Reinforcement learning-based resilient power maximization and regulation control for large-scale wind turbines under cyber actuator attacks
SUSTAINABLE ENERGY GRIDS & NETWORKS(2023)
摘要
Large-scale wind turbine systems (LS-WTSs) are vulnerable to cyber threats due to their flat control network topology, fragile protocols, and remote locations. To solve these security problems and improve electrical efficiency, this study aims to present a reinforcement learning (RL)-based attack-resilient coordinated pitch and torque control for LS-WTS under actuator attacks. The proposed scheme utilizes the RL technique to reduce the number of tuning parameters and computational complexity. To do this, a model-free agent-environment framework is first proposed with an aperiodic denial-of-service (ADoS) attack model to enable the RL features. Then, an RL-based resilient sliding-mode pitch and torque control scheme is proposed to optimize maximum power extraction and regulation by incorporating reward updates and adaptive learning rates for optimal control input selection under ADoS attacks with varying wind conditions. At the same time, the adaptive learning-rule-based gain fluctuations term is presented to mitigate the uncertain and disruption parameters in the control system. Finally, the effectiveness and robustness of the proposed method are verified through the evaluation of a 4.8MW benchmark and a 20MW PMSG-based LS-WTS, showing distinct advantages over conventional approaches. Theoretical insights are practically validated with experimental prototypes to confirm the resiliency of the presented method against network and physical impacts caused by cyber actuator attacks in the LS-WTS in various wind cases.
更多查看译文
关键词
Reinforcement learning,Actuator attacks,Resilient pitch and torque control,Reliability improvement,Large-scale wind turbine system
AI 理解论文
溯源树
样例
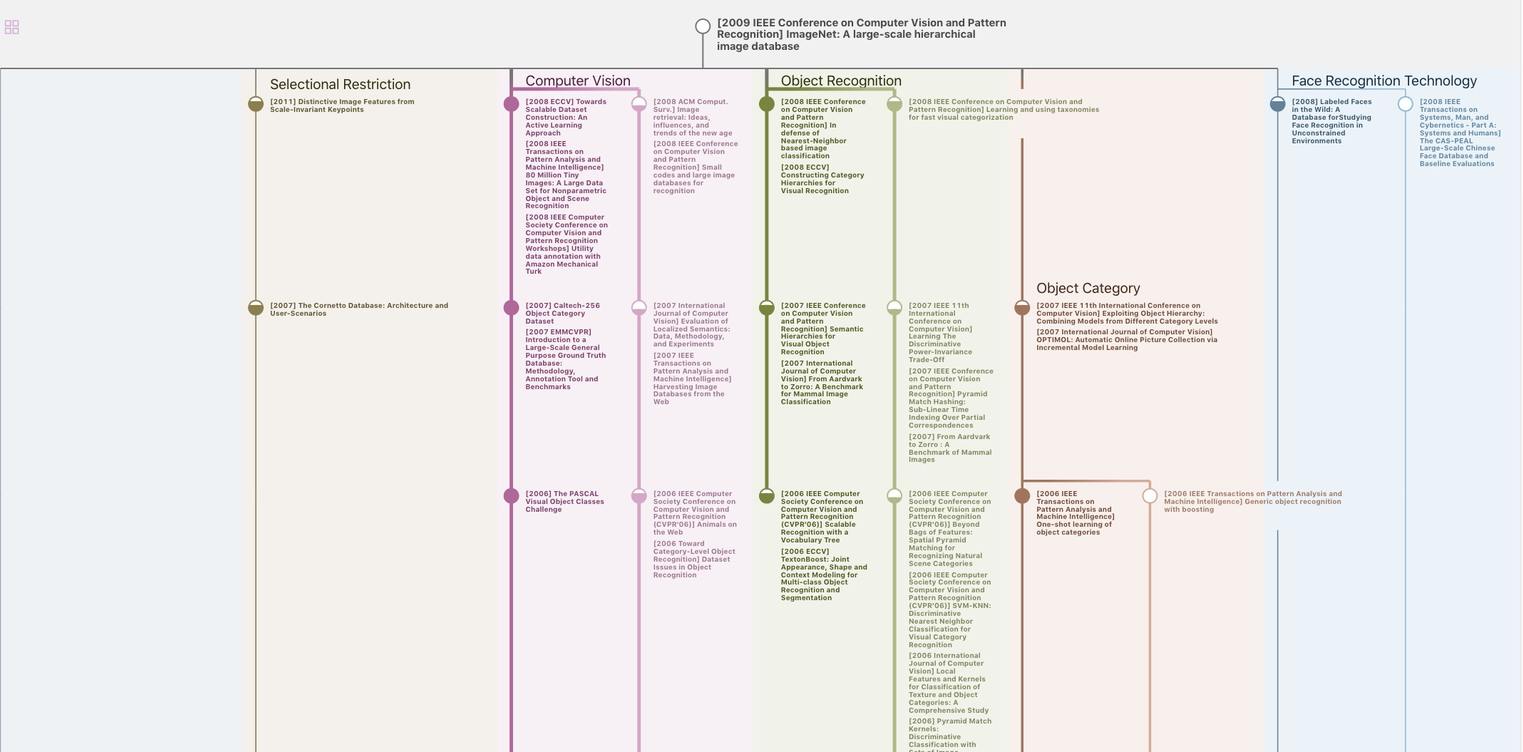
生成溯源树,研究论文发展脉络
Chat Paper
正在生成论文摘要