DeepEAD: Explainable Anomaly Detection from System Logs
ICC 2023 - IEEE INTERNATIONAL CONFERENCE ON COMMUNICATIONS(2023)
摘要
System logs record rich information for system events. Practical anomaly detection from system logs should be able to address three challenges: 1) understanding complicated attributes in event logs; 2) extracting complex context relations among events; and 3) providing concrete explanations to human analysts. In this paper, we develop an attention-equipped encoder-decoder system to capture context from system logs for explainable anomaly detection. For each target event, we collect its nearby events in chronological order as its context events. Instead of using a recurrent neural network-based encoder like previous works, we adopt a Transformer-based encoder to extract complex relations among context events and their attributes. Then, a context vector is generated and passed to the decoder, where an attention matrix is learned and used to weigh the context events for detecting the anomalies. Evaluation on the large-scale real-world Los Alamos National Laboratory dataset shows that, compared with existing works, our methods can provide fine-grained one-to-one attention to help explain the importance of each attribute in the context events to the prediction, without sacrificing detection performance.
更多查看译文
关键词
Anomaly detection,Transformer,explainable deep learning,context analysis
AI 理解论文
溯源树
样例
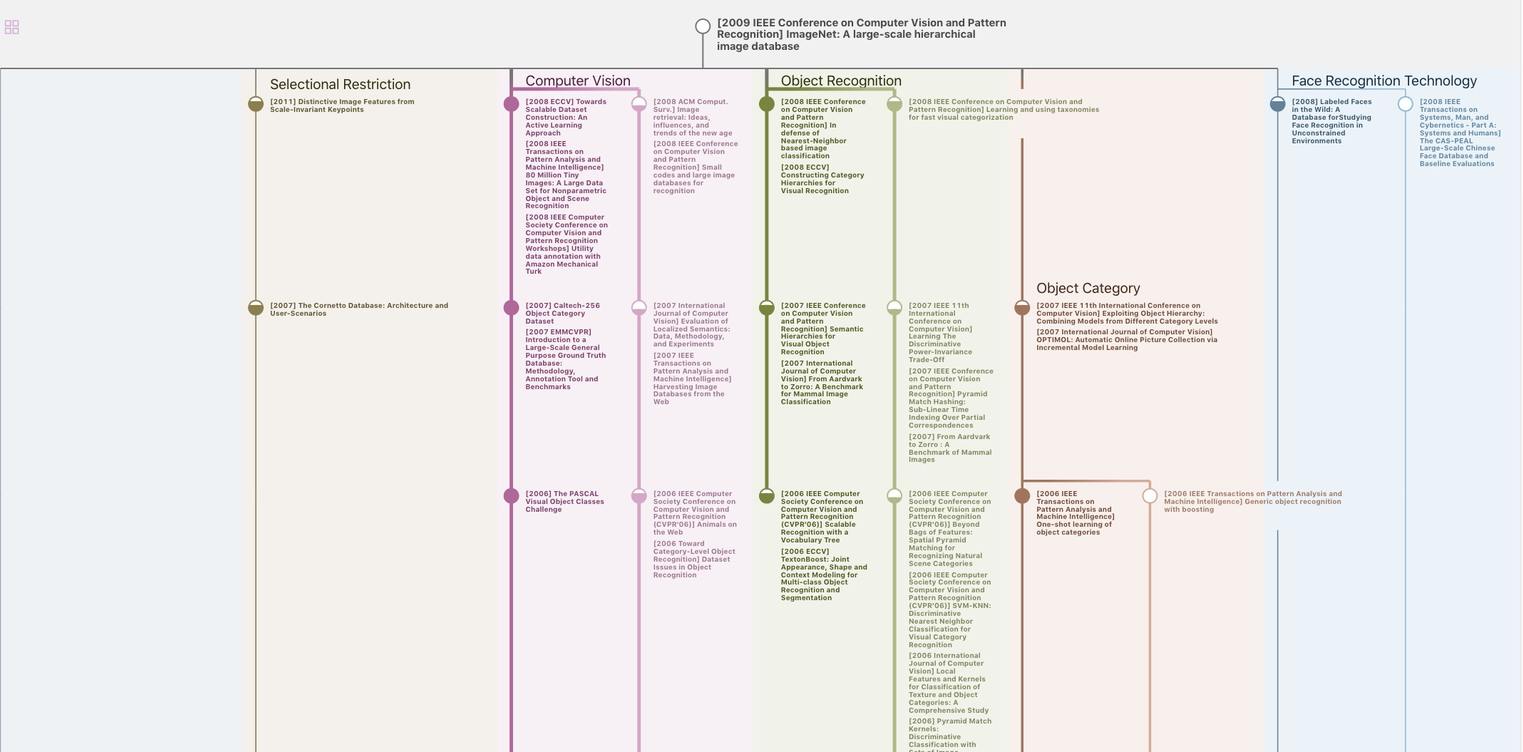
生成溯源树,研究论文发展脉络
Chat Paper
正在生成论文摘要