Fast Convolutional Analysis of Task-Based fMRI Data for ADHD Detection
ADVANCES IN COMPUTATIONAL INTELLIGENCE, IWANN 2023, PT II(2023)
摘要
Among the most common neurodevelopmental disorders, Attention Deficit Hyperactivity Disorder (ADHD) is a complex and challenging one to identify. This happens because there are no objective medical techniques, and diagnoses are made only on interviews and a set of symptoms tests evaluated by psychiatrists. However, in recent years, the use of Deep Learning techniques has emerged as a promising solution for accurately classifying ADHD, using non-intrusive advanced imaging techniques such as fMRI data. Specifically, task-based fMRI data can be especially valuable for identifying ADHD, as it enables researchers to examine the functional activity of the brain during tasks that involve working memory, which is known to be affected in individuals with this disorder. The presented paper introduces 3D-ADHD, a 3D Convolutional Neural Network-based approach that uses individual time instant of working memory task-based fMRI data for real-time ADHD classification. The proposed model not only achieves remarkable accuracy, but is also very fast in the inference phase. This makes 3D-ADHD suitable for real-time classification and integration into medical systems, including computer-aided diagnosis one. As a result, this model has great potential for clinical use and can significantly contribute to the diagnosis of ADHD. This work has the potential to advance the accurate and efficient detection of ADHD with real-time diagnoses, opening new opportunities for research on faster detection and early treatment of this dysfunction.
更多查看译文
关键词
ADHD Detection,Deep Learning,Task-based fMRI Data,Fast Detection
AI 理解论文
溯源树
样例
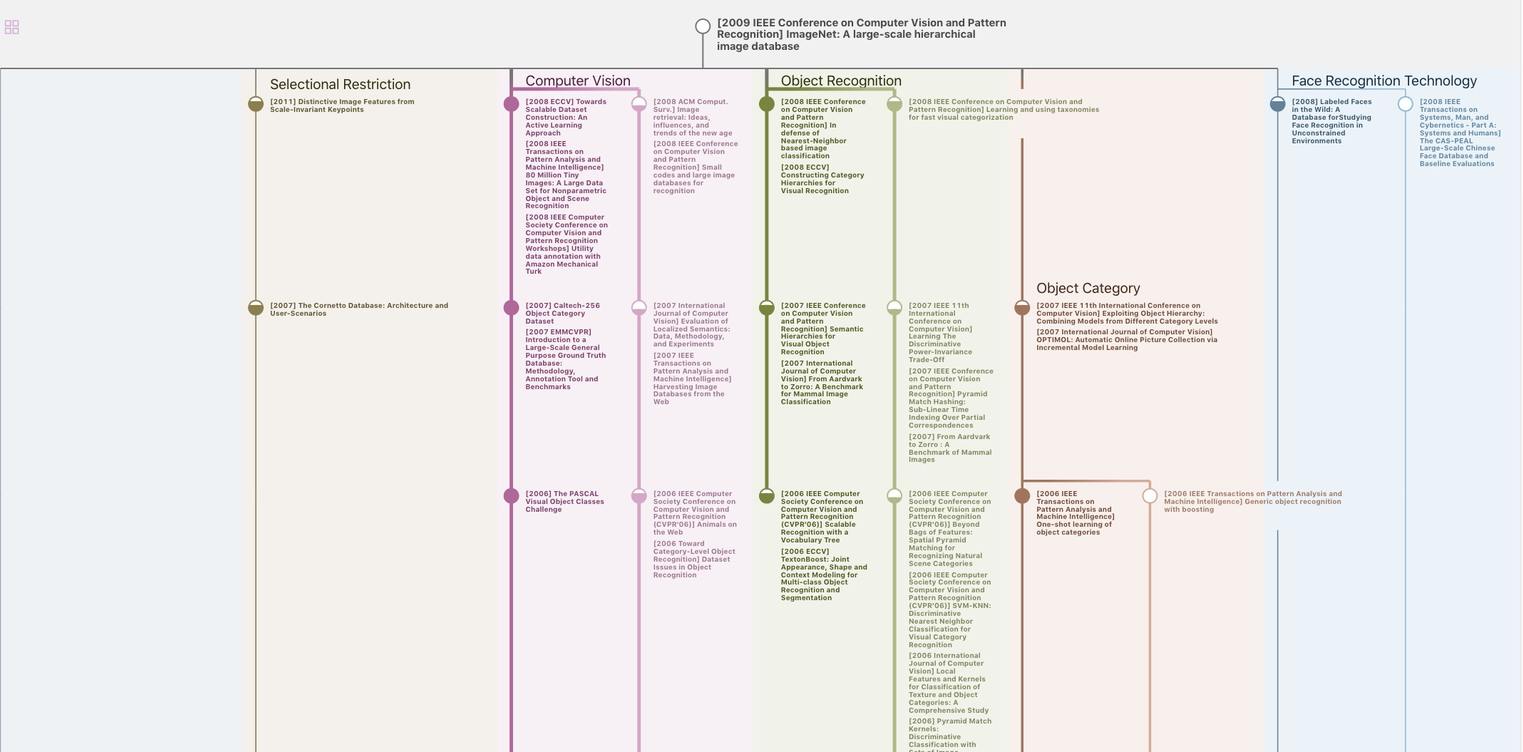
生成溯源树,研究论文发展脉络
Chat Paper
正在生成论文摘要