Meta-learning for Estimating Multiple Treatment Effects with Imbalance.
WISE(2023)
摘要
Ascertaining counterfactual questions, for instance, “Would individuals with diabetes have exhibited better if they had opted for a different medication?”, is a frequent pursuit in research. Observational studies have become increasingly significant in addressing such queries due to their extensive availability and ease of acquisition relative to Randomized Control Trials (RCTs). Recently, approaches based on representation learning and observational data have gained attention for counterfactual inference. Nevertheless, current techniques mainly concentrate on binary treatments and do not tackle the problem of imbalance, whereby data examples in specific treatment groups are relatively limited owing to inherent user preference. In this paper, we present a pioneering model-agnostic framework for counterfactual inference, namely, MetaITE, which stands for Meta-learning for Estimating Individual Treatment Effects, that resolves these research gaps. Furthermore, we introduce two complementary modules that are closely related to the problem set. The first module employs supervisory signals from multiple source treatments, while the second aligns latent distributions among treatment groups to minimize discrepancies. We assess the inference accuracy and generalization ability of MetaITE on two real-world datasets and illustrate that it surpasses state-of-the-art methods.
更多查看译文
关键词
multiple treatment effects,imbalance,meta-learning
AI 理解论文
溯源树
样例
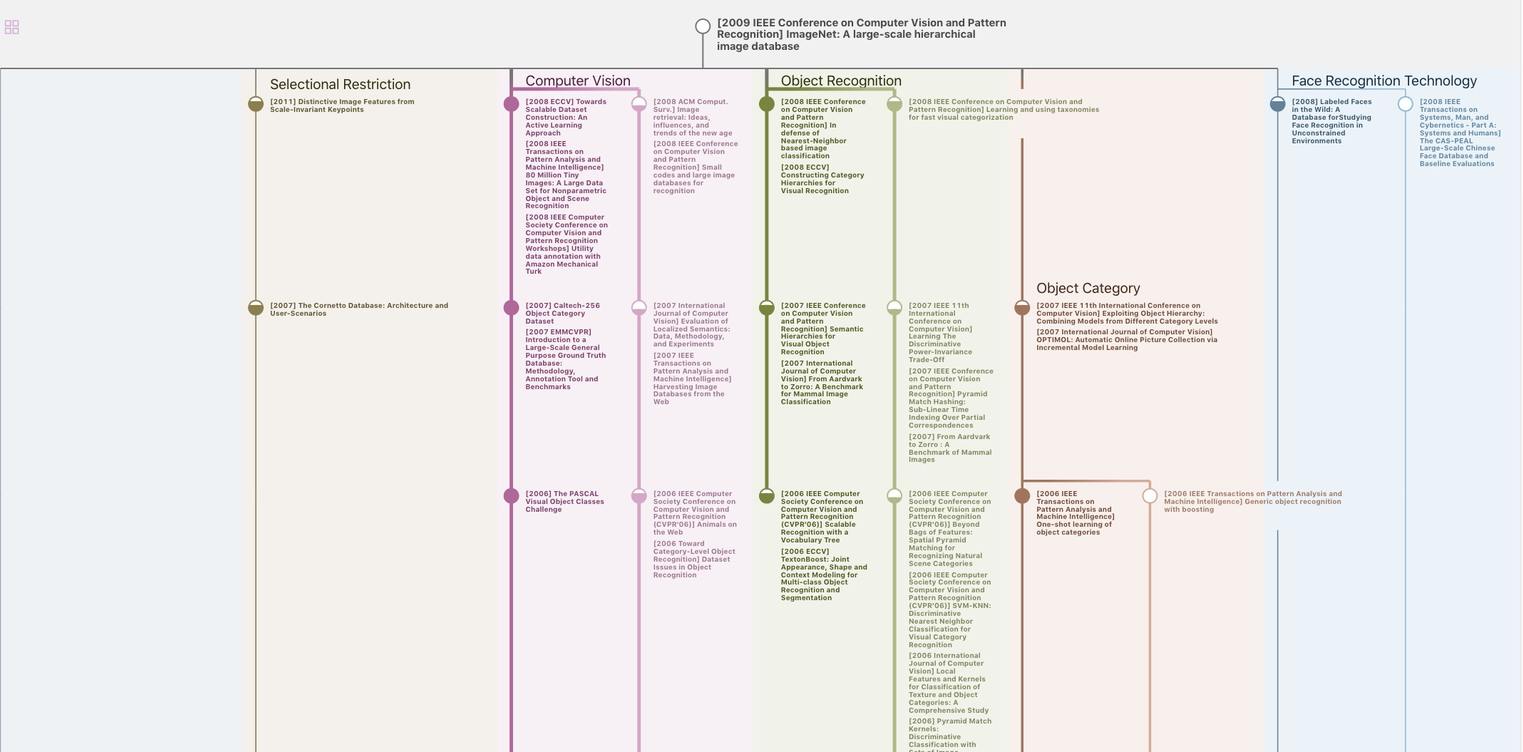
生成溯源树,研究论文发展脉络
Chat Paper
正在生成论文摘要