Boosting NSGA-II-Based Wrappers Speedup for High-Dimensional Data: Application to EEG Classification
ADVANCES IN COMPUTATIONAL INTELLIGENCE, IWANN 2023, PT I(2023)
摘要
The considerable technological evolution during the last deca-des has made it possible to deal with biological datasets of increasing higher dimensionality, such as those used in BCI applications. Thus, techniques such as feature selection, which allow obtaining the underlying information of these datasets by removing those features considered redundant or noisy, have emerged. Over the years, wrapper approaches based on evolutionary algorithms have gained great relevance, as they have proven to be one of the best procedures to tackle this problem, with NSGA-II being one of the most used search strategies. Historically, these procedures have presented a well-known bottleneck in the evaluation method. However, a more significant bottleneck appears when dealing with high-dimensional datasets, which lies in the application of the NSGA-II's selection method to very large populations. For this reason, this paper aims to alleviate this problem and, consequently, develop a parallel strategy able to reach a superlinear speedup.
更多查看译文
关键词
High-dimensionality,Feature Selection,EEG Classification,Wrapper,Bottleneck,NSGA-II,Multi-population
AI 理解论文
溯源树
样例
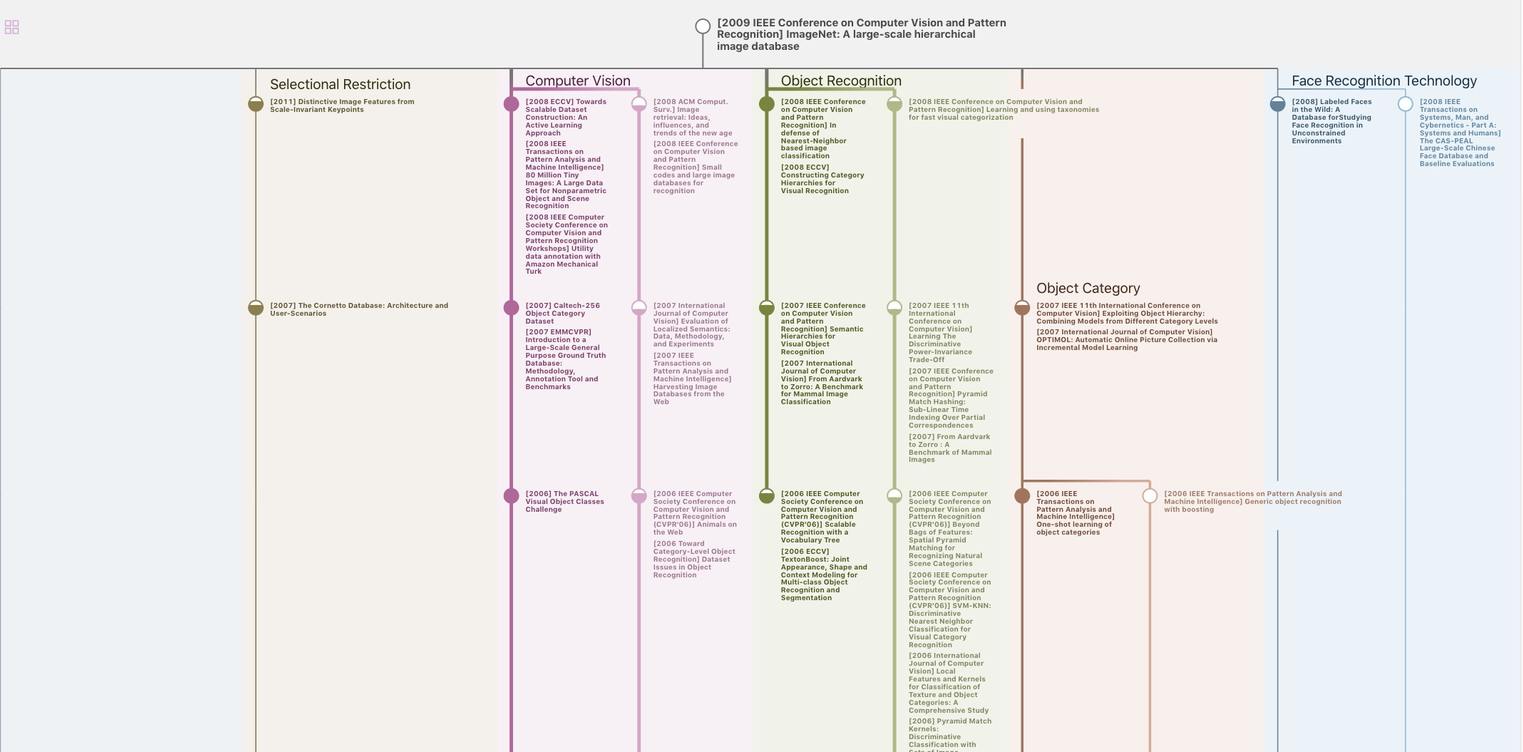
生成溯源树,研究论文发展脉络
Chat Paper
正在生成论文摘要