FairMILE: Towards an Efficient Framework for Fair Graph Representation Learning
PROCEEDINGS OF 2023 ACM CONFERENCE ON EQUITY AND ACCESS IN ALGORITHMS, MECHANISMS, AND OPTIMIZATION, EAAMO 2023(2023)
摘要
Graph representation learning models have demonstrated great capability in many real-world applications. Nevertheless, prior research indicates that these models can learn biased representations leading to discriminatory outcomes. A few works have been proposed to mitigate the bias in graph representations. However, most existing works require exceptional time and computing resources for training and fine-tuning. To this end, we study the problem of efficient fair graph representation learning and propose a novel framework FairMILE. FairMILE is a multi-level paradigm that can efficiently learn graph representations while enforcing fairness and preserving utility. It can work in conjunction with any unsupervised embedding approach and accommodate various fairness constraints. Extensive experiments across different downstream tasks demonstrate that FairMILE significantly outperforms state-of-the-art baselines in terms of running time while achieving a superior trade-off between fairness and utility.
更多查看译文
关键词
Fairness,Machine Learning,Graph Representation Learning
AI 理解论文
溯源树
样例
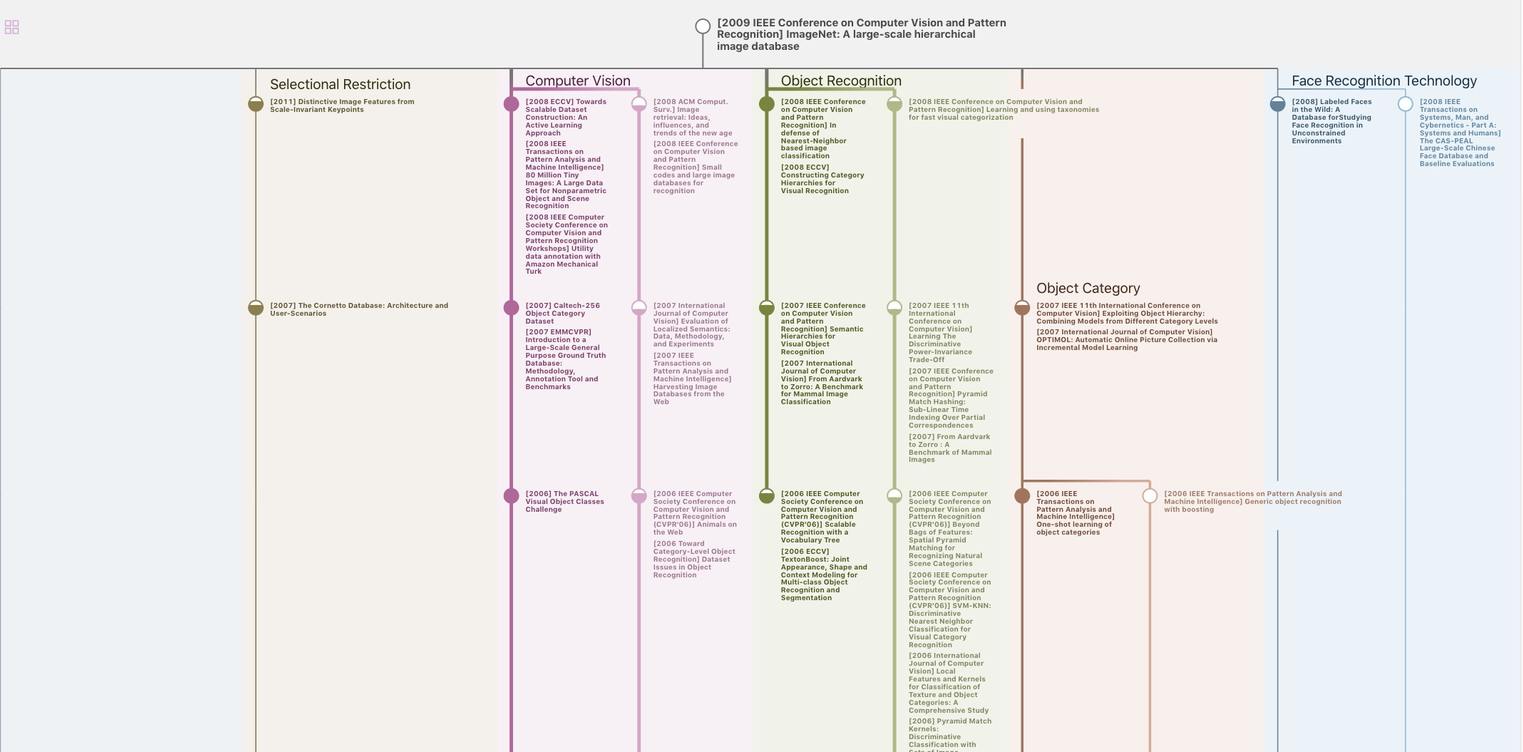
生成溯源树,研究论文发展脉络
Chat Paper
正在生成论文摘要