Investigating the Performance of Data Complexity & Instance Hardness Measures as A Meta-Feature in Overlapping Classes Problem.
ICCBDC(2023)
摘要
Since the meta-learning recommendation's quality depends on the meta-features decision quality, a common problem in meta-learning is establishing a (good) collection of meta-features that best represent the dataset properties. Therefore, many meta-feature measures/methods have been proposed during the last decade to describe the characteristics of the data. However, little attention has been paid to validating the meta-feature decisions in reflecting the actual data properties. In particular, if the meta-feature analysis is negatively affected by complex data characteristics, such as class overlap due to the distortion imposed by the noisy features at the decision boundary of the classes and thereby produces biased meta-learning recommendations that do not match the actual data characteristics (either by overestimating or underestimating the complexity). Hence, this issue is crucial to ensure the success of the meta-learning model since the learning algorithm selection decision is based on meta-feature analysis. Based on that, in this work, we aim to investigate this by assessing the performance of Complexity Measures (global/data-level measures) & Instance Hardness Measures (local/instance-level measures) as a meta-feature in reflecting the actual data complexity associated with the high-class overlapping problem. The reason for focusing on the overlapping classes problem is that several studies have proven that this data issue significantly contributes to degrading prediction accuracy, with which most real-world datasets are associated. On the other hand, the motivation for using the above measures among different meta-feature methods proposed in the literature is that since this study aims to focus on the overlapping classes problem, the above measures are mainly proposed to estimate the data complexity according to the geometrical descriptions focusing on the class overlap imposed by feature values, in which match the data problem that the study interested to investigate.
更多查看译文
AI 理解论文
溯源树
样例
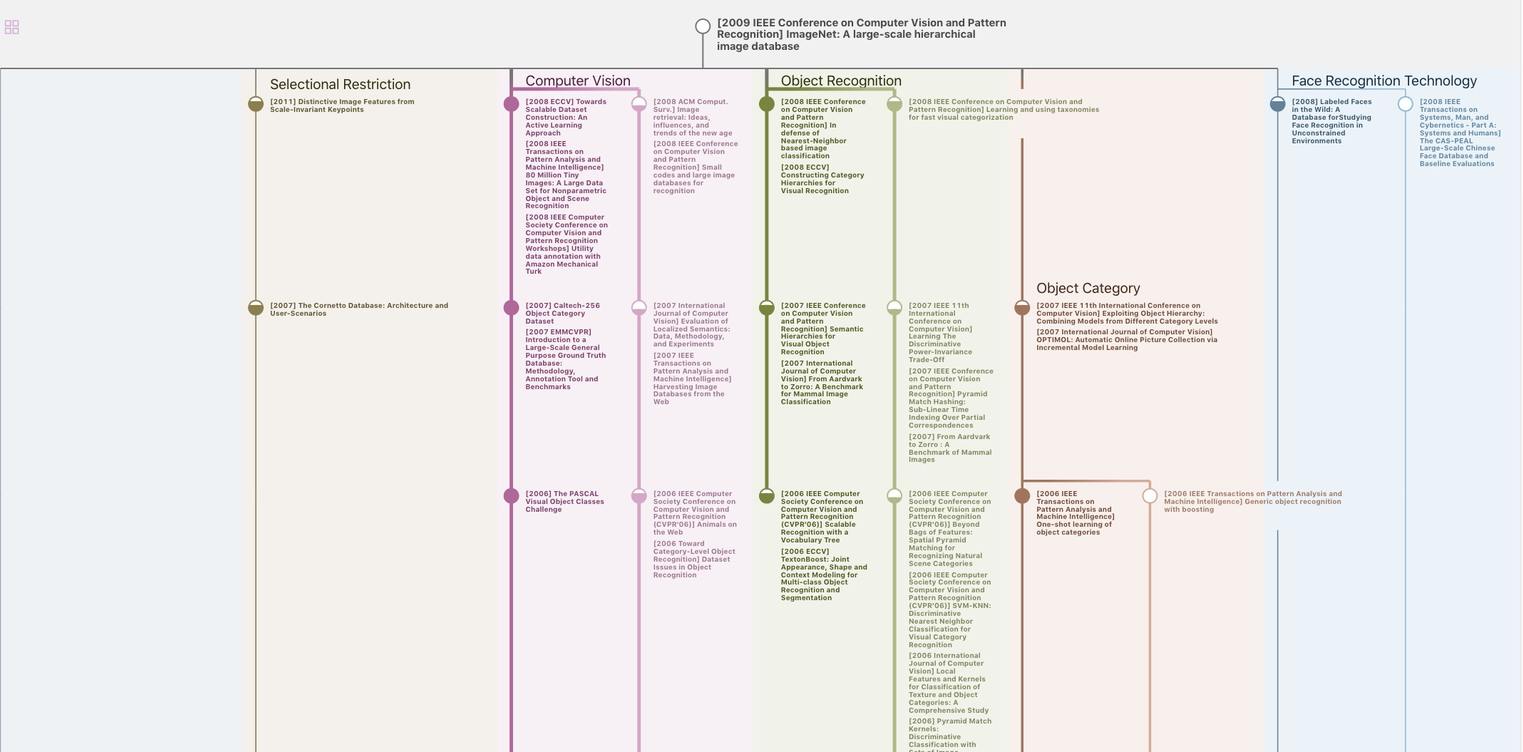
生成溯源树,研究论文发展脉络
Chat Paper
正在生成论文摘要