Enhancing Semantic Consistency in Linguistic Steganography via Denosing Auto-Encoder and Semantic-Constrained Huffman Coding.
NLPCC (2)(2023)
Abstract
Linguistic steganography is a useful technique to hide secret messages within a normal cover text, playing a crucial role in the field of data protection. Compared to the data encryption techniques, steganography can make the security data transmission process more imperceptible because the outputted stego (steganography) texts are not garbled codes but look like normal texts. Consequently, the essential of linguistic steganography is to improve the imperceptibility of the outputted stego texts. Although prior works can already generate fluent stego texts, how to ensure the semantics of stego text be natural and reasonable in human cognition is still a challenging problem. To alleviate this issue, this work proposes a novel Semantic-Preserved Linguistic Steganography Auto-Encoder (SPLS-AutoEncoder) to improve imperceptibility by enhancing semantic consistency. SPLS-AutoEncoder first minimizes the possible distortion when embedding the secret message into the cover text by using the denoising auto-encoder BART as the backbone model. Then, we propose a novel Dynamic Semantic-Constrained Huffman Coding, which uses a dynamic context information embedding and a global topic embedding to ensure semantic consistency between the cover text and stego text. Experimental results on two Chinese datasets show that our method has excellent performance compared with the previous methods. The datasets and code are released at https://github.com/Y-NLP/LinguisticSteganography/tree/main/NLPCC2023_SPLS-AutoEncoder .
MoreTranslated text
Key words
linguistic steganography,semantic consistency,auto-encoder,semantic-constrained
AI Read Science
Must-Reading Tree
Example
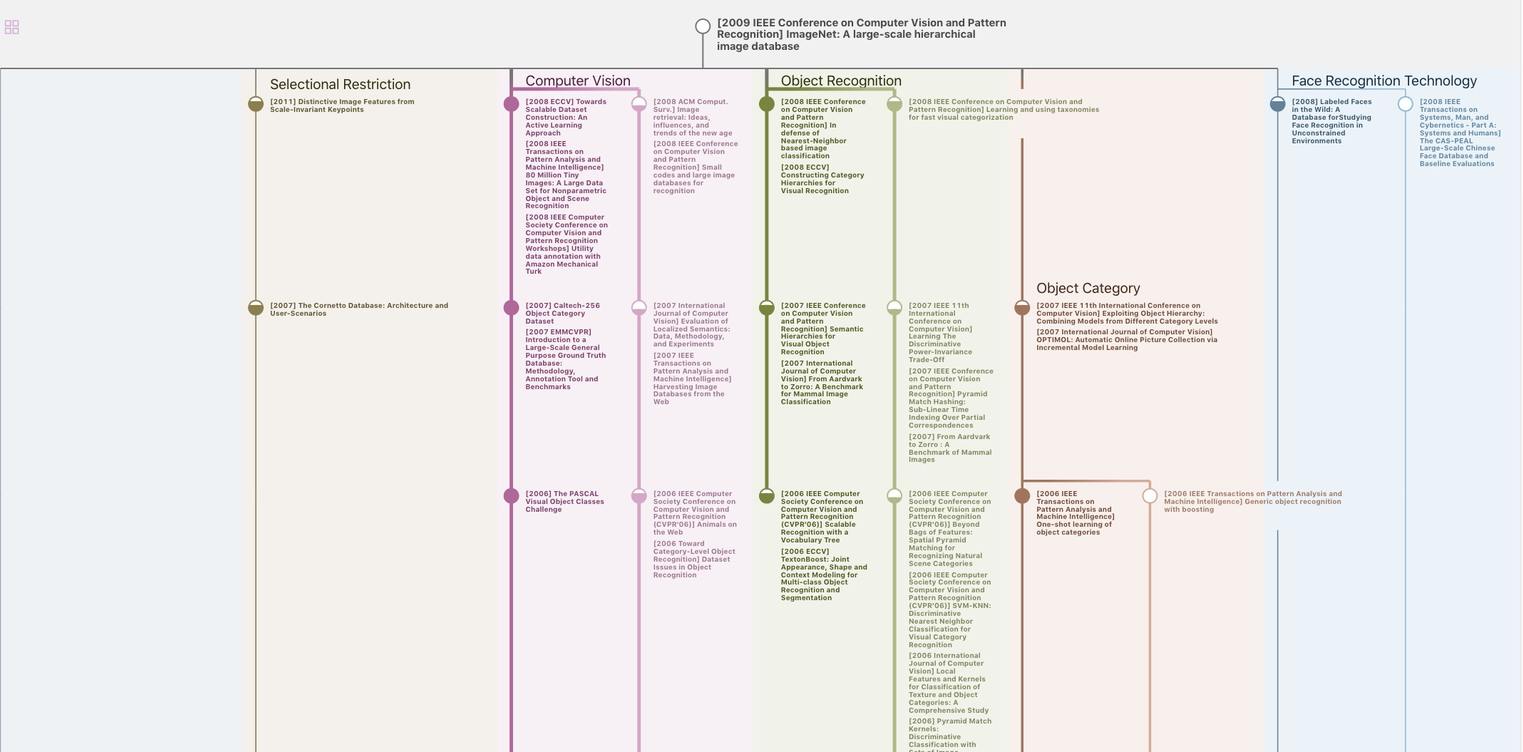
Generate MRT to find the research sequence of this paper
Chat Paper
Summary is being generated by the instructions you defined