Bilateral Insider Threat Detection: Harnessing Standalone and Sequential Activities with Recurrent Neural Networks.
WISE(2023)
摘要
Insider threats involving authorised individuals exploiting their access privileges within an organisation can yield substantial damage compared to external threats. Conventional detection approaches analyse user behaviours from logs, using binary classifiers to distinguish between malicious and non-malicious users. However, existing methods focus solely on standalone or sequential activities. To enhance the detection of malicious insiders, we propose a novel approach: bilateral insider threat detection combining RNNs to incorporate standalone and sequential activities. Initially, we extract behavioural traits from log files representing standalone activities. Subsequently, RNN models capture features of sequential activities. Concatenating these features, we employ binary classification to detect insider threats effectively. Experiments on the CERT 4.2 dataset showcase the approach’s superiority, significantly enhancing insider threat detection using features from both standalone and sequential activities.
更多查看译文
关键词
recurrent neural networks,threat,sequential activities,neural networks
AI 理解论文
溯源树
样例
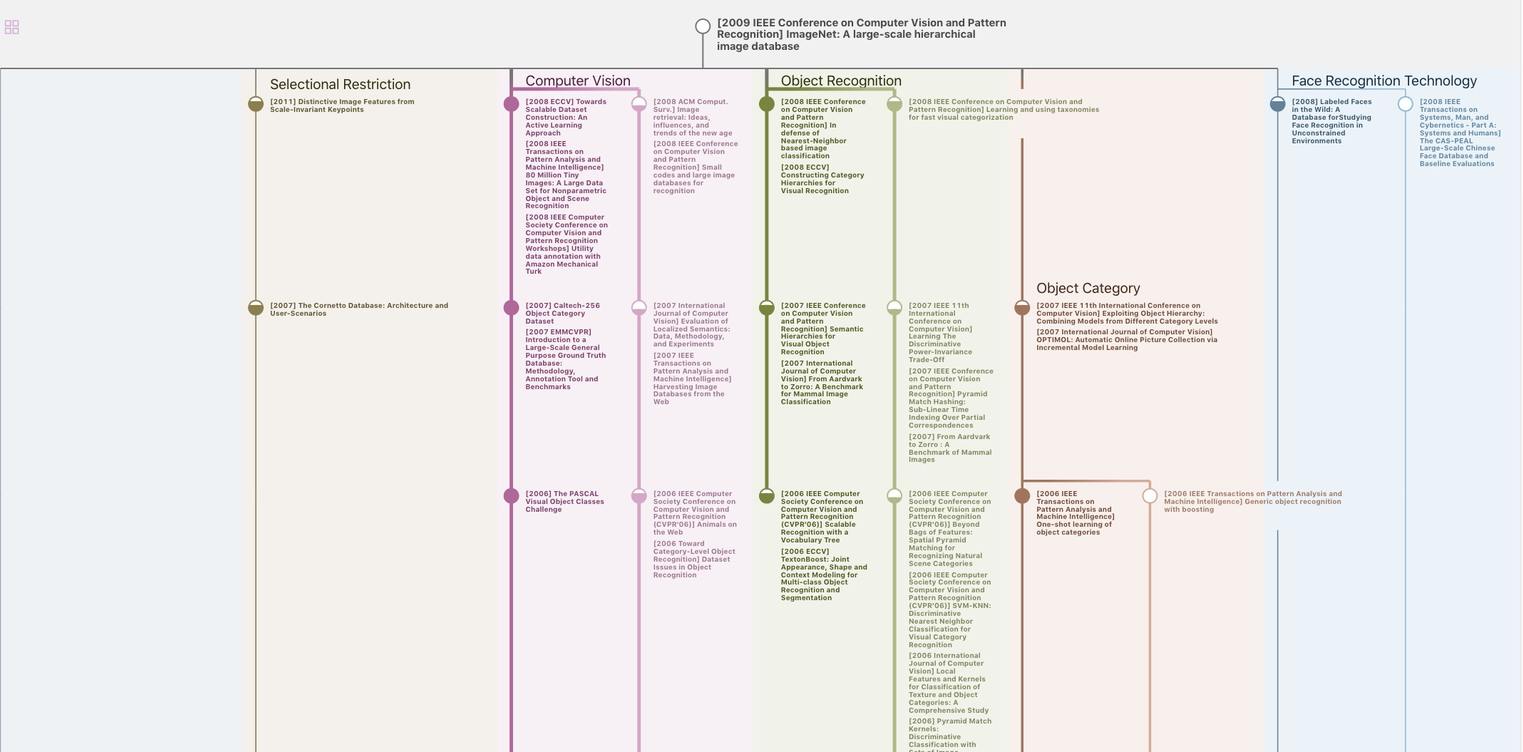
生成溯源树,研究论文发展脉络
Chat Paper
正在生成论文摘要