Fair Empirical Risk Minimization Revised
ADVANCES IN COMPUTATIONAL INTELLIGENCE, IWANN 2023, PT I(2023)
摘要
Artificial Intelligence is nowadays ubiquitous, thanks to a continuous process of commodification, revolutionizing but also impacting society at large. In this paper, we address the problem of algorithmic fairness in Machine Learning: ensuring that sensitive information does not unfairly influence the outcome of a classifier. We extend the Fair Empirical Risk Minimization framework [10] where the fair risk minimizer is estimated via constrained empirical risk minimization. In particular, we first propose a new, more general, notion of fairness which translates into a fairness constraint. Then, we propose a new convex relaxation with stronger consistency properties deriving both risk and fairness bounds. By extending our approach to kernel methods, we will also show that the proposal empirically over-performs the state-of-the-art Fair Empirical Risk Minimization approach on several real-world datasets.
更多查看译文
关键词
Machine Learning,Algorithmic Fairness,In-processing Fairness,Consistency Results,Convex Constrained Optimization,Kernel Methods
AI 理解论文
溯源树
样例
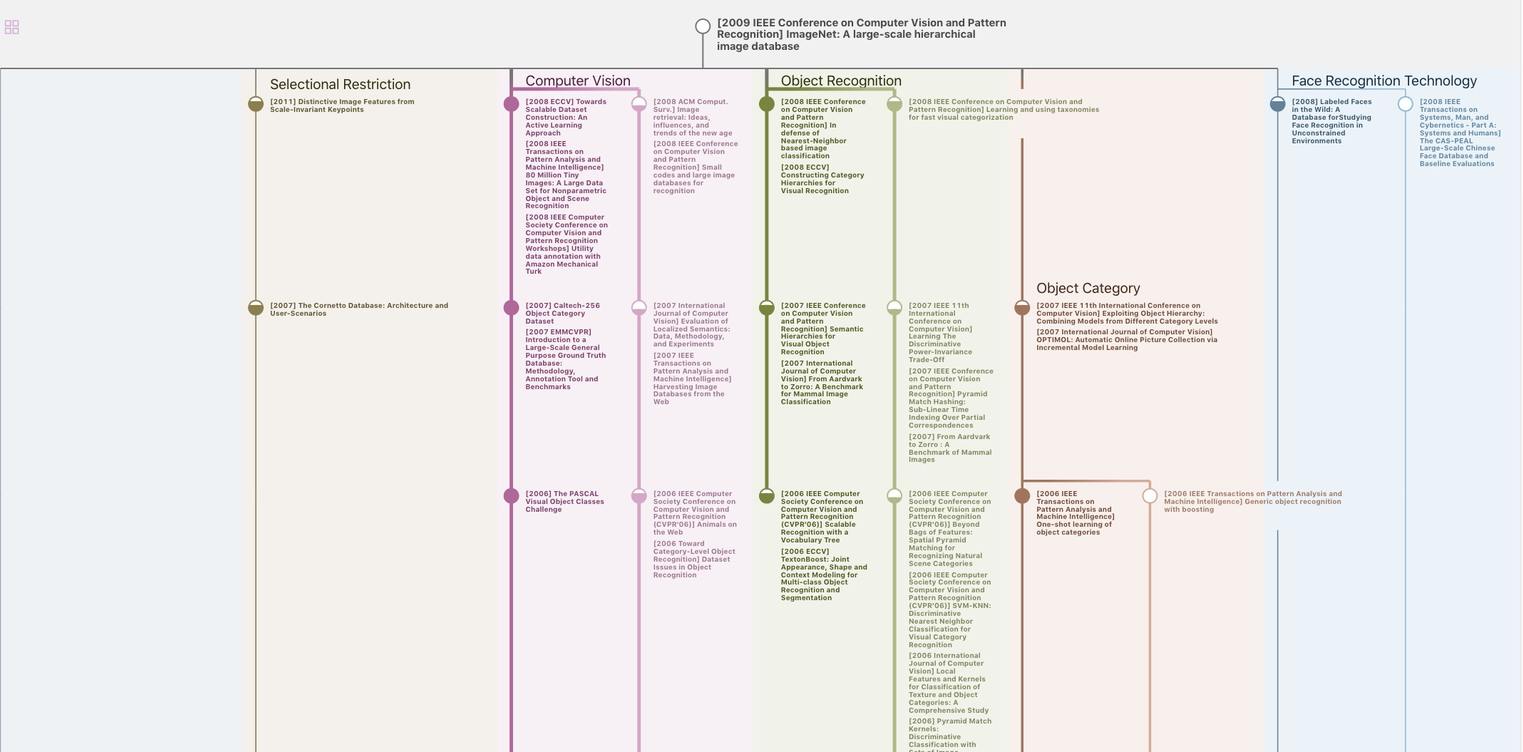
生成溯源树,研究论文发展脉络
Chat Paper
正在生成论文摘要