Two-Stage Adaptation for Cross-Corpus Multimodal Emotion Recognition.
NLPCC (2)(2023)
Abstract
The development of multimodal emotion recognition is severely limited by time-consuming annotation costs. In this paper, we pay attention to the multimodal emotion recognition task in the cross-corpus setting, which can help adapt a trained model to an unlabeled target corpus. Inspired by the recent development of pre-trained models, we adopt a multimodal emotion pre-trained model to provide a better representation learning foundation for our task. However, we may face two domain gaps when applying a pre-trained model to the cross-corpus downstream task: the scenario gap between pre-trained and downstream corpora, and the distribution gap between different downstream sets. To bridge these two gaps, we propose a two-stage adaptation method. Specifically, we first adapt a pre-trained model to the task-related scenario through task-adaptive pre-training. We then fine-tune the model with a cluster-based loss to align the distribution of two downstream sets in a class-conditional manner. Additionally, we propose a ranking-based pseudo-label filtering strategy to obtain more balanced and high-quality samples from the target sets for calculating the cluster-based loss. We conduct extensive experiments on two emotion datasets, IEMOCAP and MSP-IMPROV. The results of our experiments demonstrate the effectiveness of our proposed two-stage adaptation method and the pseudo-label filtering strategy in cross-corpus settings.
MoreTranslated text
Key words
multimodal,adaptation,two-stage,cross-corpus
AI Read Science
Must-Reading Tree
Example
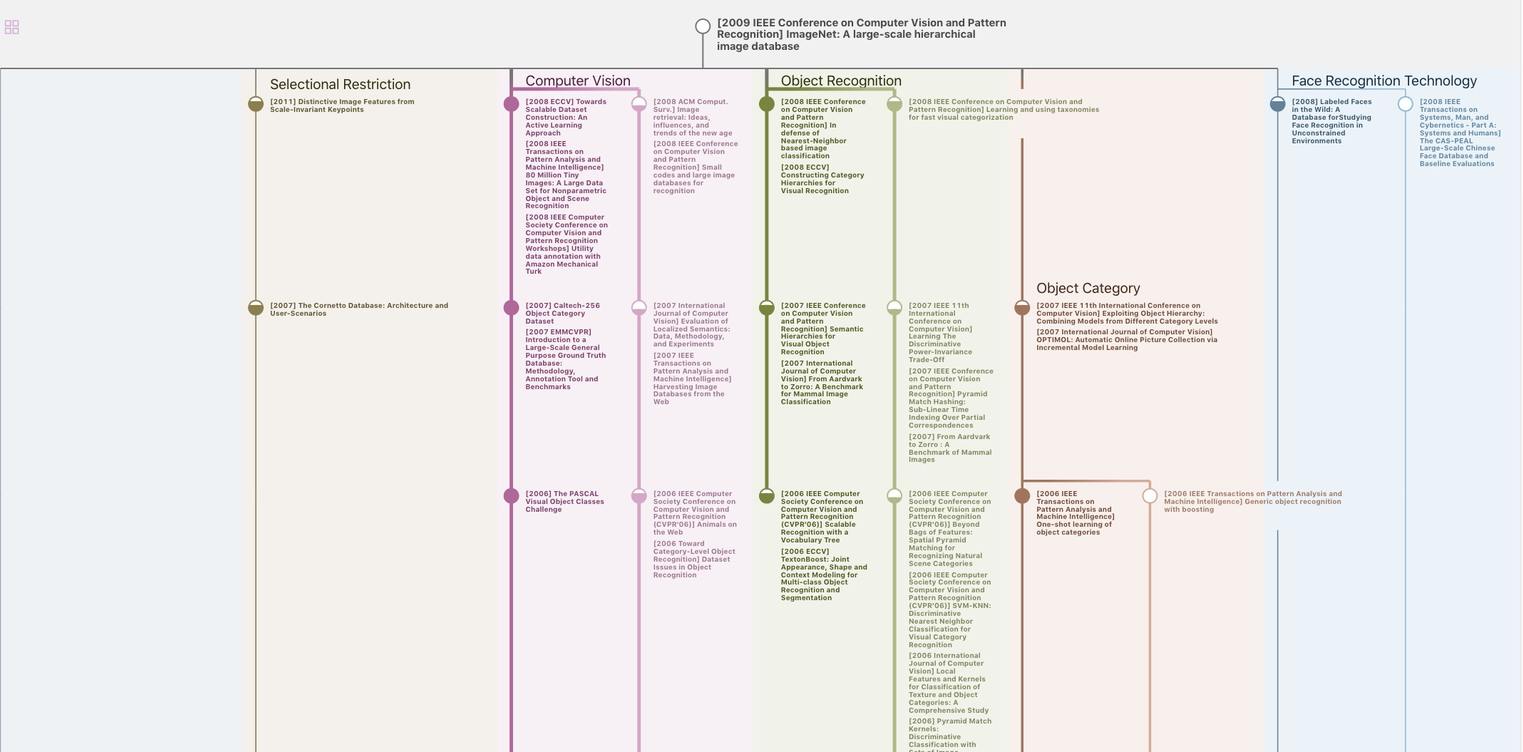
Generate MRT to find the research sequence of this paper
Chat Paper
Summary is being generated by the instructions you defined