Vector Quantized Multi-modal Guidance for Alzheimer's Disease Diagnosis Based on Feature Imputation
MACHINE LEARNING IN MEDICAL IMAGING, MLMI 2023, PT I(2024)
摘要
Magnetic Resonance Imaging (MRI) and positron emission tomography (PET) are the most used imaging modalities for Alzheimer's disease (AD) diagnosis in clinics. Although PET can better capture AD-specific pathologies than MRI, it is less used compared with MRI due to high cost and radiation exposure. Imputing PET images from MRI is one way to bypass the issue of unavailable PET, but is challenging due to severe ill-posedness. Instead, we propose to directly impute classification-oriented PET features and combine them with real MRI to improve the overall performance of AD diagnosis. In order to more effectively impute PET features, we discretize the feature space by vector quantization and employ transformer to perform feature transition between MRI and PET. Our model is composed of three stages including codebook generation, mapping construction, and classifier enhancement based on combined features. We employ paired MRI-PET data during training to enhance the performance of MRI data during inference. Experimental results on ADNI dataset including 1346 subjects show a boost in classification performance of MRI without requiring PET. Our proposed method also outperforms other state-of-the-art data imputation methods.
更多查看译文
关键词
Discrete Learning,Multimodal Learning,Incomplete Modalities,Alzheimer's Disease,Classification,Feature Imputation
AI 理解论文
溯源树
样例
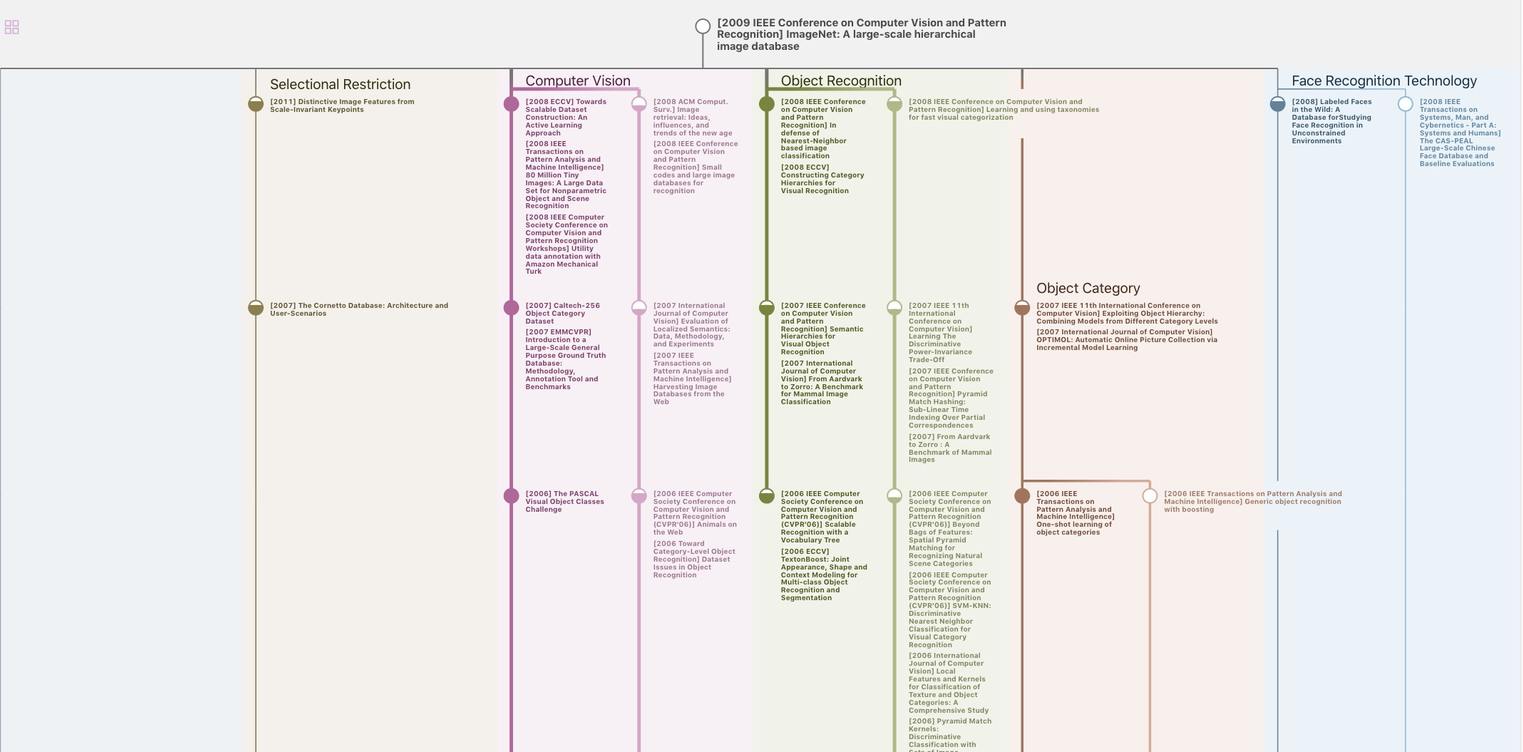
生成溯源树,研究论文发展脉络
Chat Paper
正在生成论文摘要