Apple Tree Health Recognition Through the Application of Transfer Learning for UAV Imagery.
ETFA(2023)
摘要
At present, there is a significant focus on improving agricultural productivity, which is essential both on a national and global scale. There are many factors that impact the quality and quantity of crop yields, and environmental changes such as weather conditions require careful consideration and quick action to avoid significant losses. When it comes to managing fruit orchards, large areas need to be monitored, and unmanned aerial vehicles (UAVs) are currently being used to automate and enhance the process. UAVs can capture images that can be analyzed using neural-based methods to extract useful information about the state of the trees in the orchard. However, there are some challenges that must be addressed, such as the scarcity of open-access labeled datasets and the imbalanced distribution of target classes, which include rare events or anomalous vegetation states. To tackle these issues, in this paper, a unique dataset of apple trees observations captured by UAVs has been collected and shared to the research community. This dataset includes healthy and unhealthy trees with formed and unformed crowns. Experiments were conducted using YOLOv5 neural network for object detection to evaluate its effectiveness in solving agricultural remote sensing tasks. To adjust model’s performance, we proposed a task-specific transfer learning approach that involves pre-training the model on a synthetic dataset. The synthetic dataset was generated using object-based augmentation (OBA) with the original target objects. Thereby, instead of utilizing pre-trained weights from the general domain such as COCO dataset, we use not only domain-specific UAV-derived data for pre-training but the images for the same task of apple tree health examining. The proposed approach allowed us to increase the mAP from 0.642 to 0.706 compared with the conventional approach.
更多查看译文
关键词
Apple disease,computer vision,object detection,remote sensing,unmanned aerial vehicle,agriculture
AI 理解论文
溯源树
样例
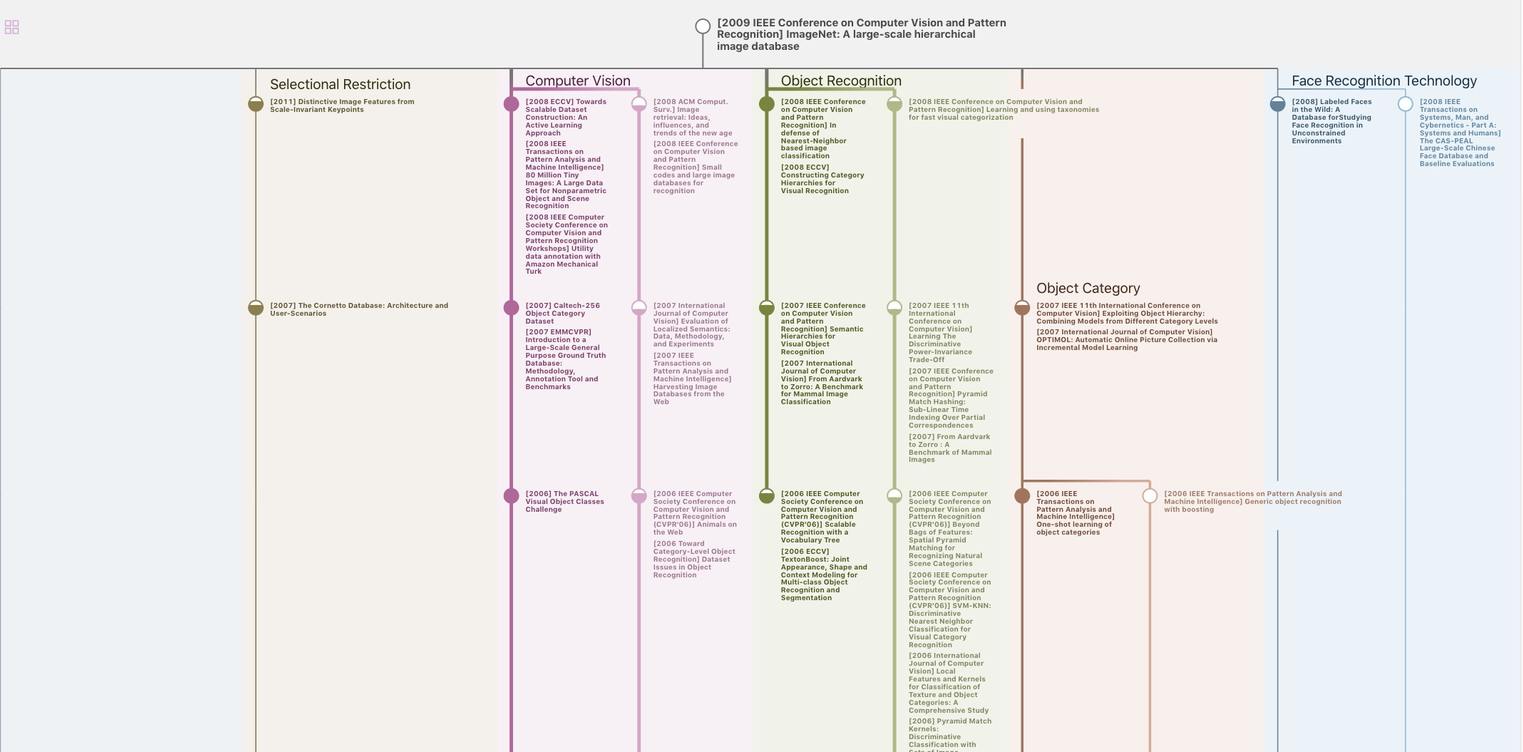
生成溯源树,研究论文发展脉络
Chat Paper
正在生成论文摘要