A Novel Skill Learning Framework for Redundant Manipulators Based on Multi-task Dynamic Movement Primitives.
ICIRA (2)(2023)
Abstract
Skill learning is a frontier problem in intelligent robot systems, which aims to make robots efficiently learn manipulation skills from human demonstration. In this paper, we propose a novel robot skill learning framework based on multi-task dynamic movement primitives (MT-DMPs) to improve the operation efficiency of redundant manipulators, which is mainly composed of sub-task segmentation module, parameter setting module, robot skill learning module and pose optimization module. We describe the design steps of the proposed framework in detail as follows: 1) Finite State Machine (FSM) is used to divide multiple tasks into a sub-task sequence, thus forming the state transition diagram for the robot to perform multiple tasks. 2) An exponential decay function is introduced to improve the basic DMPs, and the design flow for instantiating robot skills is summarized. 3) Velocity Directional Manipulability (VDM) is introduced as the evaluation index of robot motion performance, and a pose optimization model suitable for redundant manipulators is established. Finally, we build an experimental platform based on the Robot Operating System (ROS), and carry out a series of experiments. The results show that our proposed skill learning framework can improve the efficiency and accuracy of autonomous operation of redundant manipulators.
MoreTranslated text
Key words
redundant manipulators,novel skill learning framework,multi-task
AI Read Science
Must-Reading Tree
Example
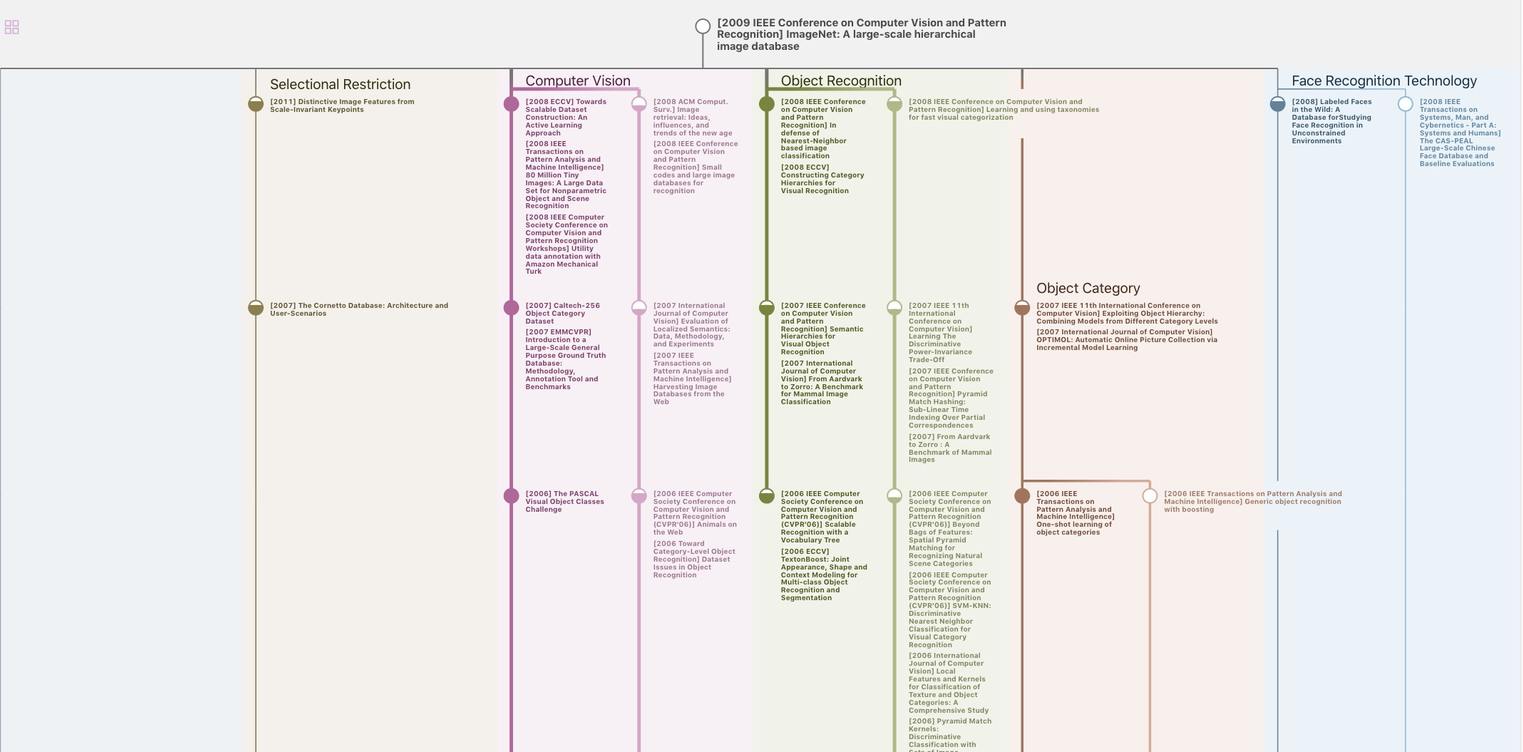
Generate MRT to find the research sequence of this paper
Chat Paper
Summary is being generated by the instructions you defined