Incentivizing Mobility of Multi-agent Vehicle Swarms with Deep Reinforcement Learning for Sensing Coverage Optimization.
UbiComp/ISWC Adjunct(2022)
摘要
Mobile Crowdsensing Systems (MCS) often utilize non-dedicated mobile agents (e.g., ridesharing cars) equipped with sensors to collect urban data as they move around a city. Utilizing these non-dedicated agents provides a cheap means for mobile crowdsensing. However, driver agents often focus on traveling to areas where they can pick up more passengers, which can cause the distribution of uploaded data to mismatch our intended distribution. In this work, we propose a passive incentivization scheme in which incentives are applied to different map locations for agents who travel to that location at that time and upload data. We design a simulated environment for multi-agent mobile crowdsensing systems based on real-world taxi data and model large amount of agents with heterogeneous utility functions and routing behaviors, namely myopic, semi-myopic, and farsighted. With this environment, we propose using reinforcement learning to search for an ideal incentivization policy. Finally, we show through experiments that a Proximal Policy Optimization-based method is capable of learning such a policy in spite of the complexities and high dimensionality of the environment and action spaces.
更多查看译文
AI 理解论文
溯源树
样例
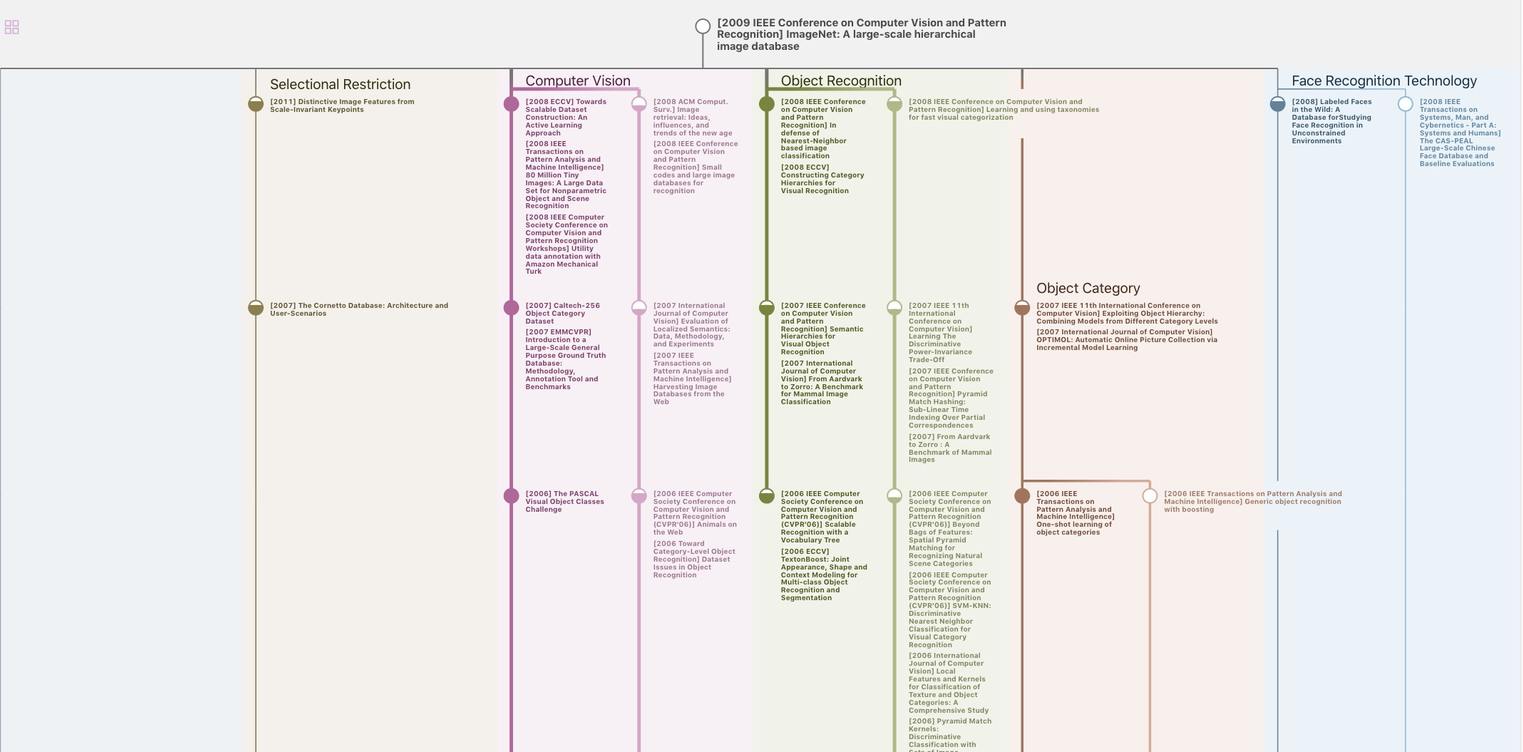
生成溯源树,研究论文发展脉络
Chat Paper
正在生成论文摘要