An AI-Guided Data Centric Strategy to Detect and Mitigate Biases in Healthcare Datasets.
CoRR(2023)
摘要
The adoption of diagnosis and prognostic algorithms in healthcare has led to concerns about the perpetuation of bias against disadvantaged groups of individuals. Deep learning methods to detect and mitigate bias have revolved around modifying models, optimization strategies, and threshold calibration with varying levels of success. Here, we generate a data-centric, model-agnostic, task-agnostic approach to evaluate dataset bias by investigating the relationship between how easily different groups are learned at small sample sizes (AEquity). We then apply a systematic analysis of AEq values across subpopulations to identify and mitigate manifestations of racial bias in two known cases in healthcare-Chest X-rays diagnosis with deep convolutional neural networks and healthcare utilization prediction with multivariate logistic regression. AEq is a novel and broadly applicable metric that can be applied to advance equity by diagnosing and remediating bias in healthcare datasets.
### Competing Interest Statement
The authors have declared no competing interest.
### Funding Statement
The National Center for Advancing Translational Sciences and the National Institutes of Health had no role in study design, data collection or analysis, preparation of the manuscript, or the decision to submit the manuscript for publication.
### Author Declarations
I confirm all relevant ethical guidelines have been followed, and any necessary IRB and/or ethics committee approvals have been obtained.
Yes
The details of the IRB/oversight body that provided approval or exemption for the research described are given below:
The publicly available images can be access via the PyTorch library. The MIMIC-CXR version 2.0 dataset is publicly available by registration at https://physionet.org/content/mimic-cxr/2.0.0/. The CheXpert dataset is publicly available by registration at https://stanfordmlgroup.github.io/competitions/chexpert/. The NIH-CXR dataset is publicly available at https://nihcc.app.box.com/v/ChestXray-NIHCC. The dataset used in the Obermeiyer analysis is available in the supplement.
I confirm that all necessary patient/participant consent has been obtained and the appropriate institutional forms have been archived, and that any patient/participant/sample identifiers included were not known to anyone (e.g., hospital staff, patients or participants themselves) outside the research group so cannot be used to identify individuals.
Yes
I understand that all clinical trials and any other prospective interventional studies must be registered with an ICMJE-approved registry, such as ClinicalTrials.gov. I confirm that any such study reported in the manuscript has been registered and the trial registration ID is provided (note: if posting a prospective study registered retrospectively, please provide a statement in the trial ID field explaining why the study was not registered in advance).
Yes
I have followed all appropriate research reporting guidelines, such as any relevant EQUATOR Network research reporting checklist(s) and other pertinent material, if applicable.
Yes
The publicly available images can be access via the PyTorch library. The MIMIC-CXR version 2.0 dataset is publicly available by registration at https://physionet.org/content/mimic-cxr/2.0.0/. The CheXpert dataset is publicly available by registration at https://stanfordmlgroup.github.io/competitions/chexpert/. The NIH-CXR dataset is publicly available at https://nihcc.app.box.com/v/ChestXray-NIHCC. The dataset used in the Obermeiyer analysis is available in the supplement.
更多查看译文
关键词
healthcare datasets,data centric strategy,mitigate biases,ai-guided
AI 理解论文
溯源树
样例
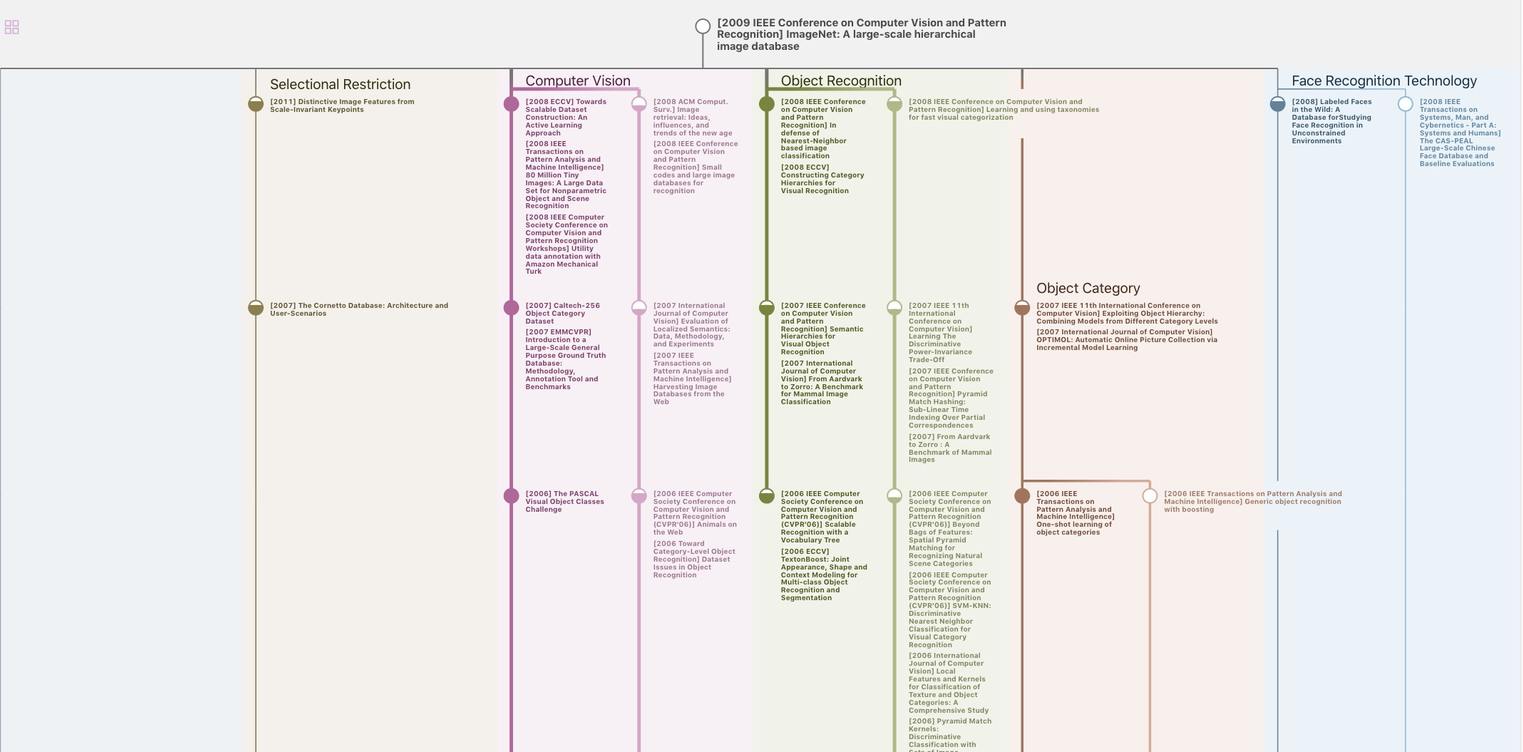
生成溯源树,研究论文发展脉络
Chat Paper
正在生成论文摘要