What Makes a Charge? Identifying Charge-Discriminative Facts with Legal Elements.
NLPCC (1)(2023)
摘要
Over the past few years, there has been a significant surge in the use of deep learning models for automated charge identification tasks, yielding impressive results. However, these models still face several limitations when it comes to explaining the rationale behind charge predictions. Recent research has sought to enhance interpretability by extracting discriminative features from factual descriptions. Nevertheless, such studies often neglect a thorough examination of the corresponding legal provisions. To tackle this issue, the objective of this paper is to establish a fine-grained connection between charges and factual components, specifically identifying charge-discriminative facts that encompass essential legal elements. Several challenges need to be addressed, including the presence of noisy sentences, imbalanced data, and the need for distinct legal elements corresponding to different charges. To tackle these challenges, this paper reframes the task as a few-shot text classification problem and introduces a meta-learning framework that integrates legal element information through a prototypical network. The experimental results demonstrate the effectiveness of this approach in improving charge prediction accuracy and establishing meaningful associations between charges and factual evidences.
更多查看译文
AI 理解论文
溯源树
样例
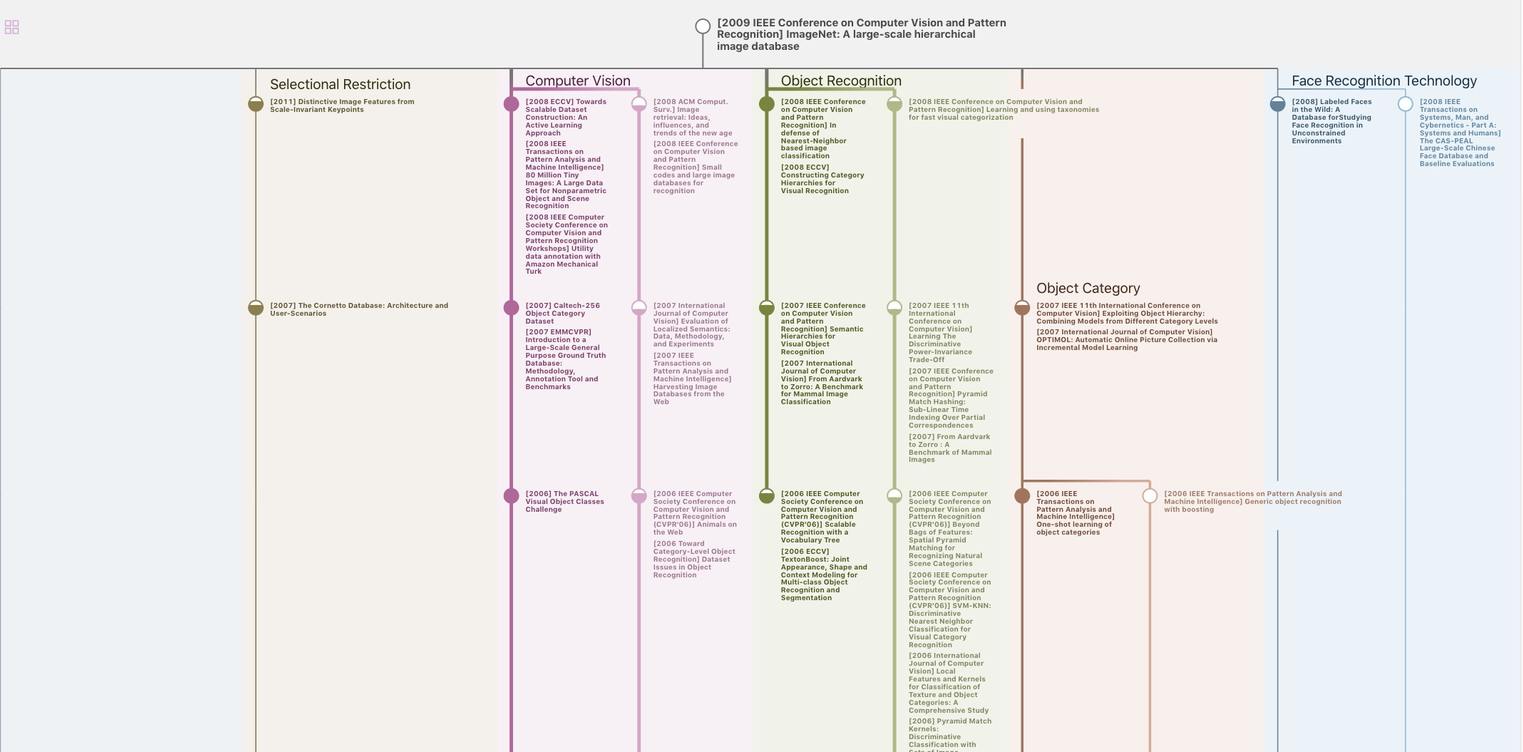
生成溯源树,研究论文发展脉络
Chat Paper
正在生成论文摘要