Accelerated Unfolding Network for Medical Image Reconstruction with Efficient Information Flow.
ICIRA (3)(2023)
摘要
Deep unfolding networks (DUNs) have become mainstream for many medical image reconstruction tasks due to their exceptional interpretability and high performance. Unlike black-box deep neural networks, DUNs can provide insight into the intermediary steps of the reconstruction process. Extensive work has been done to study classical optimization algorithms. However, there are several issues that still require further exploration, including the unfolding implications of accelerated optimization algorithms and the performance bottlenecks in unfolding algorithms. To tackle these two concerns, this paper initially validates the extent of performance enhancement achieved by accelerated variation of ADMM, as compared to the original method. As for the second issue, we point out that the coarse information fusion operations utilized in existing unfolding networks primarily impede their performance (e.g., simple addition and subtraction). Based on this, we design a simple, reasonable yet effective accelerated ADMM-based unfolding framework, which integrate multi-channel information into existing DUNs. Additionally, the developed efficient feature aggregation strategy can further enhance the performance of DUNs. We demonstrate, through MRI accelerated reconstruction experiments, that the proposed framework outperforms state-of-the-art DUNs while utilizing fewer parameters.
更多查看译文
关键词
medical image reconstruction,flow
AI 理解论文
溯源树
样例
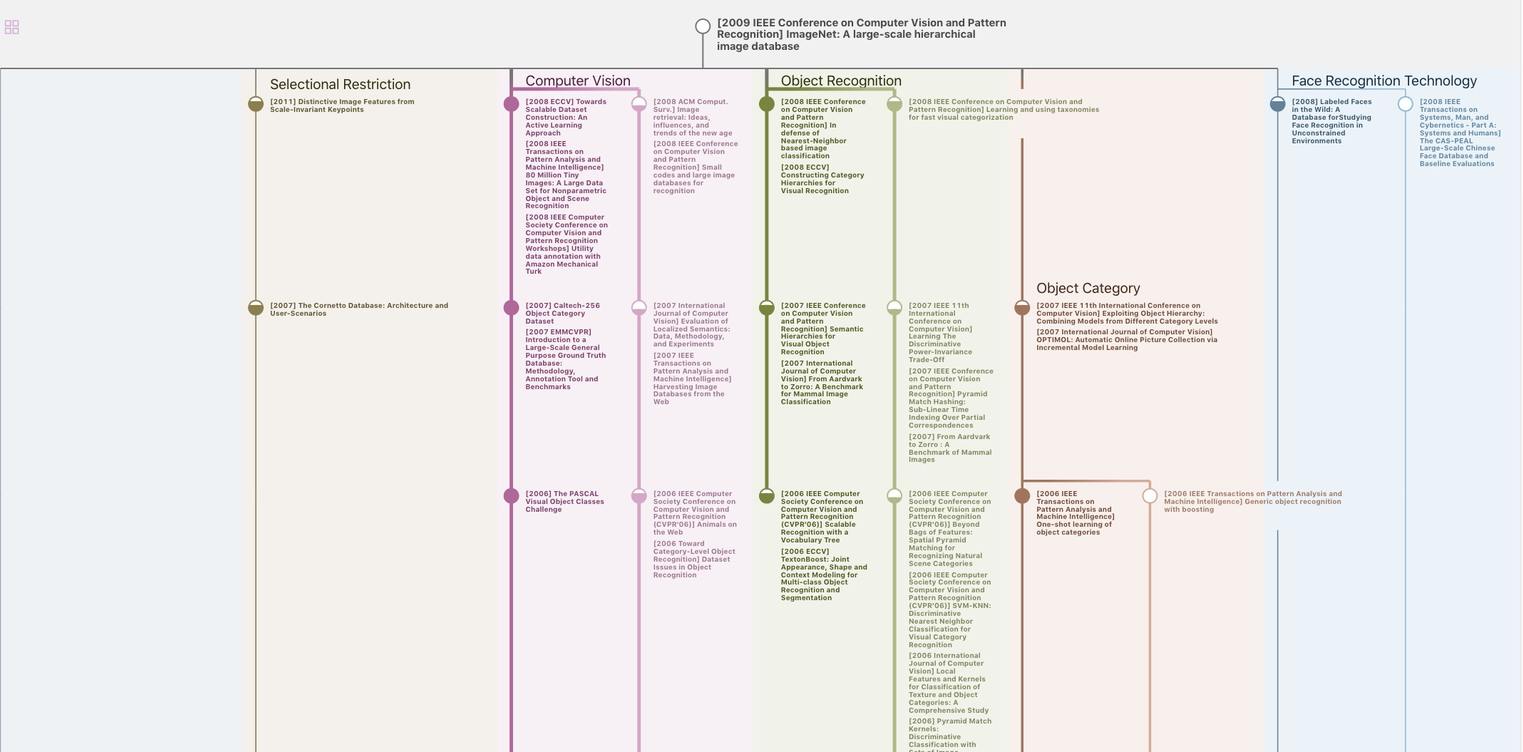
生成溯源树,研究论文发展脉络
Chat Paper
正在生成论文摘要