Incentivizing free riders improves collective intelligence in social dilemmas
PROCEEDINGS OF THE NATIONAL ACADEMY OF SCIENCES OF THE UNITED STATES OF AMERICA(2023)
摘要
Collective intelligence challenges are often entangled with collective action problems. For example, voting, rating, and social innovation are collective intelligence tasks that require costly individual contributions. As a result, members of a group often free ride on the information contributed by intrinsically motivated people. Are intrinsically motivated agents the best participants in collective decisions? We embedded a collective intelligence task in a large- scale, virtual world public good game and found that participants who joined the information system but were reluctant to contribute to the public good (free riders) provided more accurate evaluations, whereas participants who rated frequently underperformed. Testing the underlying mechanism revealed that a negative rating bias in free riders is associated with higher accuracy. Importantly, incentivizing evaluations amplifies the relative influence of participants who tend to free ride without altering the (higher) quality of their evaluations, thereby improving collective intelligence. These results suggest that many of the currently available information systems, which strongly select for intrinsically motivated participants, underperform and that collective intelligence can benefit from incentivizing free riding members to engage. More generally, enhancing the diversity of contributor motivations can improve collective intelligence in settings that are entangled with collective action problems.
更多查看译文
关键词
collective intelligence,crowd wisdom,social feedback,social dilemmas,computational social science
AI 理解论文
溯源树
样例
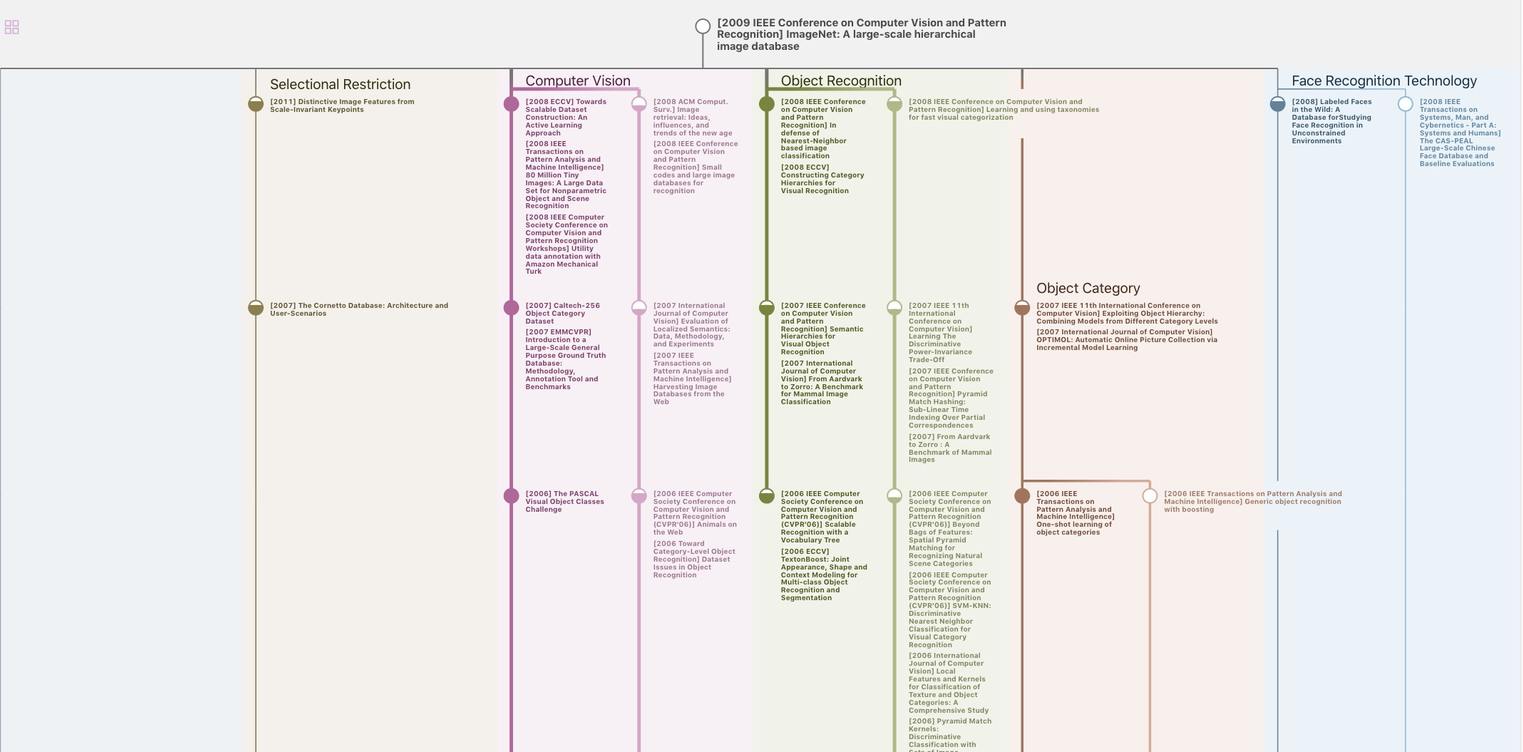
生成溯源树,研究论文发展脉络
Chat Paper
正在生成论文摘要