Application of Machine Learning Techniques to Predict Teenage Obesity Using Earlier Childhood Measurements from Millennium Cohort Study.
ICCBDC(2023)
摘要
Obesity is a major global concern with more than 2.1 billion people overweight or obese worldwide, which amounts to almost 30% of the global population. If the current trend continues, the overweight and obese population is likely to increase to 41% by 2030. Individuals developing signs of weight gain or obesity are also at the risk of developing serious illnesses such as type 2 diabetes, respiratory problems, heart disease, stroke, and even death. It is essential to detect childhood obesity as early as possible since children who are either overweight or obese in their younger age tend to stay obese in their adult lives. This research utilises the vast amount of data available via UK's millennium cohort study to construct machine learning driven framework to predict young people at the risk of becoming overweight or obese. The focus of this paper is to develop a framework to predict childhood obesity using earlier childhood data and other relevant features. The use of novel data balancing technique and inclusion of additional relevant features resulted in sensitivity, specificity, and F1-score of 77.32%, 76.81%, and 77.02% respectively. The proposed technique utilises easily obtainable features making it suitable to be used in a clinical and non-clinical environment.
更多查看译文
AI 理解论文
溯源树
样例
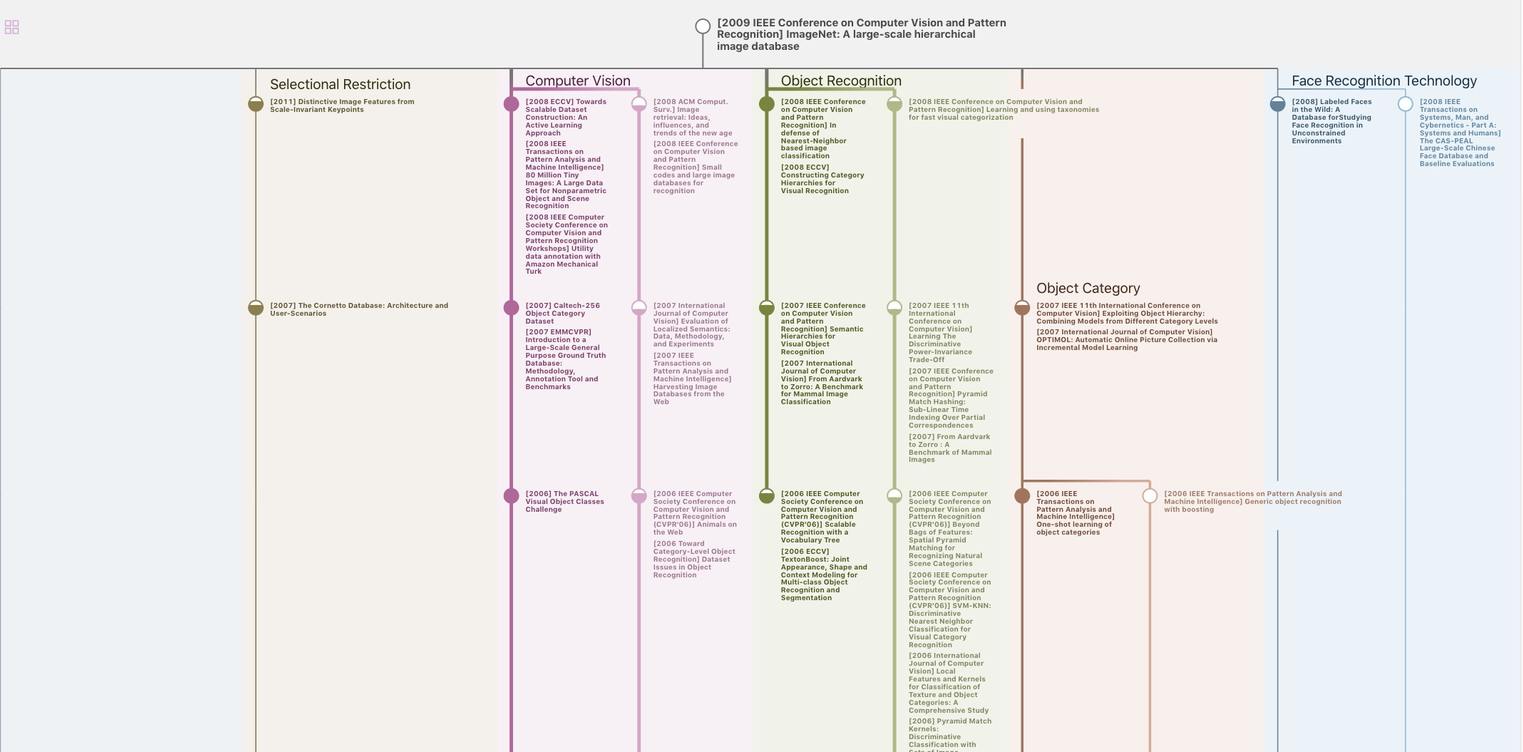
生成溯源树,研究论文发展脉络
Chat Paper
正在生成论文摘要