Reconstruction of 3D Fetal Brain MRI from 2D Cross-Sectional Acquisitions Using Unsupervised Learning Network
MLMI@MICCAI (1)(2024)
Abstract
Fetal brain magnetic resonance imaging (MRI) is becoming more important for early brain assessment in prenatal examination. Fast acquisition of three cross-sectional series/views is often used to eliminate motion effects using single-shot fast spin-echo sequences. Although stacked in 3D volumes, these slices are essentially 2D images with large slice thickness and distances (4 to 6mm) resulting blurry multiplanar views. To better visualize and quantify fetal brains, it is desirable to reconstruct 3D images from different 2D cross-sectional series. In this paper, we present a super-resolution CNN-based network for 3D image reconstruction using unsupervised learning, referred to as cross-sectional image reconstruction (C-SIR). The key idea is that different cross-sectional images can help each other for training the C-SIR model. Additionally, existing high resolution data can also be used for pre-training the network in a supervised manner. In experiments, we show that such a network can be trained to reconstruct 3D images using simulated down-sampled adult images with much better image quality and image segmentation accuracy. Then, we illustrate that the proposed C-SIR approach generates relatively clear 3D fetal images than other algorithms.
MoreTranslated text
Key words
Unsupervised learning,3D reconstruction from cross-sectional images
AI Read Science
Must-Reading Tree
Example
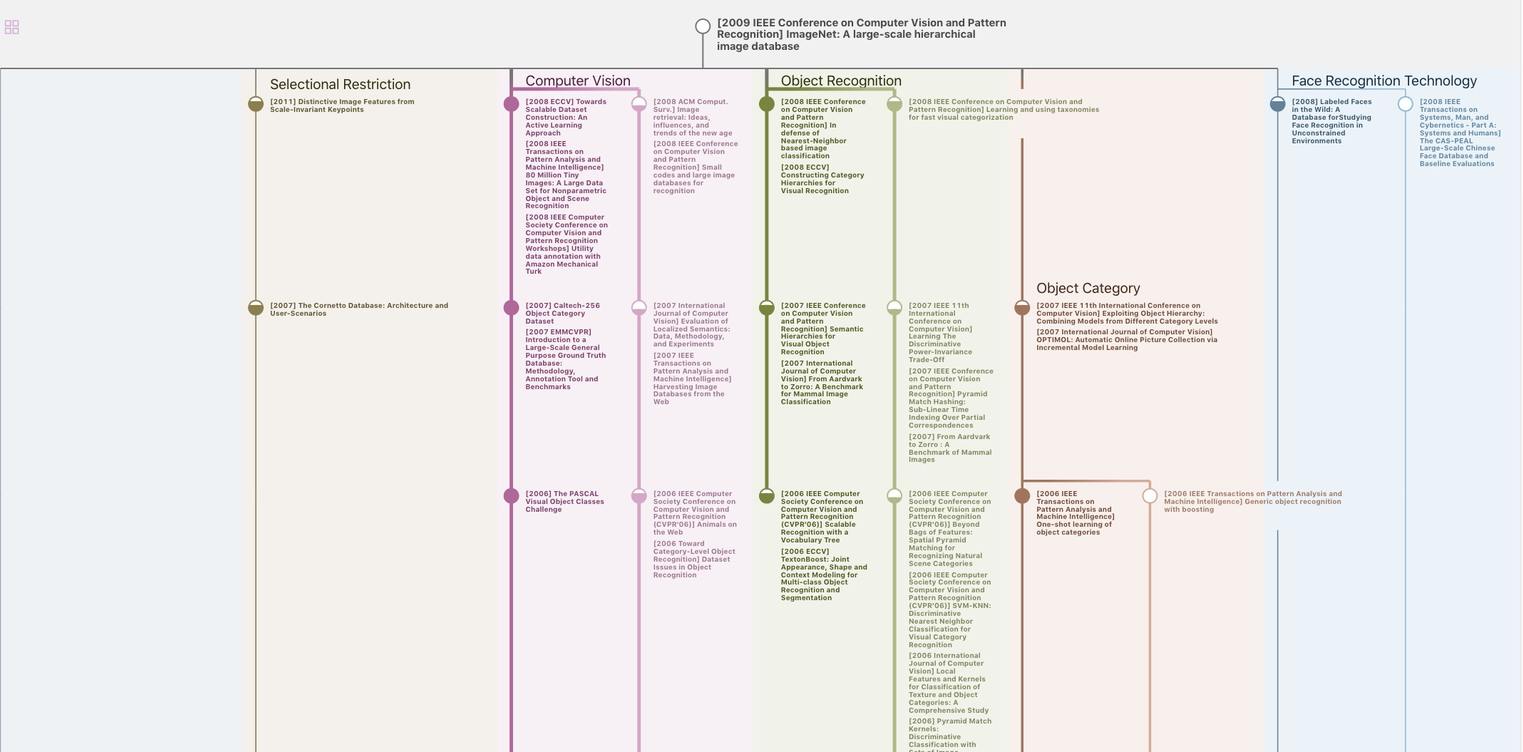
Generate MRT to find the research sequence of this paper
Chat Paper
Summary is being generated by the instructions you defined