Heterogeneous Multi-Robot Task Allocation for Garment Transformable Production using Deep Reinforcement Learning
CASE(2023)
摘要
This paper addresses the need for a more practical, flexible and scalable approach to garment manufacturing amidst the fashion industry's current shift towards transformable production. The current trend for personalized, on-demand orders has resulted in a need for greater involvement of static units capable of carrying out certain tasks (e.g. assembly, cutting, ironing) and mobile robots during the garment production process. Previous research has dealt with the development of a scheduler for these types of robots once all orders have been received. Although meaningful, this model remains impractical in realistic production settings. This paper introduces TransPRES (Transformable Production REsource Scheduler), a deep reinforcement learning-based scheduler that assigns three different kinds of static and mobile robots to individual tasks based on demand. This newly proposed method uses a tailor-made state to represent the transformable production, and an action that schedules the corresponding resource based on its type. To evaluate this approach, we developed a transformable production simulator that generates garment manufacturing orders with varying numbers of tasks, processing times, hierarchy, and resources required to complete them. Furthermore, we extended a heuristic-based approach and an additional reinforcement learning-based approach for comparison within a transformable production environment. Results show that our method outperforms previous approaches by being able to schedule 11% more tasks using 2 ms per flop which, in turn, demonstrates its effectiveness for the future of transformable production.
更多查看译文
关键词
deep reinforcement learning-based scheduler,fashion industry,flexible approach,garment manufacturing orders,garment transformable production,heterogeneous multirobot task allocation,heuristic-based approach,mobile robots,on-demand orders,realistic production settings,reinforcement learning-based approach,scalable approach,static robots,time 2.0 ms,transformable production environment,transformable production resource scheduler,transformable production simulator
AI 理解论文
溯源树
样例
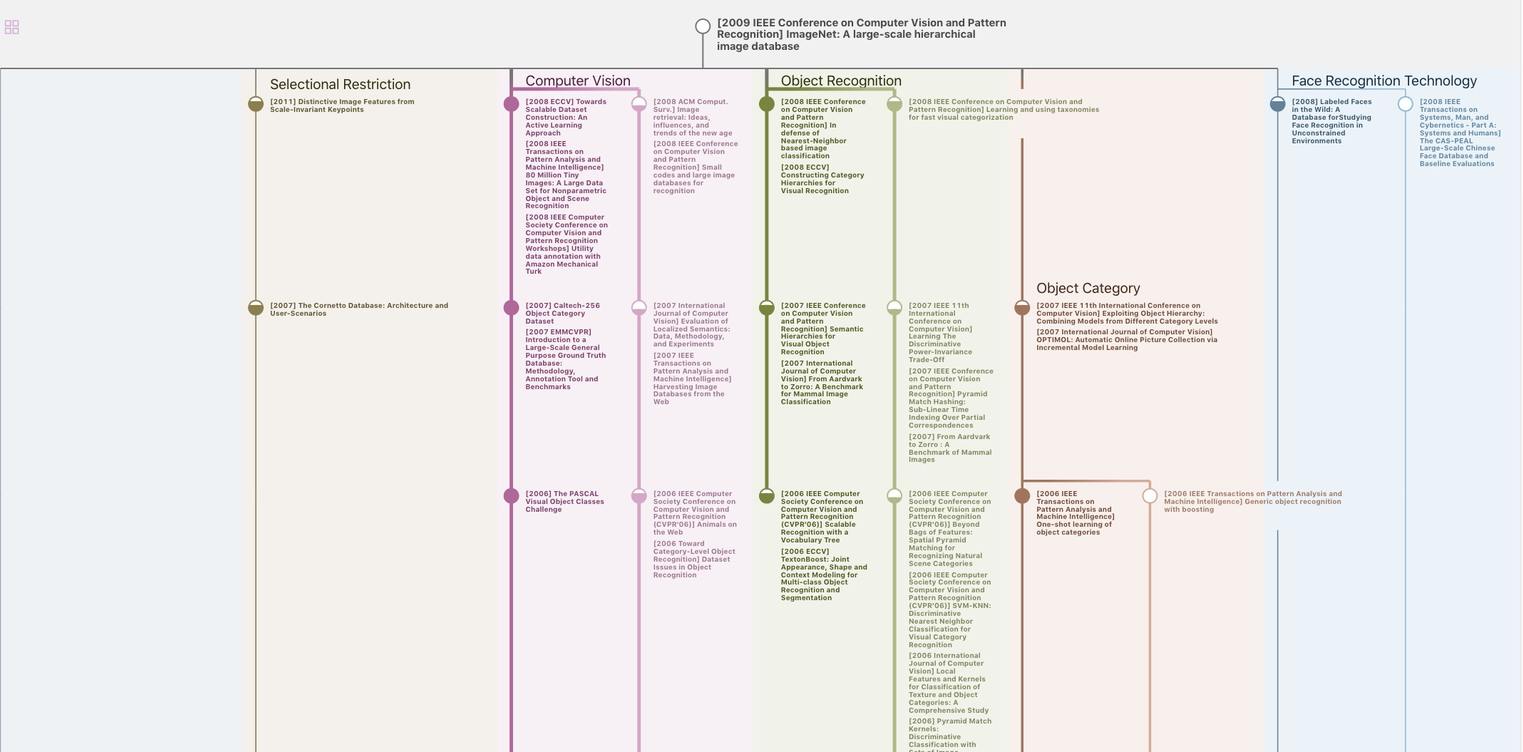
生成溯源树,研究论文发展脉络
Chat Paper
正在生成论文摘要