Adaptive 3D Geometry-based Stochastic Channel Prediction for 3D DL Selection
CoRR(2023)
摘要
This paper addresses the challenges of mobile user requirements in shadowing
and multi-fading environments, focusing on the Downlink (DL) radio node
selection based on Uplink (UL) channel estimation. One of the key issues
tackled in this research is the prediction performance in scenarios where
estimated channels are integrated. An adaptive deep learning approach is
proposed to improve performance, offering a compelling alternative to
traditional interpolation techniques for air-to-ground link selection on
demand. Moreover, our study considers a 3D channel model, which provides a more
realistic and accurate representation than 2D models, particularly in the
context of 3D network node distributions. This consideration becomes crucial in
addressing the complex multipath fading effects within geometric stochastic 3D
3GPP channel models in urban environments. Furthermore, our research emphasises
the need for adaptive prediction mechanisms that carefully balance the
trade-off between DL link forecasted frequency response accuracy and the
complexity requirements associated with estimation and prediction. This paper
contributes to advancing 3D radio resource management by addressing these
challenges, enabling more efficient and reliable communication for
energy-constrained flying network nodes in dynamic environments.
更多查看译文
关键词
stochastic channel prediction,channel prediction,3d dl,geometry-based
AI 理解论文
溯源树
样例
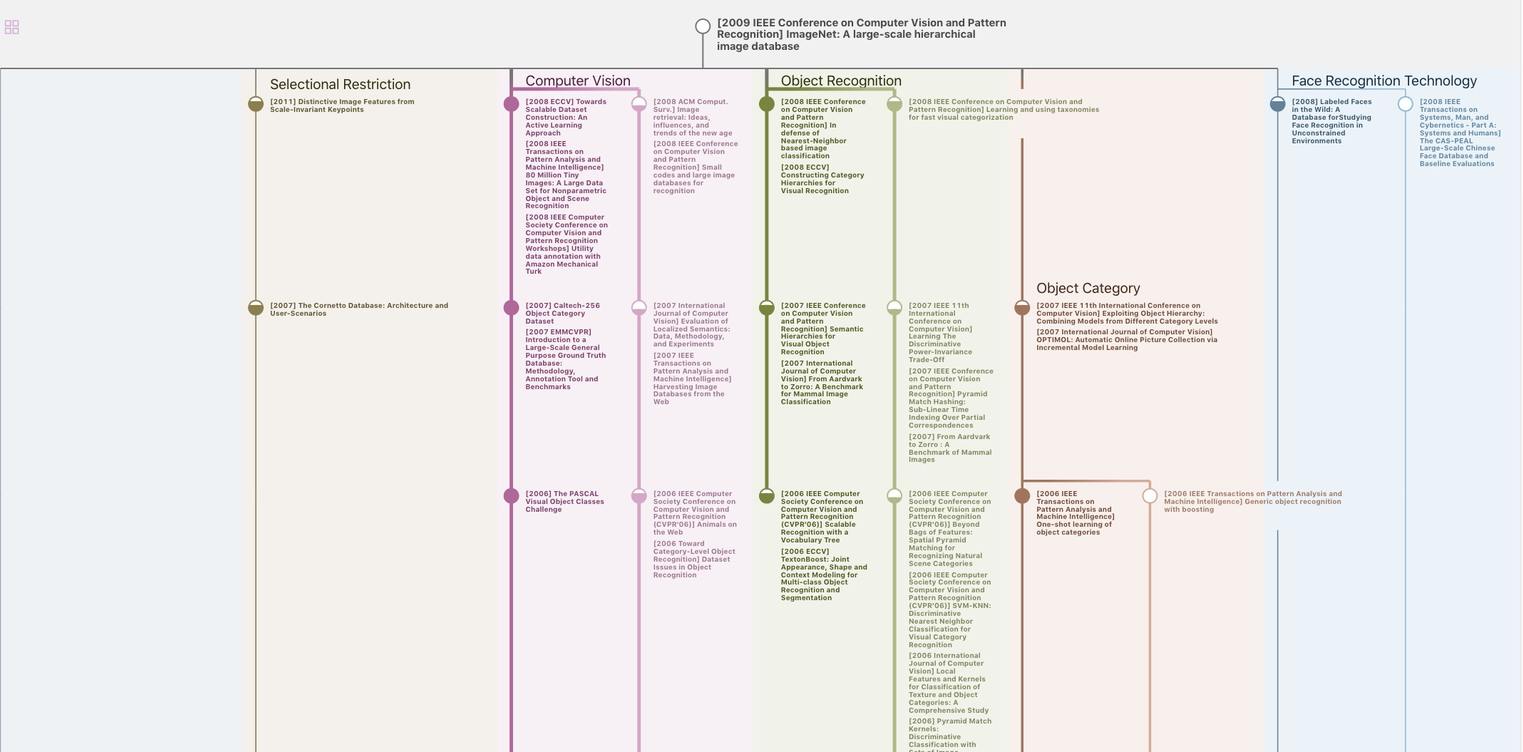
生成溯源树,研究论文发展脉络
Chat Paper
正在生成论文摘要