Label-Guided Compressed Prototypical Network for Incremental Few-Shot Text Classification.
NLPCC (1)(2023)
摘要
Incremental few-shot text classification (IFSTC) involves the sequential classification of new classes with limited instances while preserving the discriminability of old classes, which can effectively support downstream tasks such as information extraction and knowledge graph construction. The primary objective of IFSTC is to achieve a balance between plasticity and stability , where classification models should be plastic enough to learn patterns from new classes, while also being stable enough to retain knowledge learned from previously seen classes. In previous work of incremental few-shot learning, the popular approach is to compress the base classes space to enhance model plasticity . However, the application of current space compression methods to text classification presents challenges due to the difficulty in simulating latent data through text data synthesis. Moreover, freezing all model parameters to maintain model stability is not a viable solution as it fails to incorporate new knowledge. To solve the problems above, we propose Label-guided Compressed Prototypical Network (LGCPN) for IFSTC, which consists of two parts. Firstly, label-guided space compression is proposed to improve model plasticity with text data through leveraging the information carried by labels to assist in the space compression. Secondly, few-params tuning is designed to maintain model stability and enable it to learn new knowledge by selectively fine-tuning few parameters. We experimented on two public datasets to evaluate the performance of our proposed method for IFSTC. The experimental results demonstrate that our method can significantly improve the accuracy of each round in the incremental stage compared to two baselines.
更多查看译文
关键词
compressed prototypical network,classification,text,label-guided,few-shot
AI 理解论文
溯源树
样例
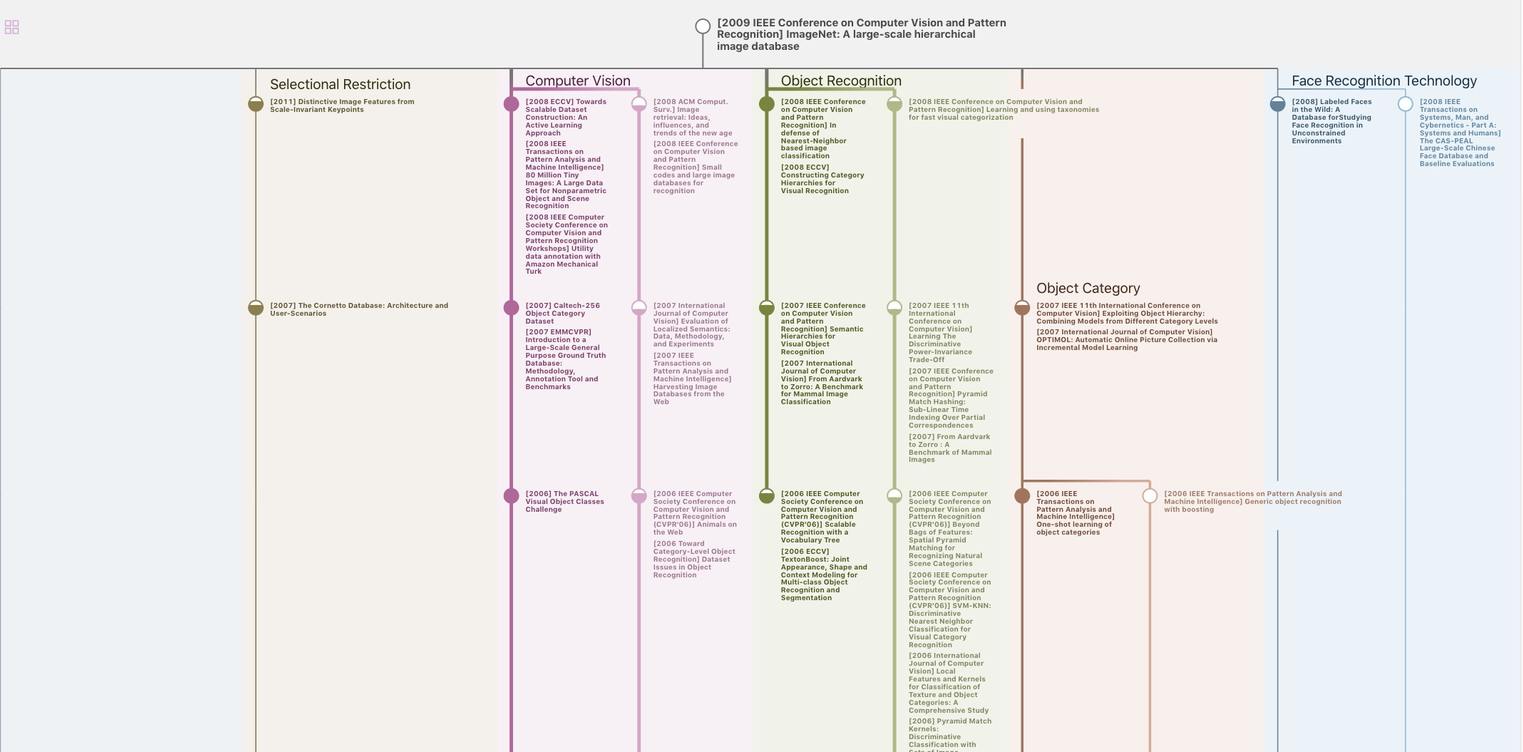
生成溯源树,研究论文发展脉络
Chat Paper
正在生成论文摘要