Sex-stratified phenotyping of comorbidities associated with an inpatient delirium diagnosis using real world data
medRxiv : the preprint server for health sciences(2023)
摘要
Delirium is a heterogeneous and detrimental mental condition often seen in older, hospitalized patients and is currently hard to predict. In this study, we leverage large-scale, real-world data using the electronic health records (EHR) to identify two cohorts comprised of 7,492 UCSF patients and 19,417 UC health system patients (excluding UCSF patients) with an inpatient delirium diagnosis and the same number of propensity score-matched control patients without delirium. We found significant associations between comorbidities or laboratory test values and an inpatient delirium diagnosis which were validated independently. Most of these associations were those previously-identified as risk factors for delirium, including metabolic abnormalities, mental health diagnoses, and infections. Some of the associations were sex-specific, including those related to dementia subtypes and infections. We further explored the diagnostic associations with anemia and bipolar disorder by conducting longitudinal analyses from the time of first diagnosis of the risk factor to development of delirium demonstrating a significant relationship across time. Finally, we show that an inpatient delirium diagnosis leads to dramatic increases in mortality outcome across both cohorts. These results demonstrate the powerful application of leveraging EHR data to shed insights into prior diagnoses and laboratory test values that could help predict development of inpatient delirium and emphasize the importance of considering patient demographic characteristics including documented sex when making these assessments.
### Competing Interest Statement
The authors have declared no competing interest.
### Funding Statement
This work was supported by the NIH grants T32GM007618 to L.K and A.T., NIA R01AG060393, R01AG057683 to MS.
### Author Declarations
I confirm all relevant ethical guidelines have been followed, and any necessary IRB and/or ethics committee approvals have been obtained.
Yes
The details of the IRB/oversight body that provided approval or exemption for the research described are given below:
All analysis of University of California, San Francisco and University of California-wide electronic health record data was performed under the approval of the Institutional Review Boards from University of California, San Francisco, University of California, Davis, University of California, Irvine, University of California, Los Angeles, and University of California, San Diego. All clinical data were de-identified and written informed consent was waived by the institutions.
I confirm that all necessary patient/participant consent has been obtained and the appropriate institutional forms have been archived, and that any patient/participant/sample identifiers included were not known to anyone (e.g., hospital staff, patients or participants themselves) outside the research group so cannot be used to identify individuals.
Yes
I understand that all clinical trials and any other prospective interventional studies must be registered with an ICMJE-approved registry, such as ClinicalTrials.gov. I confirm that any such study reported in the manuscript has been registered and the trial registration ID is provided (note: if posting a prospective study registered retrospectively, please provide a statement in the trial ID field explaining why the study was not registered in advance).
Yes
I have followed all appropriate research reporting guidelines, such as any relevant EQUATOR Network research reporting checklist(s) and other pertinent material, if applicable.
Yes
The UCSF EHR database is available to individuals affiliated with UCSF who can contact the UCSF Clinical and Translational Science Institute (CTSI) (ctsi@ucsf.edu) or the UCSF Information Commons team for more information (Info.Commons@ucsf.edu). The UC-wide EHR database is only available to UC researchers who have completed analyses in their respective UC first and have provided justification for scaling their analyses across UC health centers (more details at https://www.ucop.edu/uc-health/departments/center-for-data-driven-insights-and-innovations-cdi2.html or by contacting healthdata@ucop.edu. The code used for analysis will be made available on github.
更多查看译文
关键词
inpatient delirium diagnosis,comorbidities,phenotyping,sex-stratified
AI 理解论文
溯源树
样例
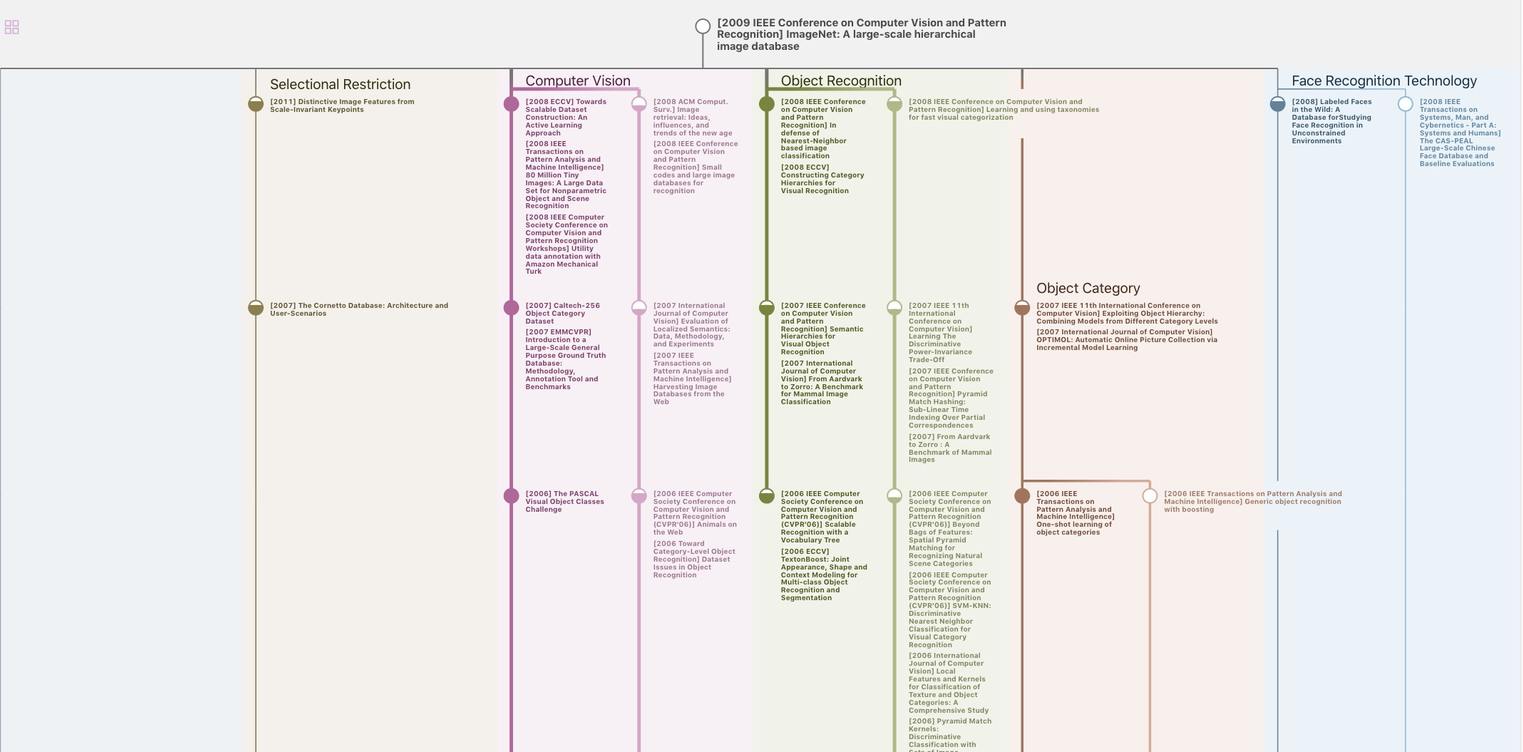
生成溯源树,研究论文发展脉络
Chat Paper
正在生成论文摘要