Cross-Graph Embedding With Trainable Proximity for Graph Alignment
IEEE TRANSACTIONS ON KNOWLEDGE AND DATA ENGINEERING(2023)
摘要
Graph alignment, also known as network alignment, has many applications in data mining tasks. It aims to find the node correspondence across disjoint graphs. With recent representation learning advancements, embedding-based graph alignment has become a hot topic. Existing embedding-based methods focus either on structural proximity across graphs or on the positional proximity within a single graph. However, only considering the structural similarity will make the position relation of nodes not clear enough, which makes it easy to misalign the nodes close in distance, while only considering the position proximity of a single graph will make the node embeddings from different graphs in different subspaces. To mitigate this issue, we propose a novel model CEGA for Cross-graph Embedding-based Graph Alignment, which can generate node embeddings to reflect structural proximity and positional proximity simultaneously. Meanwhile, we make the proximity trainable thus it can be learned to best suit the alignment task at hand automatically. We show that CEGA outperforms existing graph alignment methods in accuracy under unsupervised scenarios through extensive experiments on public benchmarks.
更多查看译文
关键词
Task analysis,Feature extraction,Sun,Optimization,Technological innovation,Switches,Predictive models,Graph alignment,node embedding,proximity
AI 理解论文
溯源树
样例
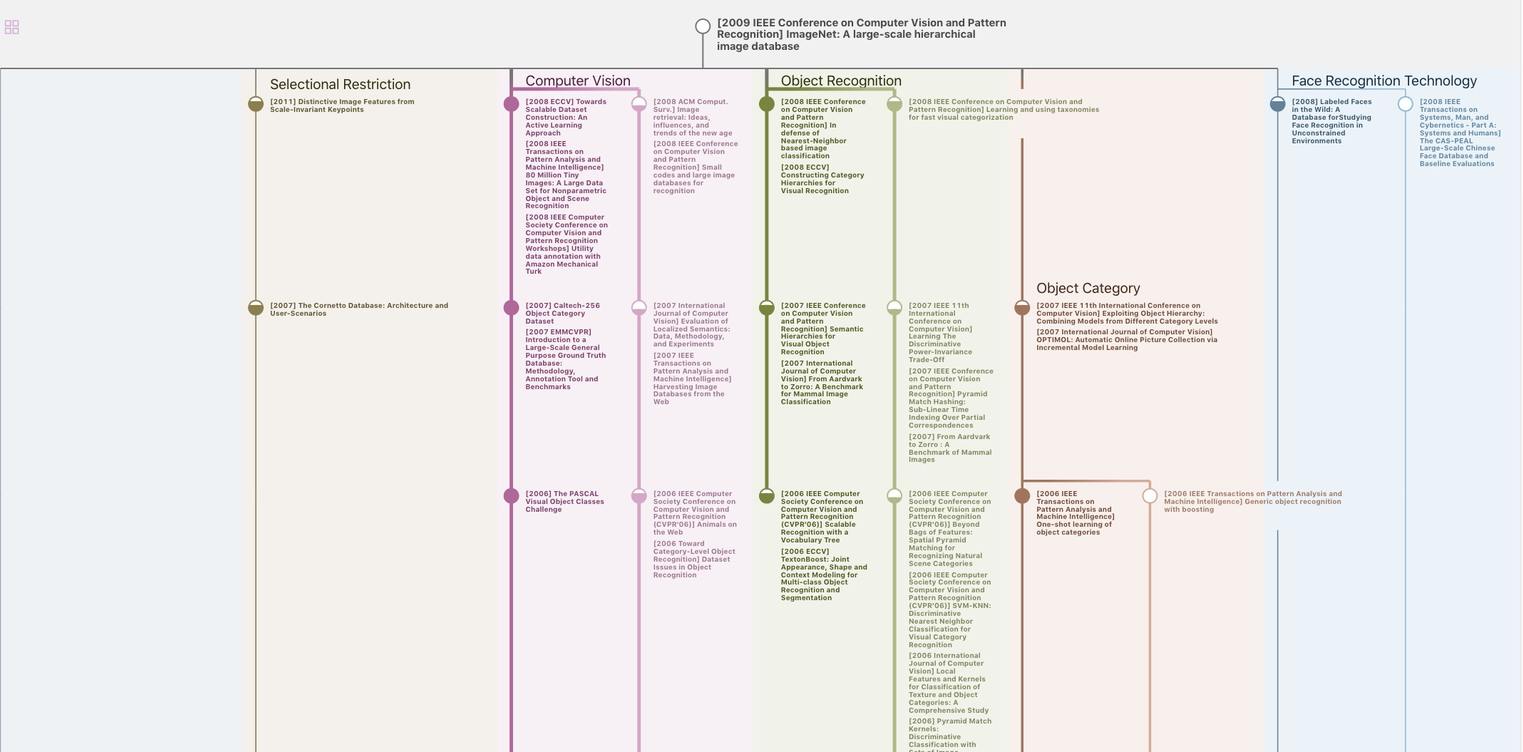
生成溯源树,研究论文发展脉络
Chat Paper
正在生成论文摘要