A stable-state multi-objective evolutionary algorithm based on decomposition
EXPERT SYSTEMS WITH APPLICATIONS(2024)
摘要
The decomposition-based multi-objective evolutionary algorithm (MOEA/D) has been shown to effectively solve real-world multi-objective optimization problems (MOPs). The uniformly distributed weight vectors guide the population to continuously evolve towards the Pareto front (PF). However, the random matching mode between individuals and weight vectors leads to some excellent individuals being replaced in the evolution process. Meanwhile, the number of replaced individuals in the neighborhood is not controlled. This causes a waste of computing resources and fails to balance the diversity and convergence of the population effectively. Referring to the influence on body adjustment of homeostasis in the medical field, the stable-state mechanism is proposed to keep the dynamic balance between exploration and exploitation. Therefore, this paper presents the stable-state multi-objective evolutionary algorithm based on decomposition (MOEA/D-SS) that adopts a new stable-state replacement strategy to adjust the number of replaced individuals within each neighborhood. Furthermore, a stable-state neighborhoods adjustment strategy was proposed to adjust the size of each neighborhood. This mechanism can adjust the convergence and diversity of newly generated individuals at different stages. Finally, several benchmark test suites (i.e., ZDT, DTLZ, and UF) and a practical optimization problem are used to test the performance of MOEA/D-SS. The experimental results demonstrate that the proposed algorithm outperforms other comparative algorithms.
更多查看译文
关键词
Multi-objective evolutionary algorithm,Decomposition,Stable-state replacement,Matching mechanism,Neighborhood adjustment
AI 理解论文
溯源树
样例
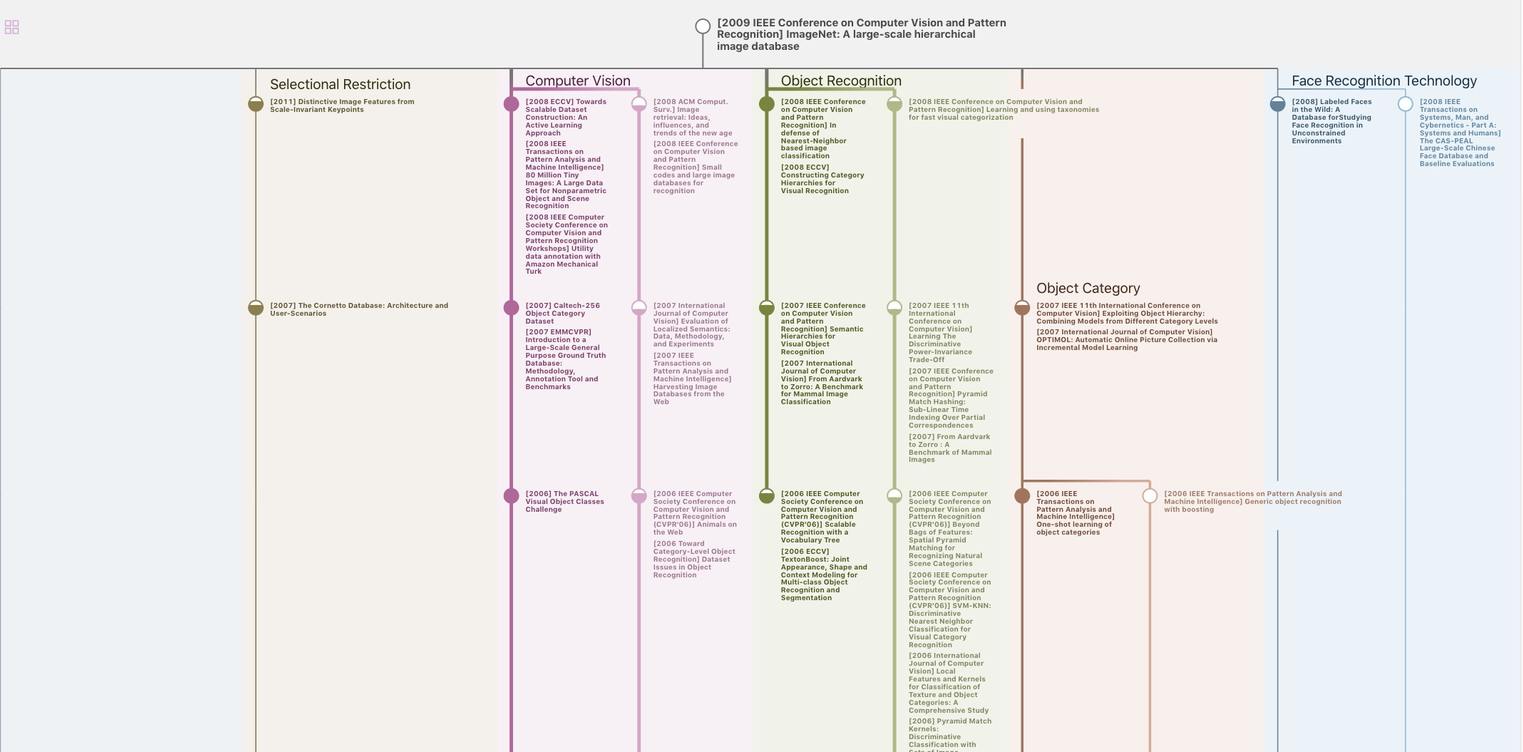
生成溯源树,研究论文发展脉络
Chat Paper
正在生成论文摘要