Health and Social Care.
### Author Declarations
I confirm all relevant ethical guidelines have been followed, and any necessary IRB and/or ethics committee approvals have been obtained.
Yes
The details of the IRB/oversight body that provided approval or exemption for the research described are given below:
London Central NHS Research Ethics Committee granted ethical approval (18/LO/2066).
I confirm that all necessary patient/participant consent has been obtained and the appropriate institutional forms have been archived, and that any patient/participant/sample identifiers included were not known to anyone (e.g., hospital staff, patients or participants themselves) outside the research group so cannot be used to identify individuals.
Yes
I understand that all clinical trials and any other prospective interventional studies must be registered with an ICMJE-approved registry, such as ClinicalTrials.gov. I confirm that any such study reported in the manuscript has been registered and the trial registration ID is provided (note: if posting a prospective study registered retrospectively, please provide a statement in the trial ID field explaining why the study was not registered in advance).
Yes
I have followed all appropriate research reporting guidelines, such as any relevant EQUATOR Network research reporting checklist(s) and other pertinent material, if applicable.
Yes
All data used in the present study are either in the public domain, or private data.
更多查看译文
关键词
telehealth electrocardiogram signals,single-lead,open-source
AI 理解论文
溯源树
样例
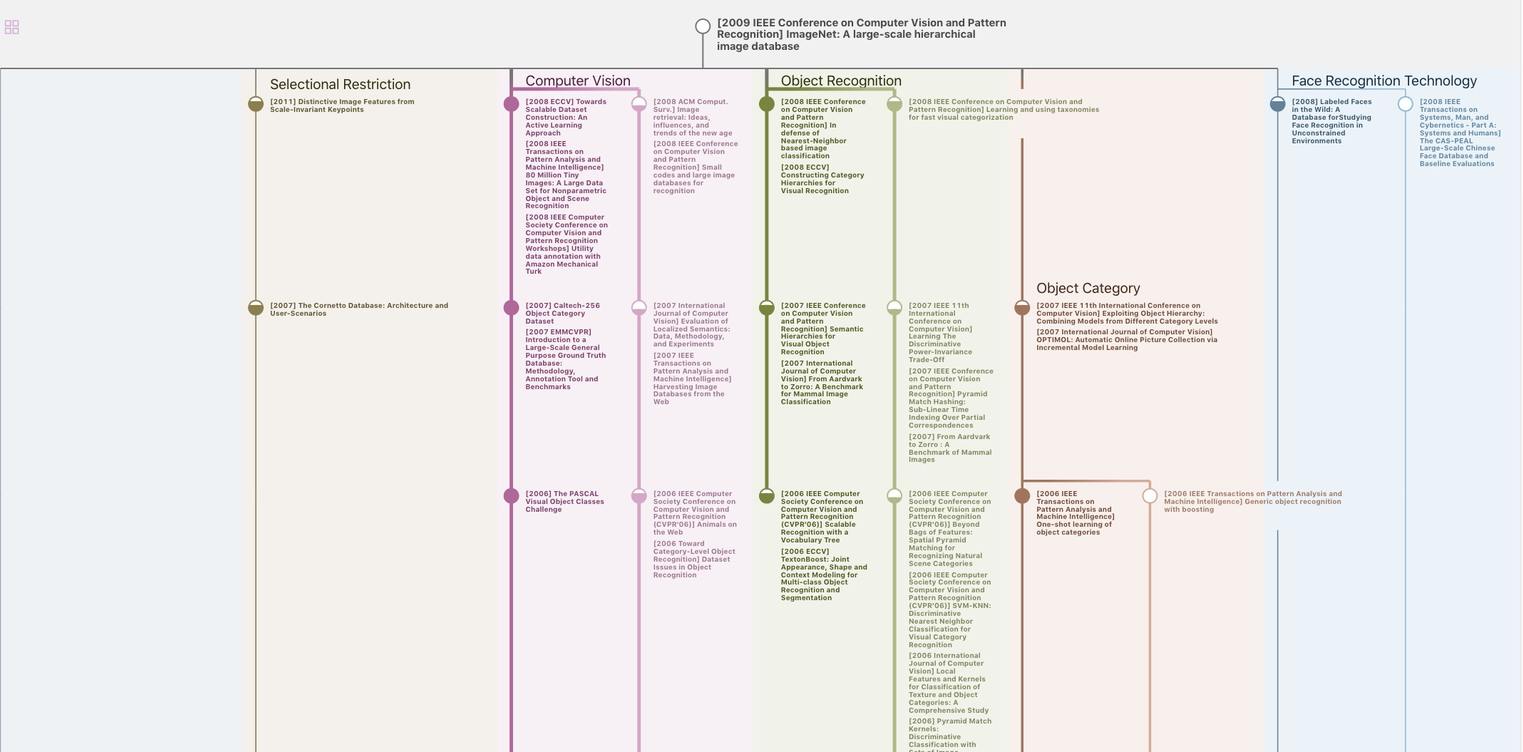
生成溯源树,研究论文发展脉络
Chat Paper
正在生成论文摘要