Hybrid multi-objective metaheuristic and possibilistic intuitionistic fuzzy c-means algorithms for cluster analysis
Soft Computing(2023)
摘要
This study proposes a hybrid multi-objective meta-heuristics and possibilistic intuitionistic fuzzy c -means (PIFCM) algorithms for cluster analysis. The PIFCM algorithms combine Atanassov’s intuitionistic fuzzy sets (IFSs) with possibilistic fuzzy c -means (PFCM) algorithms. In this study, three metaheuristic algorithms are used to improve the clustering results, specifically a genetic algorithm (GA), a particle swarm optimization (PSO) algorithm, and a gradient evolution (GE) algorithm. Therefore, three clustering algorithms are proposed, including multi-objective GA-based PIFCM (MOGA–PIFCM), multi-objective PSO-based PIFCM (MOPSO–PIFCM), and multi-objective GE-based PIFCM (MOGE–PIFCM). Their performance results are compared with those of other clustering algorithms, such as intuitionistic fuzzy c -means (IFCM) algorithm, possibilistic intuitionistic fuzzy c -means (PIFCM) algorithm, single-objective GA–PIFCM algorithm, single-objective PSO–PIFCM algorithm, and single-objective GE–PIFCM algorithm using 15 benchmark datasets. Adjusted Rand Index and accuracy measures are employed as performance indices for comparison. Experiment results shows that the MOGE–PIFCM obtains better solutions than the other clustering algorithms in terms of all performance validation indices.
更多查看译文
关键词
Cluster analysis,Meta-heuristics,Genetic algorithm,Particle swarm optimization algorithm,Gradient evolution algorithm,Intuitionistic fuzzy sets,Possibilistic fuzzy c-means algorithm,Multiple objectives
AI 理解论文
溯源树
样例
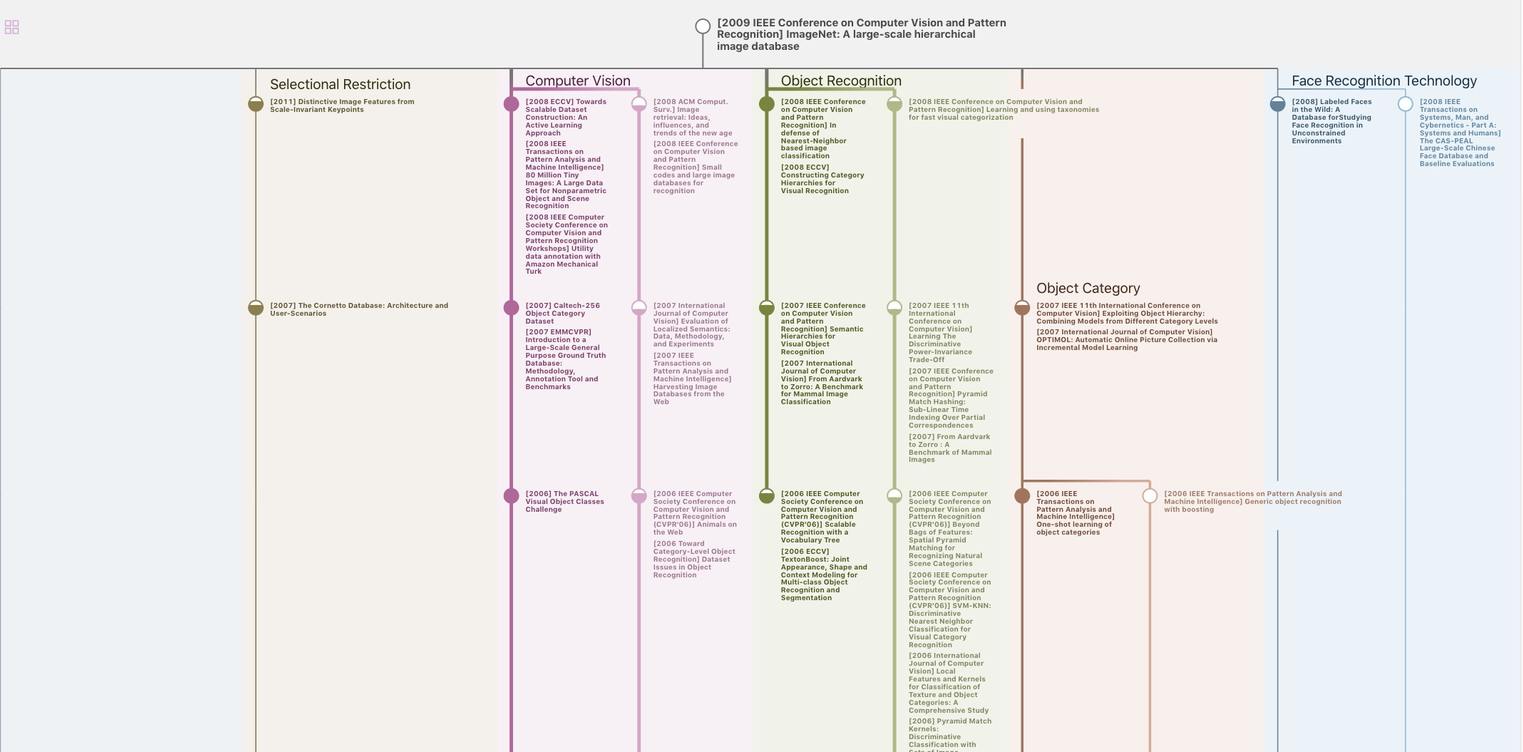
生成溯源树,研究论文发展脉络
Chat Paper
正在生成论文摘要