The Application of Hybrid Dynamic Recurrent Fuzzy Neural Network in Lower Limb Rehabilitation Function Evaluation.
ICIRA (2)(2023)
摘要
To enhance the human-robot interaction ability of a lower-limb rehabilitation robot for stroke patients, it is crucial to accurately quantify the rehabilitation status. This paper proposes an adaptive rehabilitation assessment approach based on a Takagi-Sugeno (T-S) fuzzy neural network aided by a multi-signal acquisition platform. By extracting five kinematic data and electromyographic (EMG) data of clinical rehabilitation training, the method obtains the lower limb characteristic parameters of patients to simulate the rehabilitation evaluation process. The multi-factor line regression method evaluates the impact of maximum joint activity, free acceleration, angular acceleration, average velocity, jerk, and EMG signal data on the evaluation results. The mapping between rehabilitation feature metrics and recovery conditions is initially estimated using a T-S fuzzy neural network. The paper then proposes a hybrid optimization learning method for the above network, which includes particle swarm optimization (PSO) and recursive least squares estimator (RLSE). PSO modifies the function parameters used to calculate the membership degree to optimize the fitness of fuzzy rules. However, the PSO algorithm can easily trap into local optima, so the freedom coefficient that calculates the consequent of fuzzy rules is further corrected based on RLSE. The iterative learning of the T-S fuzzy neural network is completed using gradient descent. Finally, the proposed approach is compared for convergence efficiency with two intelligent algorithms, and the results demonstrate the proposed network’s excellent convergence performance and high model accuracy (RMSE = 2.336 × 10 - 3 ), which is essential for lower-limb rehabilitation.
更多查看译文
关键词
rehabilitation,lower limb,neural network
AI 理解论文
溯源树
样例
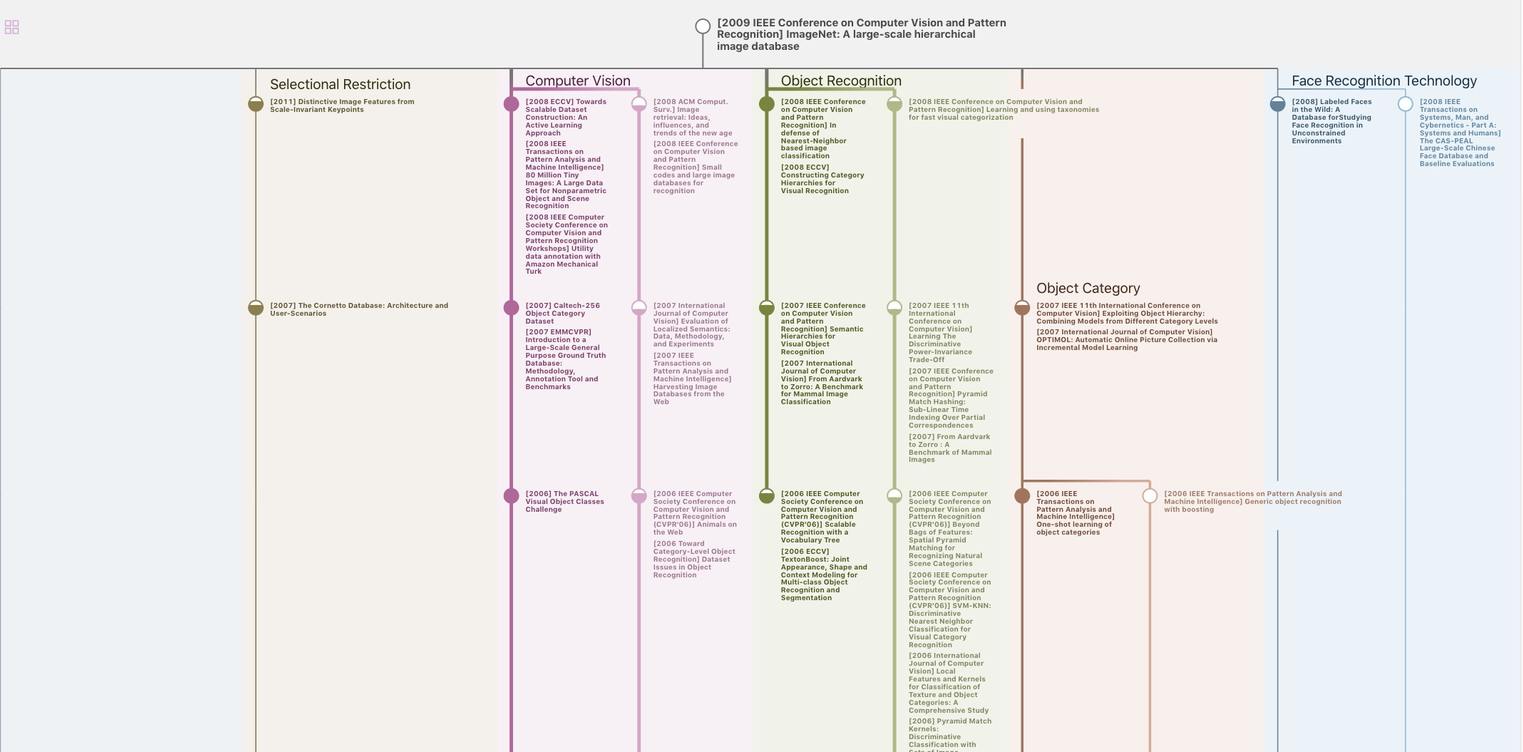
生成溯源树,研究论文发展脉络
Chat Paper
正在生成论文摘要