Estimating the Optimal Training Set Size of Keyword Spotting for Historical Handwritten Document Transcription.
IGS(2023)
Abstract
We address the problem of estimating the tradeoff between the size of the training set and the performance of a KWS when used to assist the transcription of small collections of historical handwritten documents. As this application domain is characterized by a lack of data, and techniques such as transfer learning and data augmentation require more resources than those that are commonly available in the organizations holding the collections, we address the problem of getting the best out of the available data. For this purpose, we reformulate the problem as that of finding the size of the training set leading to a KWS whose performance, when used to support the transcription, allows to obtain the largest reduction of the human efforts to achieve the complete transcription of the collection. The results of a large set of experiments on three publicly available datasets largely adopted as a benchmark for performance evaluation show that a training set made of 5 to 8 pages is enough for achieving the largest reduction, independently of the actual pages included in the training set and the corresponding keyword lists. They also show that the actual time reduction depends much more on the keyword list than on the KWS performance.
MoreTranslated text
Key words
keyword spotting,optimal training set size
AI Read Science
Must-Reading Tree
Example
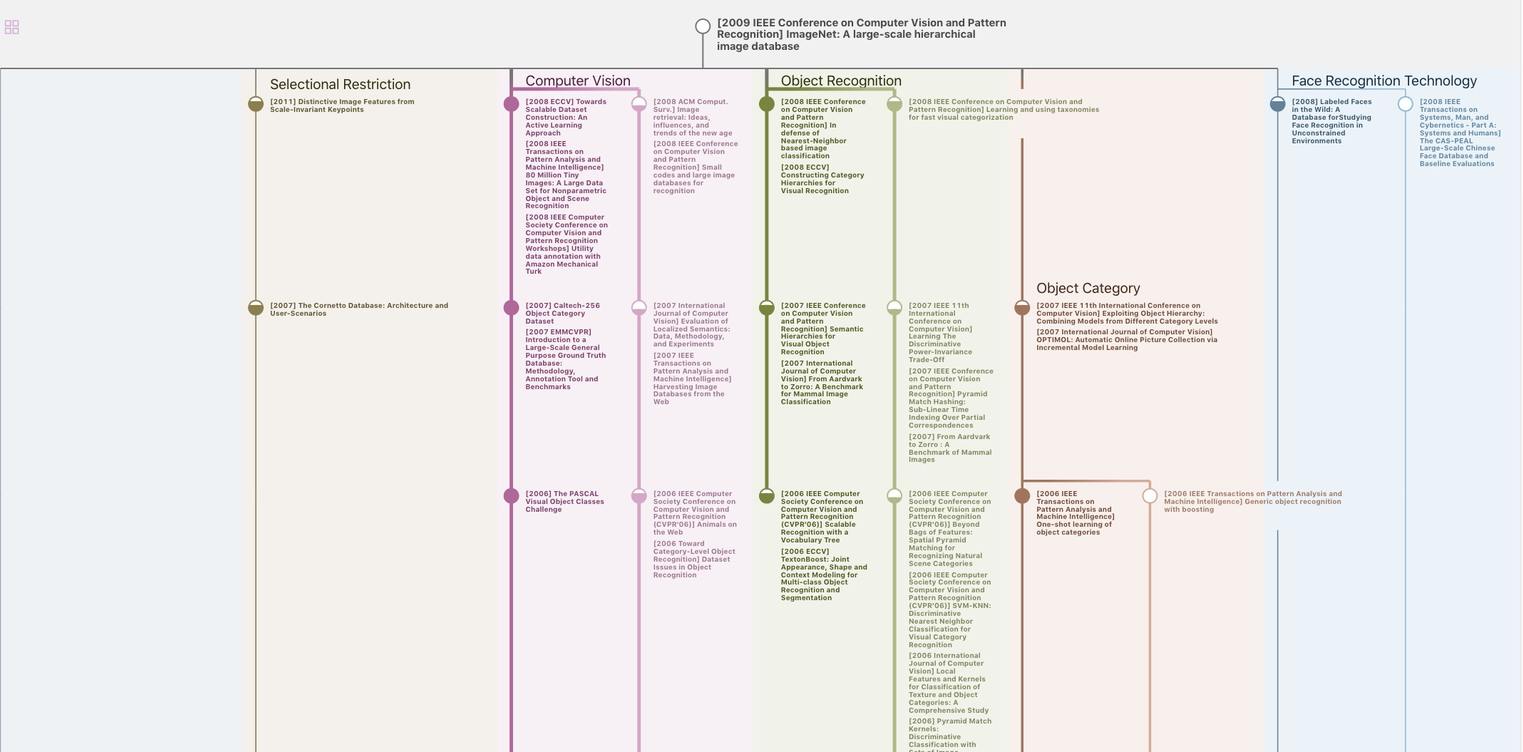
Generate MRT to find the research sequence of this paper
Chat Paper
Summary is being generated by the instructions you defined