Causal MRC: Mitigating Position Bias Based on Causal Graph
DATABASE SYSTEMS FOR ADVANCED APPLICATIONS. DASFAA 2023 INTERNATIONAL WORKSHOPS, BDMS 2023, BDQM 2023, GDMA 2023, BUNDLERS 2023(2023)
摘要
Extractive Machine Reading Comprehension (MRC) requires models to obtain start and end positions of answers from a given passage. MRCmodelsmay tend to rely on position bias as a shortcut, and thus they fail to learn the multi-source knowledge from both passages and questions sufficiently. Recent debiasing methods proposed to exclude the position prior during inference. However, they cannot distinguish the good position context and bad position bias from the whole prior. In this paper, we propose a novel MRC framework CausalMRC based on causal graph to mitigate position bias. Motivated by causal inference, we design a causal graph for MRC to formulate the position bias as the direct causal effect of passages on answers. Specifically, wemitigate the position bias by subtracting the direct position effect from the total causal effect. Experiments demonstrate that our proposed CausalMRC achieves competitive performance on the biased SQuAD dataset while performing robustly on the original SQuAD.
更多查看译文
关键词
Machine reading comprehension,Causal graph,Position bias
AI 理解论文
溯源树
样例
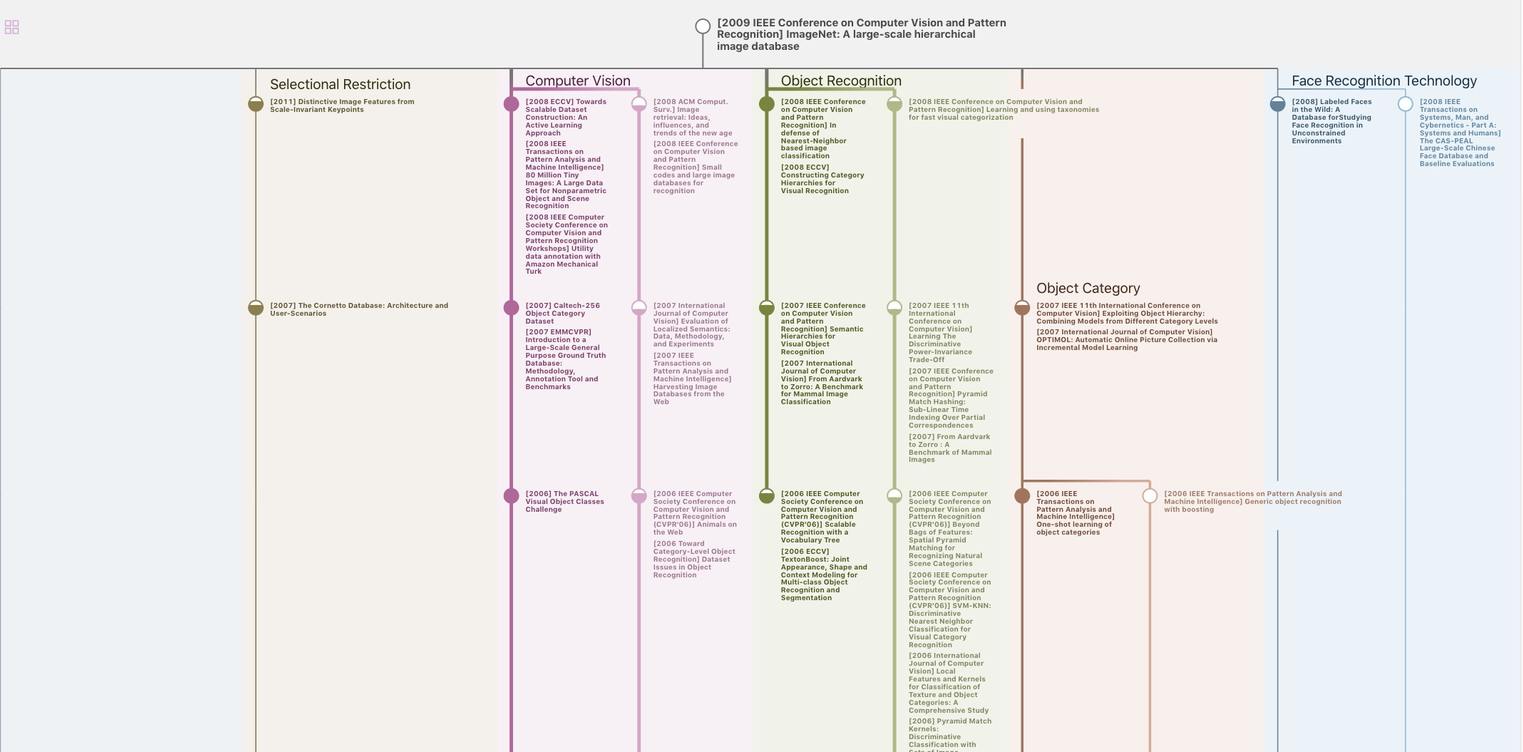
生成溯源树,研究论文发展脉络
Chat Paper
正在生成论文摘要