Towards Automatic Feature Extraction and Sample Generation of Grain Structure by Variational Autoencoder
COMPUTATIONAL MATERIALS SCIENCE(2024)
关键词
Grain structure,Machine learning,Variational autoencoder
AI 理解论文
溯源树
样例
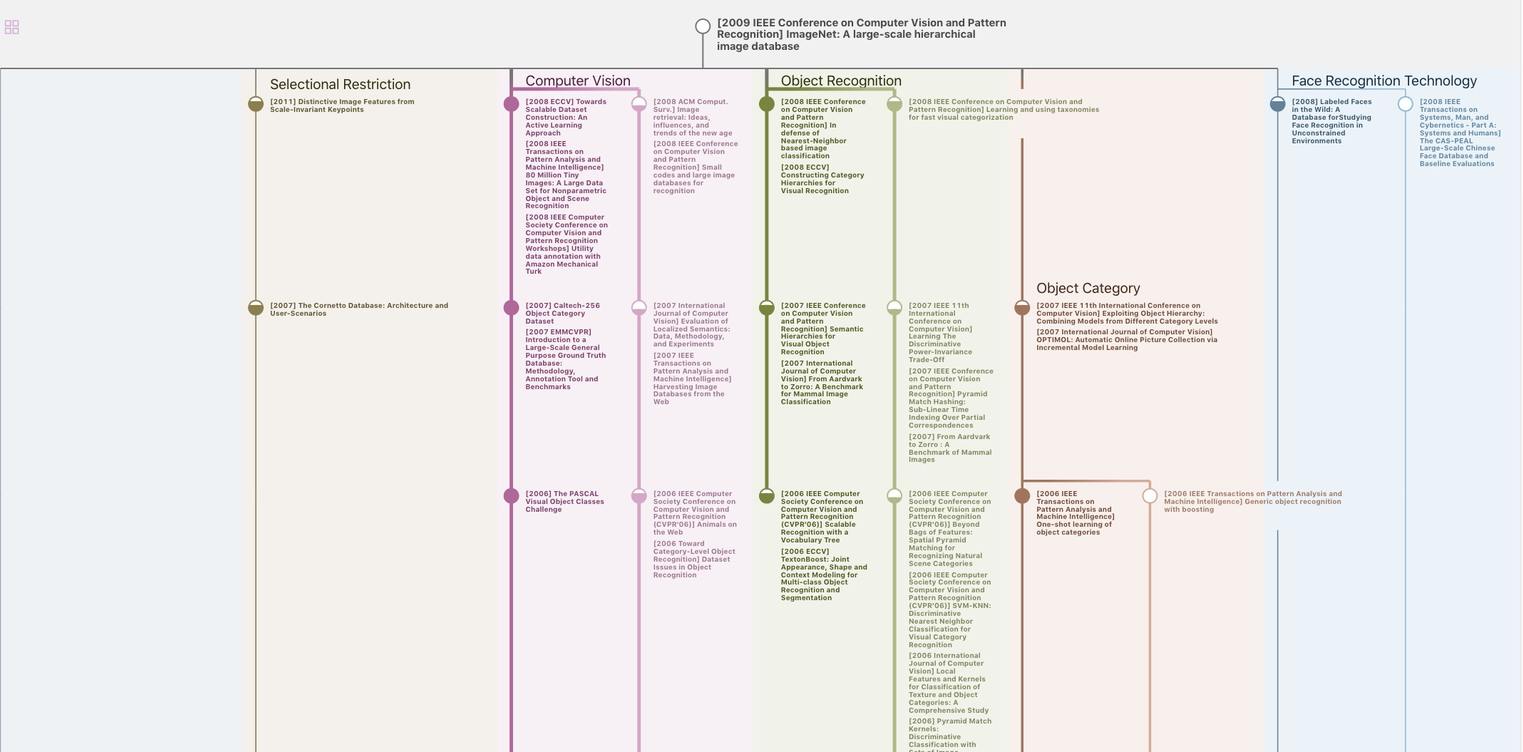
生成溯源树,研究论文发展脉络
Chat Paper
正在生成论文摘要