Outlier Detection and Correction for Time Series Data of Tunnel Boring Machine.
ICIRA (6)(2023)
摘要
Time series data mining has attracted ever-growing interest in the past decades thanks to its appealing significance in analyzing the operational statuses of tunnel boring machine (TBM) and extracting valuable information from the collected signals. The quality of raw data is mainly affected by outliers and pre-processing includes the task of detecting and correcting these outliers. In this study, a hierarchical sliding window-based method for handling outliers is proposed. First, the sliding window technique is used to divide the initial time series into several sub-time series. Subsequently, the radius of the confidence interval for the slope of each sub-time series is efficiently calculated to identify any outlier sub-time series. The local outlier factor algorithm is employed to further ascertain the outliers. Finally, for the detected outliers, the k-nearest neighbor algorithm is used to correct the outliers. The experimental results validate the practicality of the proposed method for engineering applications.
更多查看译文
关键词
tunnel boring machine,time series data
AI 理解论文
溯源树
样例
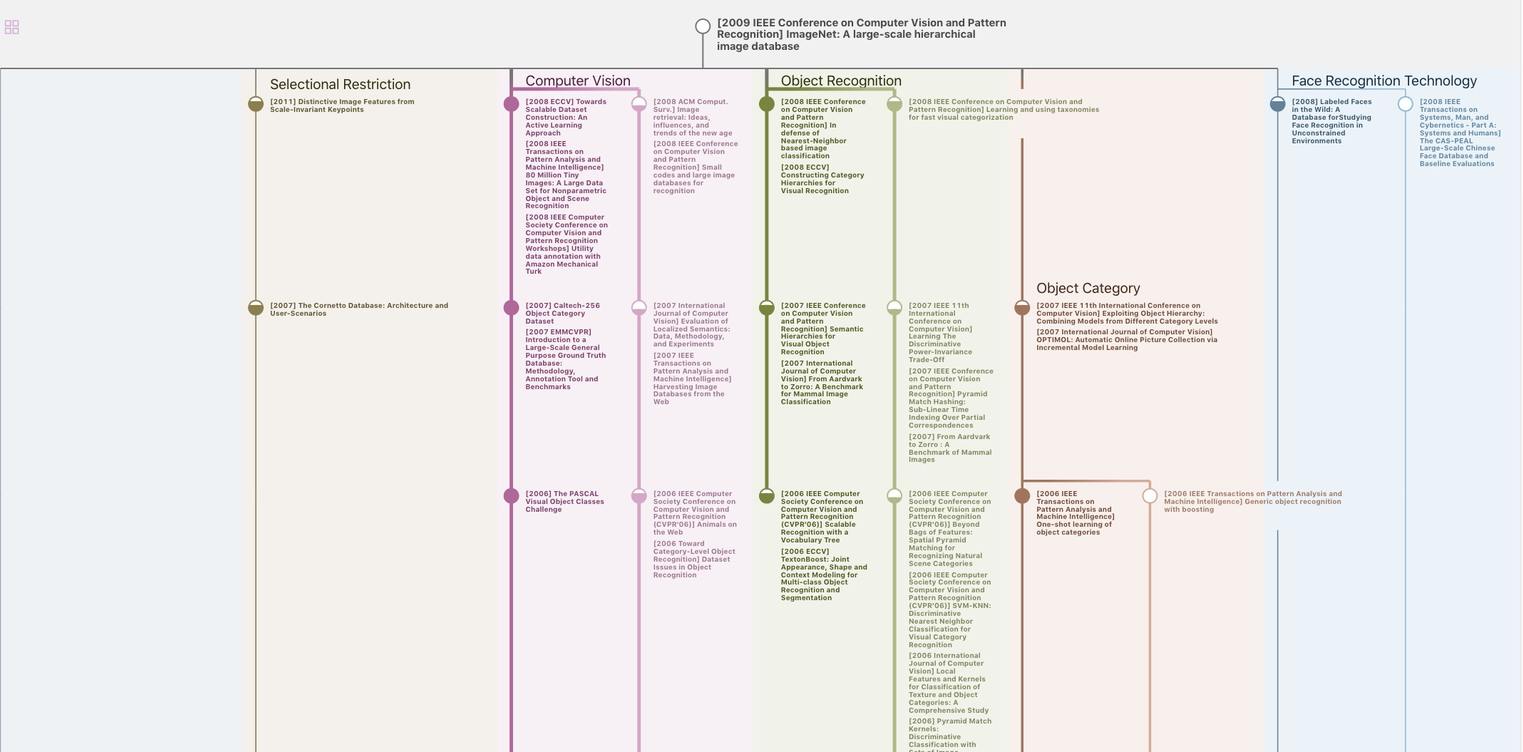
生成溯源树,研究论文发展脉络
Chat Paper
正在生成论文摘要